Basic Ensembles of Vanilla-Style Deep Learning Models Improve Liver Segmentation From CT Images
arxiv(2020)
摘要
Segmentation of the liver from 3D computer tomography (CT) images is one of the most frequently performed operations in medical image analysis. In the past decade, Deep Learning Models (DMs) have offered significant improvements over previous methods for liver segmentation. The success of DMs is usually owed to the user's expertise in deep learning as well as to intricate training procedures. The need for bespoke expertise limits the reproducibility of empirical studies involving DMs. Today's consensus is that an ensemble of DMs works better than the individual component DMs. In this study we set off to explore the potential of ensembles of publicly available, `vanilla-style' DM segmenters Our ensembles were created from four off-the-shelf DMs: U-Net, Deepmedic, V-Net, and Dense V-Networks. To prevent further overfitting and to keep the overall model simple, we use basic non-trainable ensemble combiners: majority vote, average, product and min/max. Our results with two publicly available data sets (CHAOS and 3Dircadb1) demonstrate that ensembles are significantly better than the individual segmenters on four widely used metrics.
更多查看译文
关键词
liver segmentation,deep learning,basic ensembles,vanilla-style
AI 理解论文
溯源树
样例
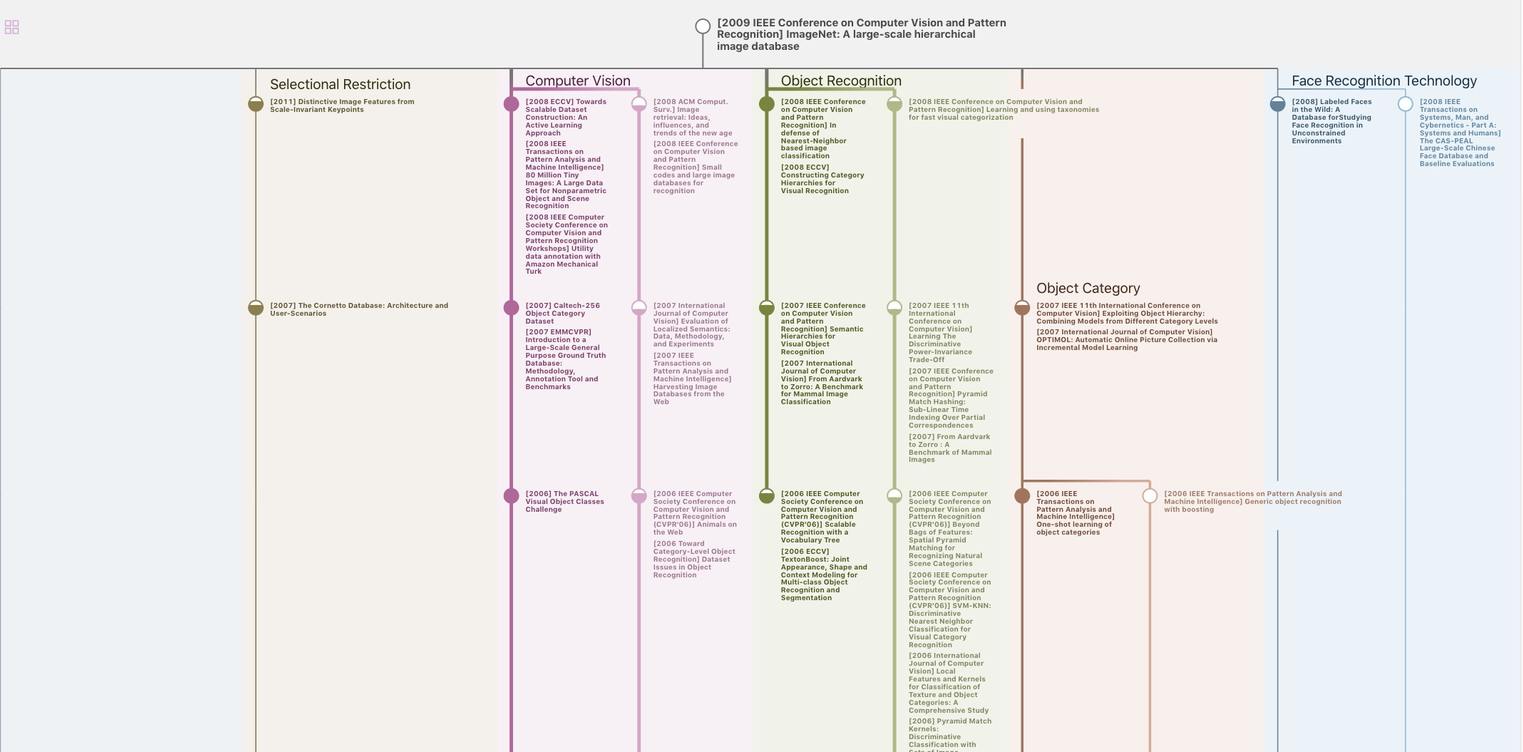
生成溯源树,研究论文发展脉络
Chat Paper
正在生成论文摘要