Breast microcalcifications detection based on fusing features with DTCWT.
JOURNAL OF X-RAY SCIENCE AND TECHNOLOGY(2020)
摘要
BACKGROUND: Breast cancer is a common disease in women. Early detection and early treatment can reduce breast cancer mortality. Studies have shown that breast cancer microcalcifications is one of the important clinical manifestations of early breast cancer, and sometimes even the only manifestation. When the mammography image shows typical malignant microcalcification, it can be diagnosed as breast cancer without any other signs of malignancy. In the aided diagnosis of microcalcifications, it is a crucial step to automatically find and locate regions of interest containing microcalcifications. However, the existing feature extraction method for microcalcifications only extracts features in the time domain or wavelet domain, and does not completely represent all the information of the region of interest. An extraction method based on the combination of Dual-Tree Complex Wavelet Transform (DTCWT) and texture features is proposed in the paper. METHODS: First, the processing operations including denoising, enhancement, and edge detection were performed on mammograms. Sub-image segmentation is then performed. DTCWT features and texture features are extracted for each sub-image.DTCWT features are combined with texture features, and then genetic algorithm is used for feature optimization. The features are classified by the Extreme Learning Machine (ELM) to achieve rapid detection and automatic extraction of ROI with microcalcifications. The experimental results verify that the feature model proposed in this paper has the highest detection rate for ROI regions. The ROI region extracted by the proposed feature model was used as subsequent experimental data. Three different methods were used to detect the microcalcifications, including Top-hat, wavelet transform, and methods combining Top-Hat and wavelet transform. RESULTS: The method was applied to 100 mammograms from the mammograms database of women in Northeast China. In the automatic extraction of ROI, the accuracy, sensitivity, specificity, positive accuracy and negative accuracy of the proposed model combined with DTCWT were 95.92%, 96.71%, 92.20%, 93.65%, 96.33%, respectively. When the Top-hat algorithm was used for microcalcifications detection, the sensitivity reached 89.6%, and the false positive detection rate was 2.6. When the wavelet transform algorithm was used for microcalcifications detection, the sensitivity was 91.1%, and the false positive detection rate was 3.28. When the combined algorithm was used for microcalcifications detection, the sensitivity was 86.7%, and the false positive detection rate decreased to 1.35. CONCLUSIONS: The proposed model combined with DTCWT features achieves better result in the automatic extraction of ROI. Moreover, in the subsequent detection of microcalcifications based on three methods, the three methods achieved better results in sensitivity and false positive detection rate, respectively.
更多查看译文
关键词
Breast Cancer,Microcalcifications,DTCWT,ELM,Mammogram
AI 理解论文
溯源树
样例
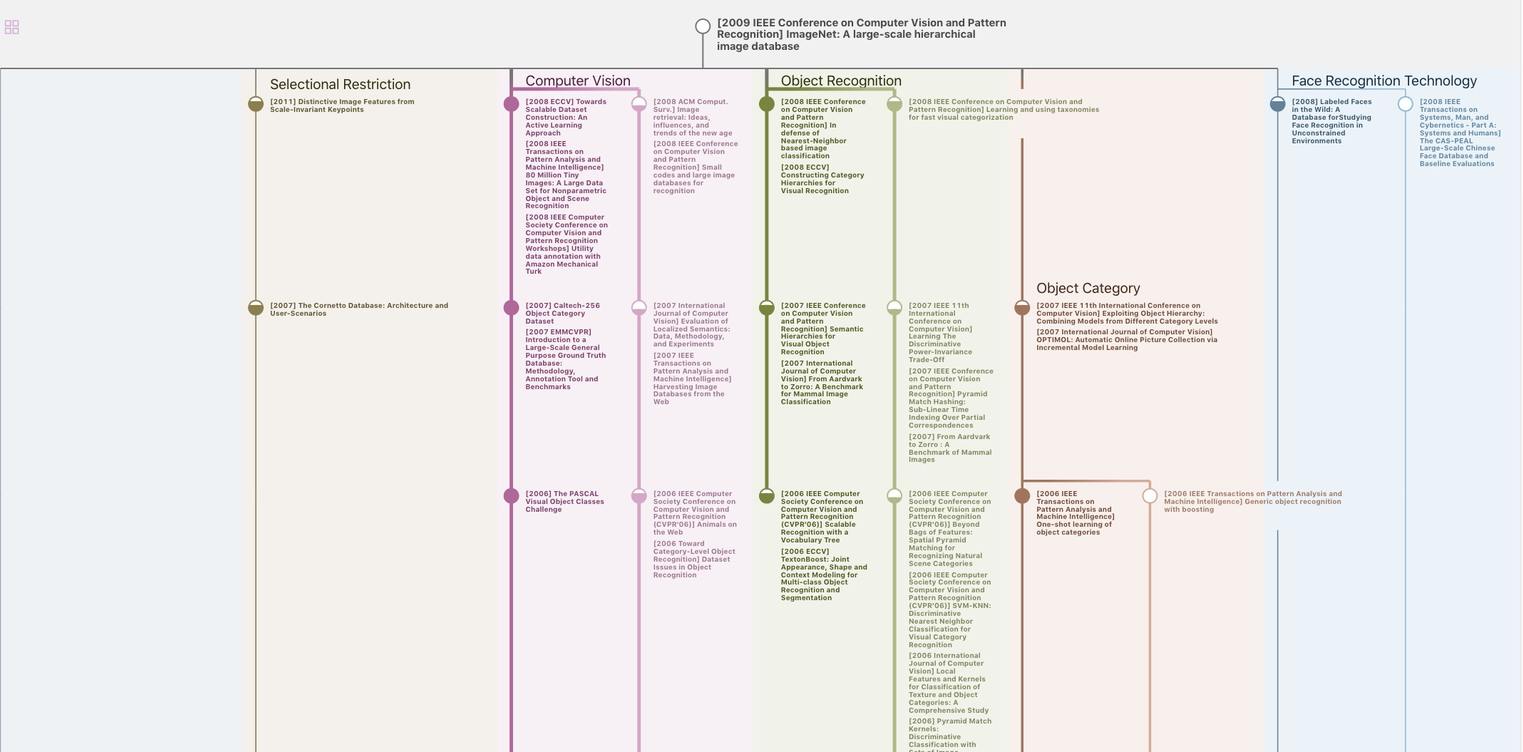
生成溯源树,研究论文发展脉络
Chat Paper
正在生成论文摘要