Canonicalizing Organization Names For Recruitment Domain
PROCEEDINGS OF THE 7TH ACM IKDD CODS AND 25TH COMAD (CODS-COMAD 2020)(2020)
摘要
Online recruitment industry relies on various Knowledge Bases (KB) for enabling search and recommendation systems. These KBs comprise of diverse, non-standard, and large volume of namedentities as they are created from vast unstructured user-generated content (mostly CVs). Such non-standard representation of each entity causes significant vocabulary gap in KB which results in redundancy, incompleteness, and ambiguity in the retrieved information. The problem is even more challenging in domains where external sources of context do not exist.To address these challenges, we propose a two-tier architecture that (a) finds the distance parameter for clustering entities using a novel pairwise similarity between all entity mentions, and, (b) then uses these similarity (scores) to create canonical clusters representing unique entity in the KB. Our experiments on proprietary data of 25,602 unique companies and 23,690 unique institutes show that the pair-wise similarity score using Siamese network outperforms (97% and 82% F1-score) standard string similarity measures. Finally, clustering methods over the similarity scores achieve 90% and 80% micro F1-score.
更多查看译文
关键词
datasets, neural networks, gaze detection, text tagging
AI 理解论文
溯源树
样例
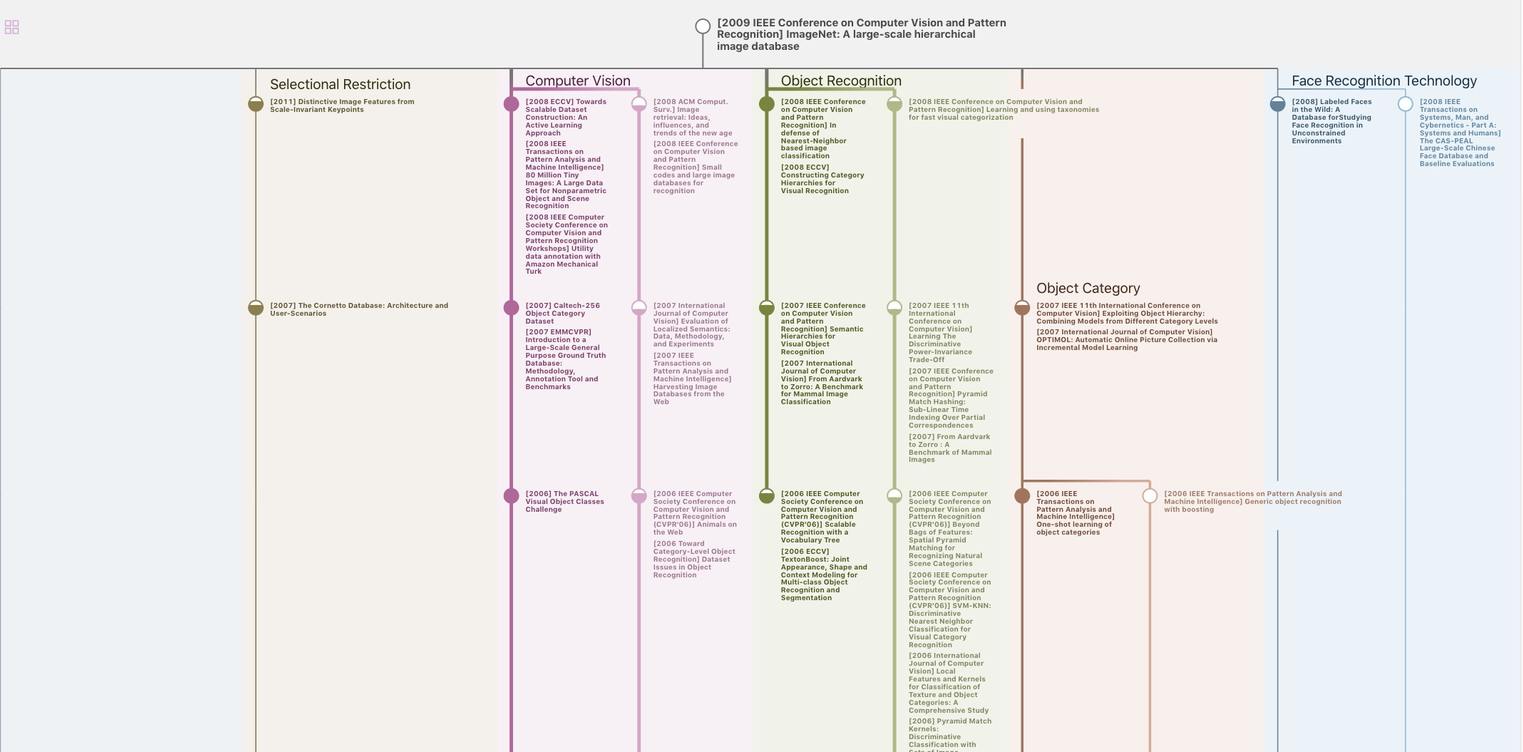
生成溯源树,研究论文发展脉络
Chat Paper
正在生成论文摘要