Decision Making Under Uncertainty With Convolutional Deep Gaussian Processes
PROCEEDINGS OF THE 7TH ACM IKDD CODS AND 25TH COMAD (CODS-COMAD 2020)(2020)
摘要
Deep learning models such as convolutional neural networks have brought advances in computer vision and were found to surpass human accuracy in computer vision problems. This has resulted in their use in many safety critical applications such as autonomous driving and healthcare, where decision making under uncertainty is crucial. However, deep learning models are vulnerable to out of sample and adversarial examples and they can be very risky to use in safety critical applications. Deep Gaussian process provide a Bayesian non-parametric approach to deep learning and are capable of modelling the uncertainty in data and model. In this paper, we show the uncertainty quantification capabilities of Convolutional deep Gaussian processes for computer vision problems. We show that convolutional deep Gaussian processes provide better uncertainty estimates for various settings such as out-of-distribution samples, adversarial attacks and uncertainty calibration experiments. They are found to be more robust than convolutional neural networks, Bayesian convolutional neural networks and deep Gaussian processes for image classification.
更多查看译文
关键词
Gaussian processes, deep learning, Bayesian learning, uncertainty quantification
AI 理解论文
溯源树
样例
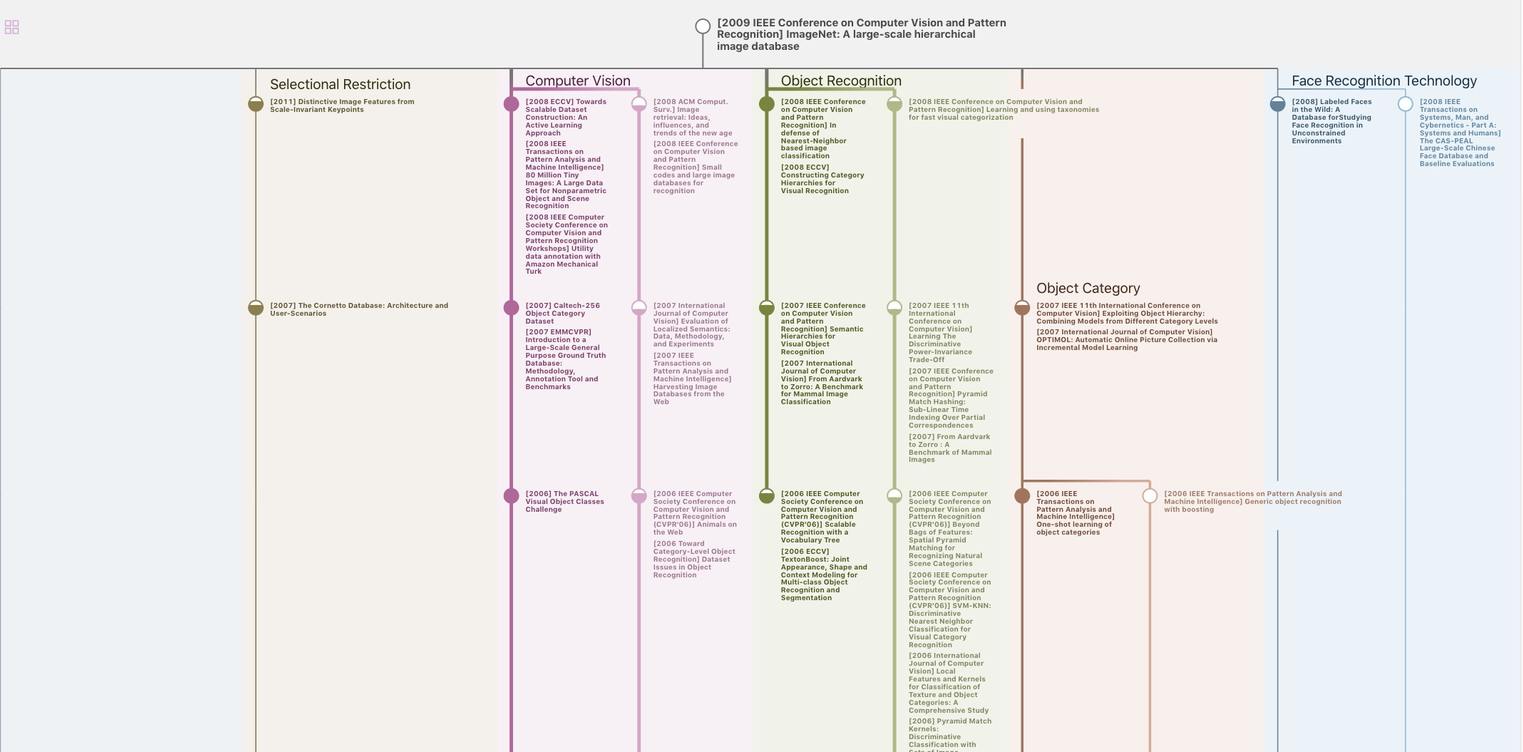
生成溯源树,研究论文发展脉络
Chat Paper
正在生成论文摘要