The Relative Importance of Clinical and Socio-Demographic Variables in Prognostic Prediction in Non-Small Cell Lung Cancer: A Variable Importance Approach.
MEDICAL CARE(2020)
摘要
Background: Prognostic modeling in health care has been predominantly statistical, despite a rapid growth of literature on machine-learning approaches in biological data analysis. We aim to assess the relative importance of variables in predicting overall survival among patients with non-small cell lung cancer using a Variable Importance (VIMP) approach in a machine-learning Random Survival Forest (RSF) model for posttreatment planning and follow-up. Methods: A total of 935 non-small cell lung cancer patients were randomly and equally divided into 2 training and testing cohorts in an RFS model. The prognostic variables included age, sex, race, the TNM Classification of Malignant Tumors (TNM) stage, smoking history, Eastern Cooperative Oncology Group performance status, histologic type, treatment category, maximum standard uptake value of whole-body tumor (SUVmaxWB), whole-body metabolic tumor volume (MTVwb), and Charlson Comorbidity Index. The VIMP was calculated using a permutation method in the RSF model. We further compared the VIMP of the RSF model to that of the standard Cox survival model. We examined the order of VIMP with the differential functional forms of the variables. Results: In both the RSF and the standard Cox models, the most important variables are treatment category, TNM stage, and MTVwb. The order of VIMP is more robust in RSF model than in Cox model regarding the differential functional forms of the variables. Conclusions: The RSF VIMP approach can be applied alongside with the Cox model to further advance the understanding of the roles of prognostic factors, and improve prognostic precision and care efficiency.
更多查看译文
关键词
prognostic prediction,variable importance,machine learning,lung cancer,random survival forest model
AI 理解论文
溯源树
样例
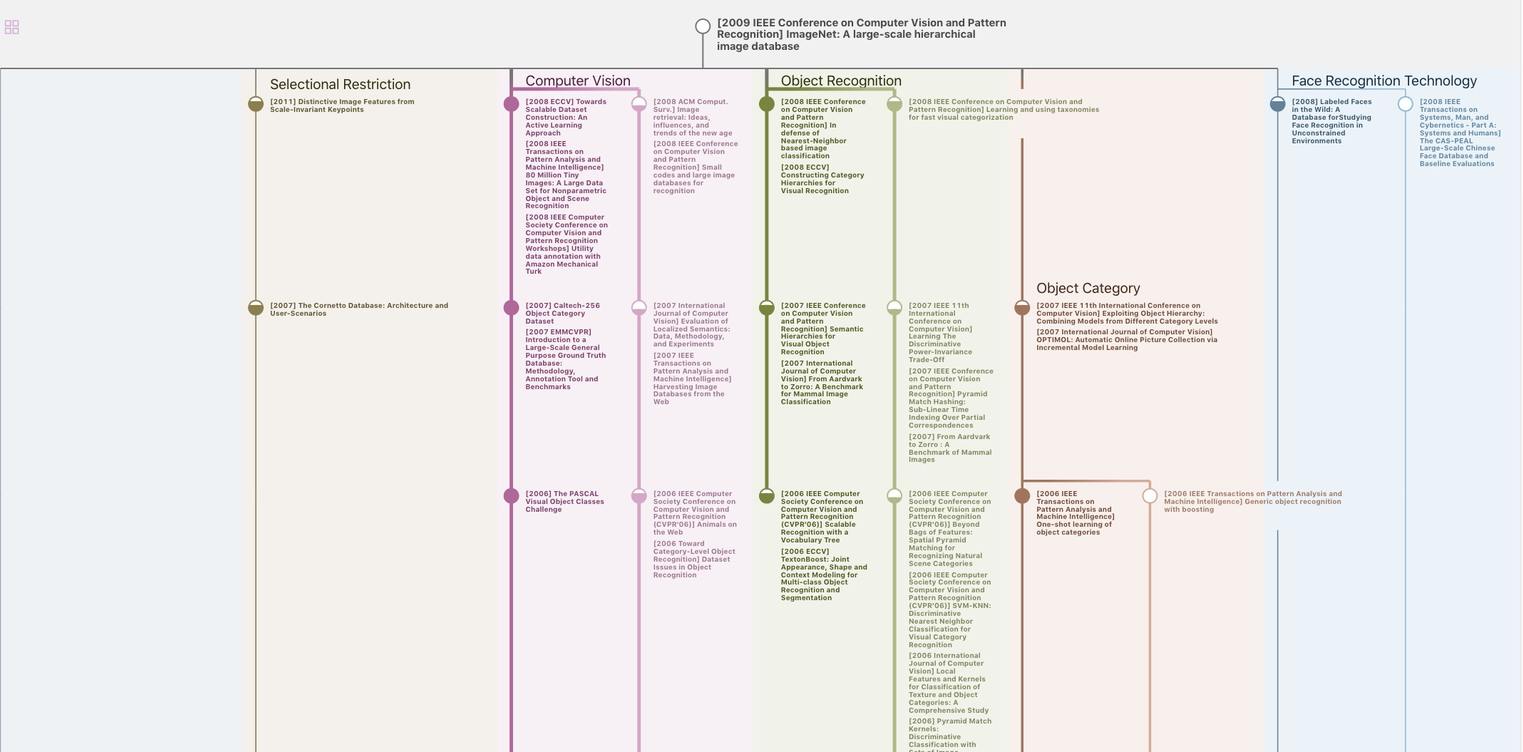
生成溯源树,研究论文发展脉络
Chat Paper
正在生成论文摘要