RPN: A Residual Pooling Network for Efficient Federated Learning
ECAI 2020: 24TH EUROPEAN CONFERENCE ON ARTIFICIAL INTELLIGENCE(2020)
摘要
Federated learning is a new machine learning framework which enables different parties to collaboratively train a model while protecting data privacy and security. Due to model complexity, network unreliability and connection in-stability, communication cost has became a major bottleneck for applying federated learning to real-world applications. Current existing strategies are either need to manual setting for hyper-parameters, or break up the original process into multiple steps, which make it hard to realize end-to-end implementation. In this paper, we propose a novel compression strategy called Residual Pooling Network (RPN). Our experiments show that RPN not only reduce data transmission effectively, but also achieve almost the same performance as compared to standard federated learning. Our new approach performs as an end-to-end procedure, which should be readily applied to all CNN-based model training scenarios for improvement of communication efficiency, and hence make it easy to deploy in real-world application without human intervention.
更多查看译文
关键词
efficient federated learning,residual pooling network,rpn
AI 理解论文
溯源树
样例
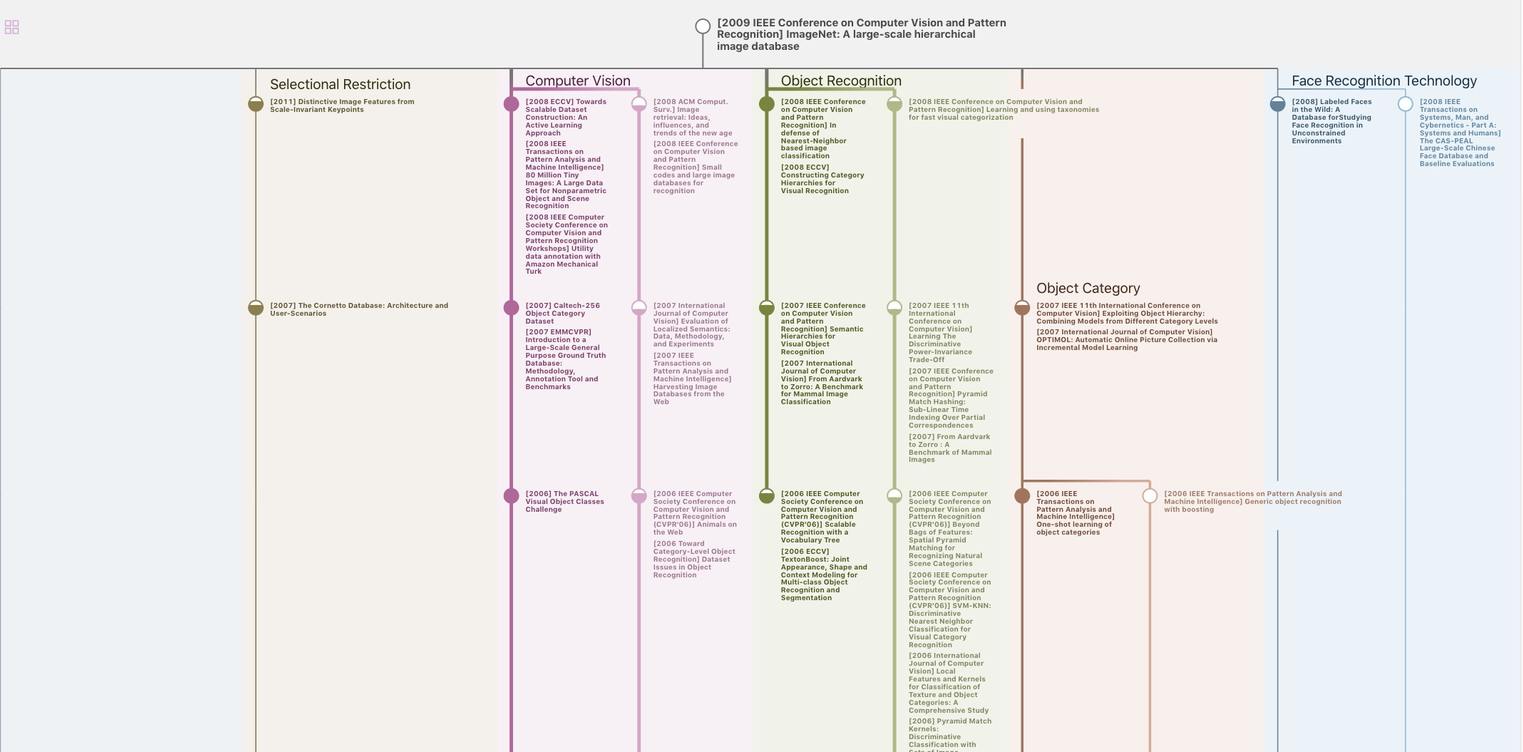
生成溯源树,研究论文发展脉络
Chat Paper
正在生成论文摘要