Recurrent Memory Reasoning Network for Expert Finding in Community Question Answering.
WSDM '20: The Thirteenth ACM International Conference on Web Search and Data Mining Houston TX USA February, 2020(2020)
摘要
Expert finding is a task designed to enable recommendation of the right person who can provide high-quality answers to a requester's question. Most previous works try to involve a content-based recommendation, which only superficially comprehends the relevance between a requester's question and the expertise of candidate experts by exploring the content or topic similarity between the requester's question and the candidate experts' historical answers. However, if a candidate expert has never answered a question similar to the requester's question, then existing methods have difficulty making a correct recommendation. Therefore, exploring the implicit relevance between a requester's question and a candidate expert's historical records by perception and reasoning should be taken into consideration. In this study, we propose a novel \textslrecurrent memory reasoning network (RMRN) to perform this task. This method focuses on different parts of a question, and accordingly retrieves information from the histories of the candidate expert.Since only a small percentage of historical records are relevant to any requester's question, we introduce a Gumbel-Softmax-based mechanism to select relevant historical records from candidate experts' answering histories. To evaluate the proposed method, we constructed two large-scale datasets drawn from Stack Overflow and Yahoo! Answer. Experimental results on the constructed datasets demonstrate that the proposed method could achieve better performance than existing state-of-the-art methods.
更多查看译文
关键词
Expert Finding, Community Question Answering, Memory Network
AI 理解论文
溯源树
样例
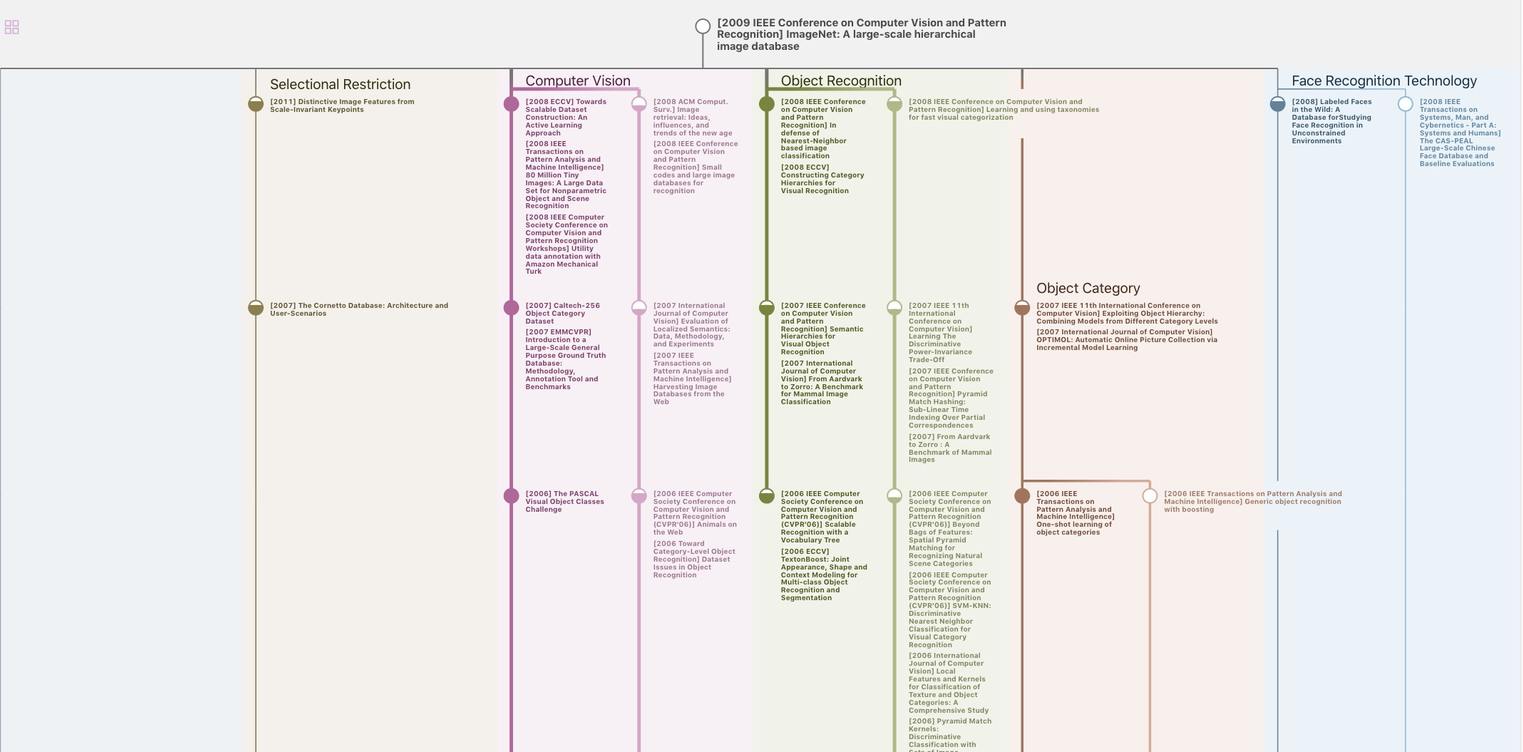
生成溯源树,研究论文发展脉络
Chat Paper
正在生成论文摘要