Context-aware Deep Model for Joint Mobility and Time Prediction.
WSDM '20: The Thirteenth ACM International Conference on Web Search and Data Mining Houston TX USA February, 2020(2020)
摘要
Mobility prediction, which is to predict where a user will arrive based on the user's historical mobility records, has attracted much attention. We argue that it is more useful to know not only where but also when a user will arrive next in many scenarios such as targeted advertising and taxi service. In this paper, we propose a novel context-aware deep model called DeepJMT for jointly performing mobility prediction (to know where) and time prediction (to know when). The DeepJMT model consists of (1) a hierarchical recurrent neural network (RNN) based sequential dependency encoder, which is more capable of capturing a user's mobility regularities and temporal patterns compared to vanilla RNN based models; (2) a spatial context extractor and a periodicity context extractor to extract location semantics and the user's periodicity, respectively; and (3) a co-attention based social & temporal context extractor which could extract the mobility and temporal evidence from social relationships. Experiments conducted on three real-world datasets show that DeepJMT outperforms the state-of-the-art mobility prediction and time prediction methods.
更多查看译文
关键词
mobility prediction, user modeling, location based services, neural networks
AI 理解论文
溯源树
样例
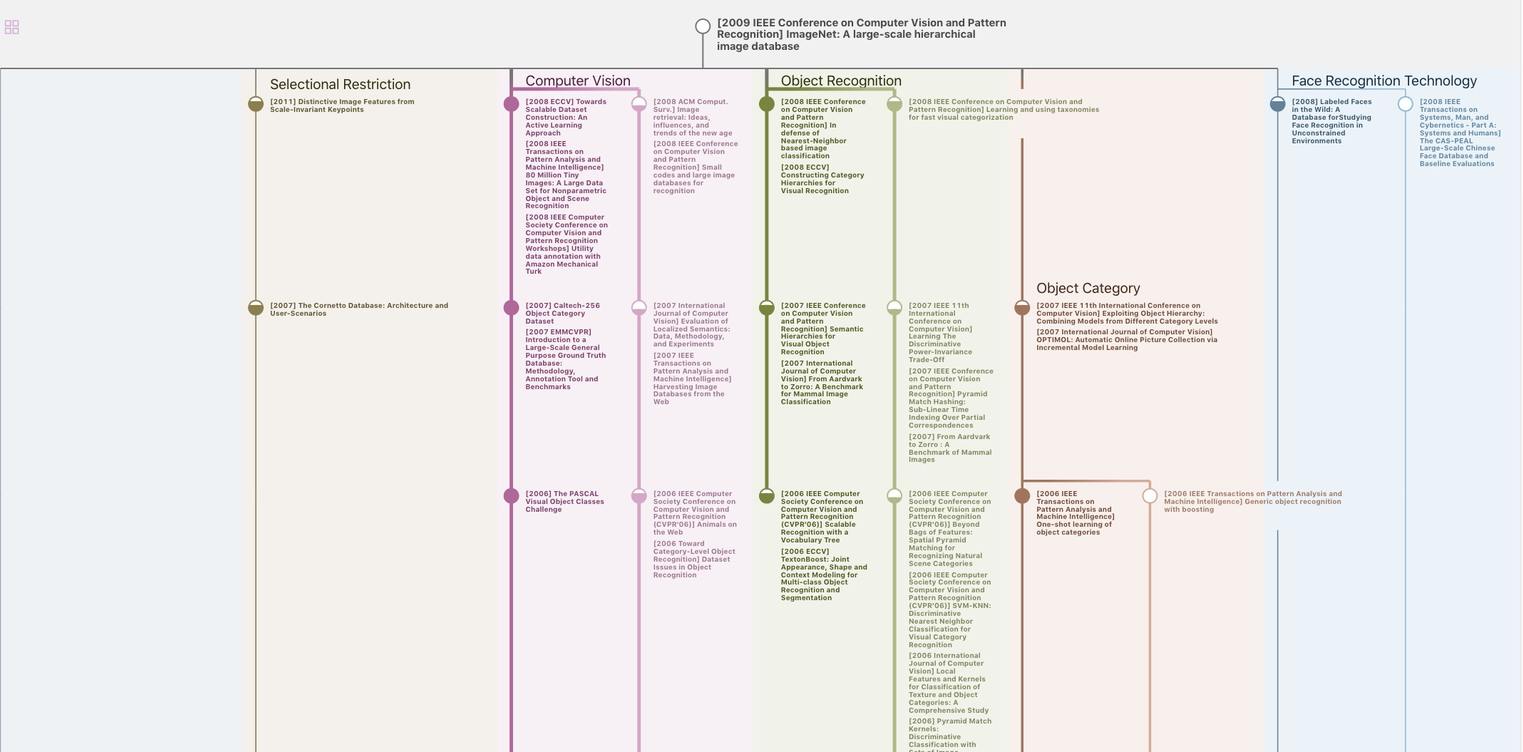
生成溯源树,研究论文发展脉络
Chat Paper
正在生成论文摘要