CounterExample Guided Neural Synthesis
arxiv(2020)
摘要
Program synthesis is the generation of a program from a specification. Correct synthesis is difficult, and methods that provide formal guarantees suffer from scalability issues. On the other hand, neural networks are able to generate programs from examples quickly but are unable to guarantee that the program they output actually meets the logical specification. In this work we combine neural networks with formal reasoning: using the latter to convert a logical specification into a sequence of examples that guides the neural network towards a correct solution, and to guarantee that any solution returned satisfies the formal specification. We apply our technique to synthesising loop invariants and compare the performance to existing solvers that use SMT and existing techniques that use neural networks. Our results show that the formal reasoning based guidance improves the performance of the neural network substantially, nearly doubling the number of benchmarks it can solve.
更多查看译文
关键词
synthesis
AI 理解论文
溯源树
样例
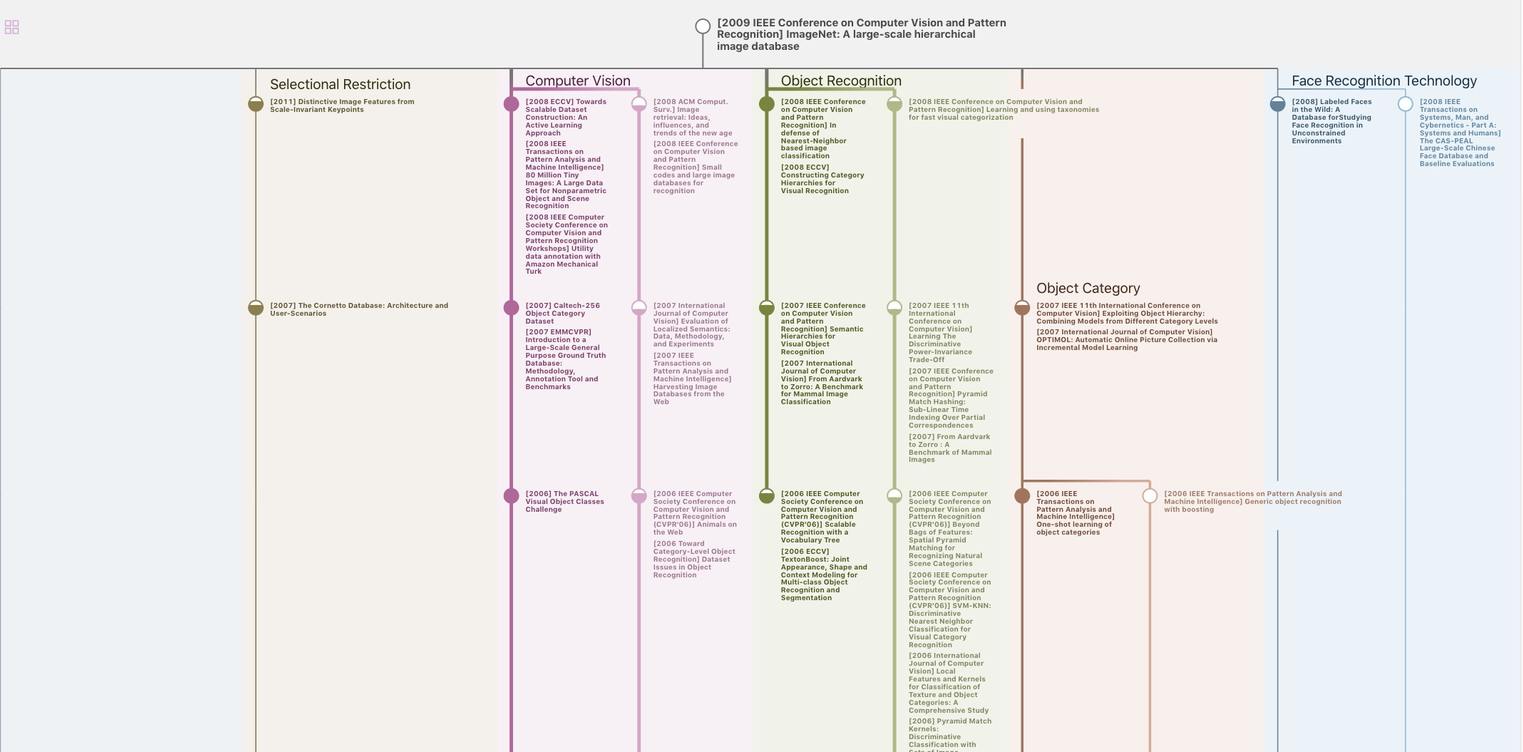
生成溯源树,研究论文发展脉络
Chat Paper
正在生成论文摘要