Guiding Corpus-based Set Expansion by Auxiliary Sets Generation and Co-Expansion
WWW '20: The Web Conference 2020 Taipei Taiwan April, 2020(2020)
摘要
Given a small set of seed entities (e.g., “USA”, “Russia”), corpus-based set expansion is to induce an extensive set of entities which share the same semantic class (Country in this example) from a given corpus. Set expansion benefits a wide range of downstream applications in knowledge discovery, such as web search, taxonomy construction, and query suggestion. Existing corpus-based set expansion algorithms typically bootstrap the given seeds by incorporating lexical patterns and distributional similarity. However, due to no negative sets provided explicitly, these methods suffer from semantic drift caused by expanding the seed set freely without guidance. We propose a new framework, Set-CoExpan, that automatically generates auxiliary sets as negative sets that are closely related to the target set of user’s interest, and then performs multiple sets co-expansion that extracts discriminative features by comparing target set with auxiliary sets, to form multiple cohesive sets that are distinctive from one another, thus resolving the semantic drift issue. In this paper we demonstrate that by generating auxiliary sets, we can guide the expansion process of target set to avoid touching those ambiguous areas around the border with auxiliary sets, and we show that Set-CoExpan outperforms strong baseline methods significantly.
更多查看译文
关键词
Set Expansion, Bootstrap Methods, Semantic Computing, Web Mining
AI 理解论文
溯源树
样例
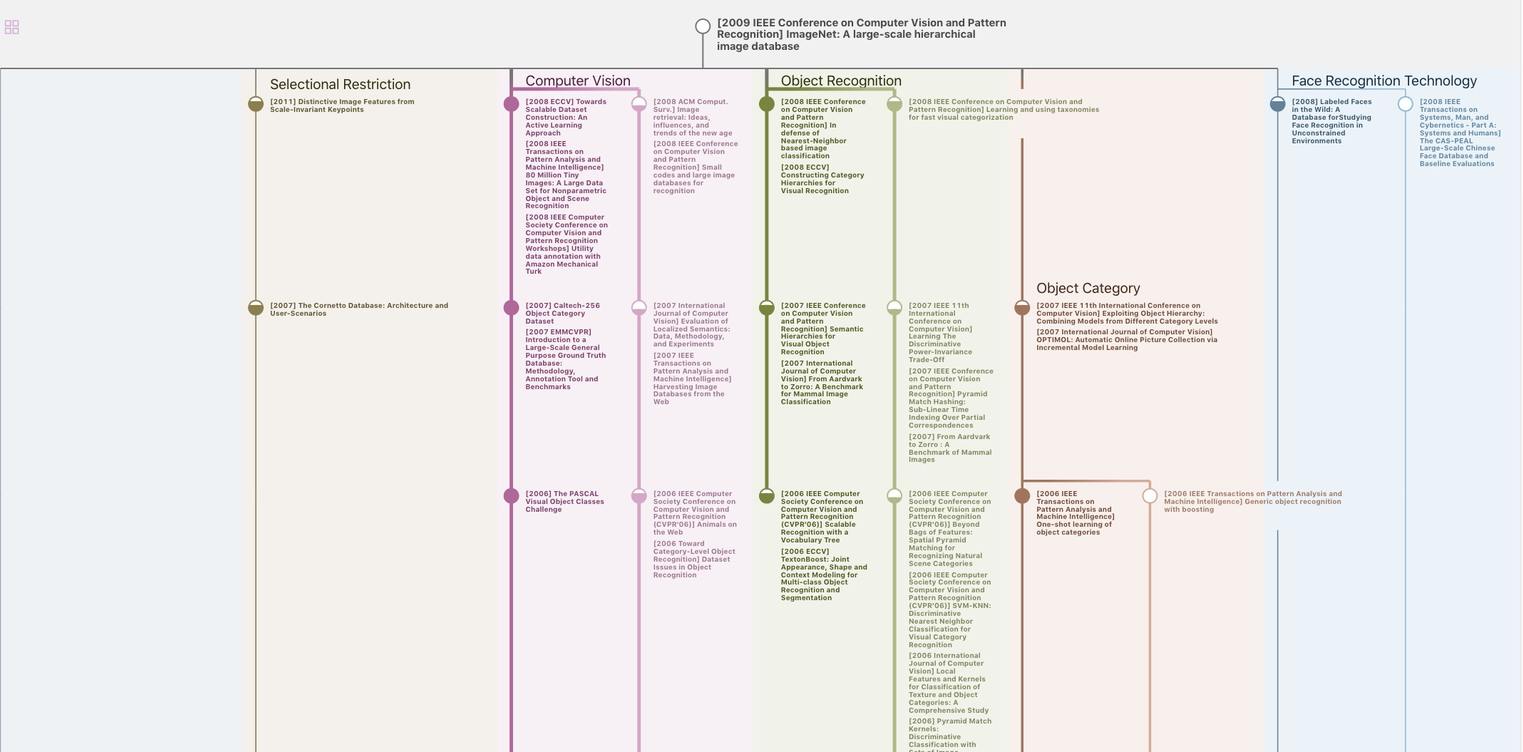
生成溯源树,研究论文发展脉络
Chat Paper
正在生成论文摘要