Gradient and Hessian approximations in Derivative Free Optimization
arxiv(2020)
摘要
This work investigates finite differences and the use of interpolation models to obtain approximations to the first and second derivatives of a function. Here, it is shown that if a particular set of points is used in the interpolation model, then the solution to the associated linear system (i.e., approximations to the gradient and diagonal of the Hessian) can be obtained in $\mathcal{O}(n)$ computations, which is the same cost as finite differences, and is a saving over the $\mathcal{O}(n^3)$ cost when solving a general unstructured linear system. Moreover, if the interpolation points are formed using a `regular minimal positive basis', then the error bound for the gradient approximation is the same as for a finite differences approximation. Numerical experiments are presented that show how the derivative estimates can be employed within an existing derivative free optimization algorithm, thus demonstrating one of the potential practical uses of these derivative approximations.
更多查看译文
AI 理解论文
溯源树
样例
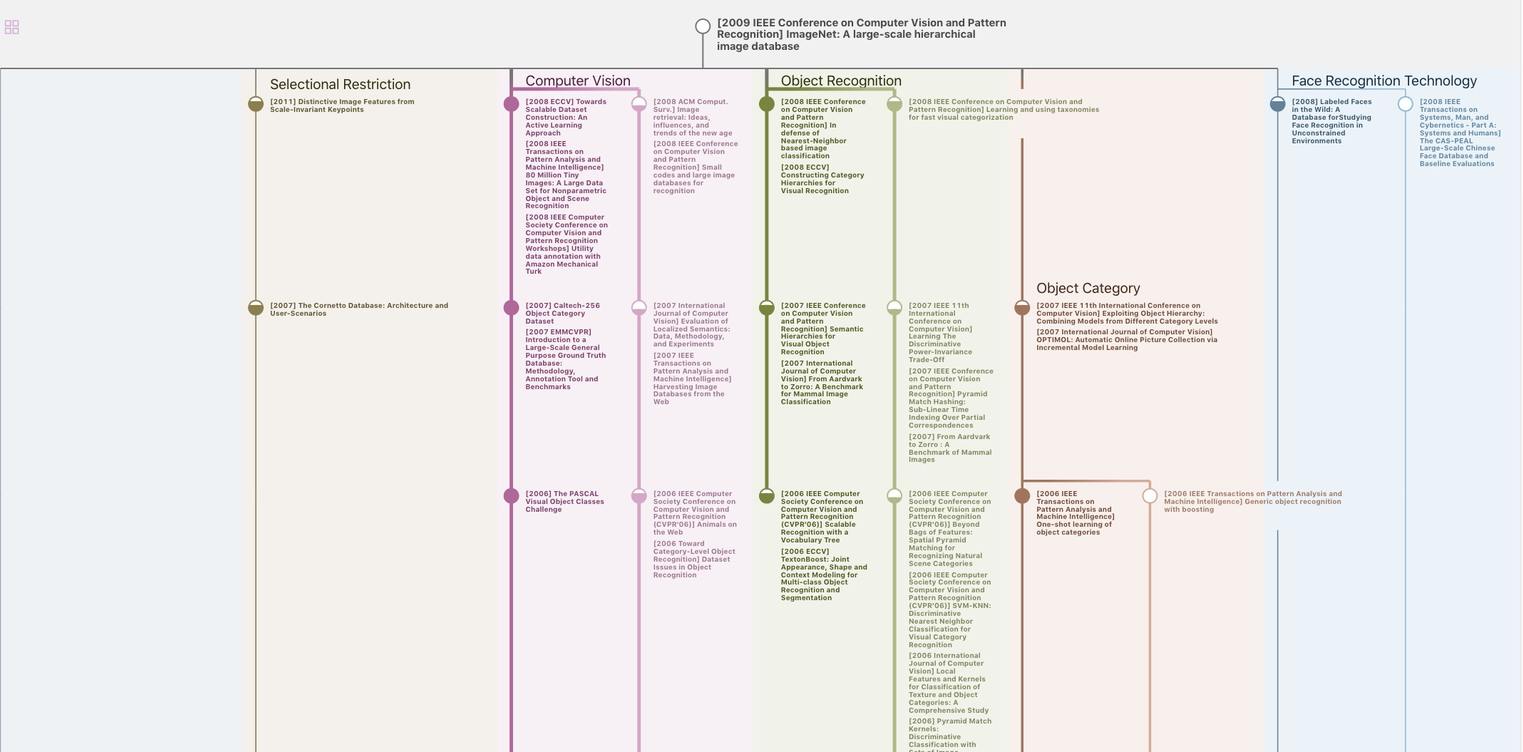
生成溯源树,研究论文发展脉络
Chat Paper
正在生成论文摘要