Identification Of Non-Linear Rf Systems Using Backpropagation
2020 IEEE INTERNATIONAL CONFERENCE ON COMMUNICATIONS WORKSHOPS (ICC WORKSHOPS)(2020)
摘要
In this work, we use deep unfolding to view cascaded non-linear RF systems as model-based neural networks. This view enables the direct use of a wide range of neural network tools and optimizers to efficiently identify such cascaded models. We demonstrate the effectiveness of this approach through the example of digital self-interference cancellation in full-duplex communications where an IQ imbalance model and a non-linear PA model are cascaded in series. For a self-interference cancellation performance of approximately 44.5 dB, the number of model parameters can be reduced by 74% and the number of operations per sample can be reduced by 79% compared to an expanded linear-in-parameters polynomial model.
更多查看译文
关键词
Backpropagation, memory polynomial, parallel Hammerstein model, Volterra series, SI cancellation
AI 理解论文
溯源树
样例
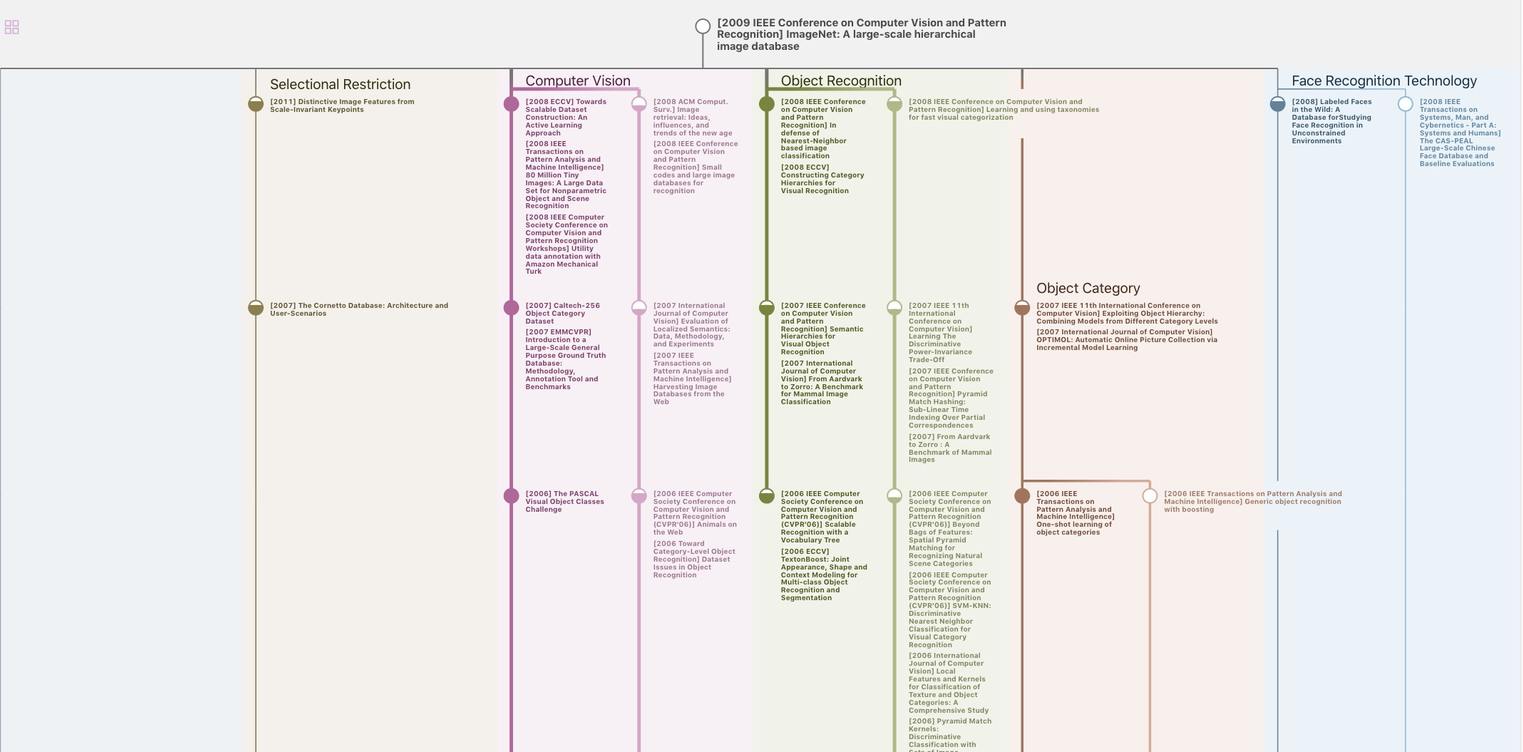
生成溯源树,研究论文发展脉络
Chat Paper
正在生成论文摘要