Loss Function Optimization Based on Adversarial Networks.
Frontiers in Artificial Intelligence and Applications(2019)
摘要
Autoencoder networks have been successfully applied to dimensionality reduction and information retrieval tasks. Lower-dimensional representations can improve performance on many tasks, such as image compression, reconstruction and clustering. The Mean Square Error (MSE) is commonly used as a loss function for autoencoder networks. The loss function suffers from performance degradation because it lacks the component distribution information of the input image. In the deep learning literature, recent works have shown the benefits of using adversarialbased losses to improve the performance on various image reconstruction and clustering tasks. This paper proposes a new algorithm which is called GAN-WMSE to generate weights for the MSE based on adversarial networks. With the distribution information integrated into the loss function, the autoencoder network and the adversarial weight network are jointly trained. Experiments on different image datasets show that the improved autoencoder networks employing our loss function can increase performance by 9.8% for PSNR, 10.3% for SSIM in image reconstruction, and 6.2% for image clustering.
更多查看译文
关键词
Adversarial networks,Autoencoder network,Image clustering,Image reconstruction,Loss function
AI 理解论文
溯源树
样例
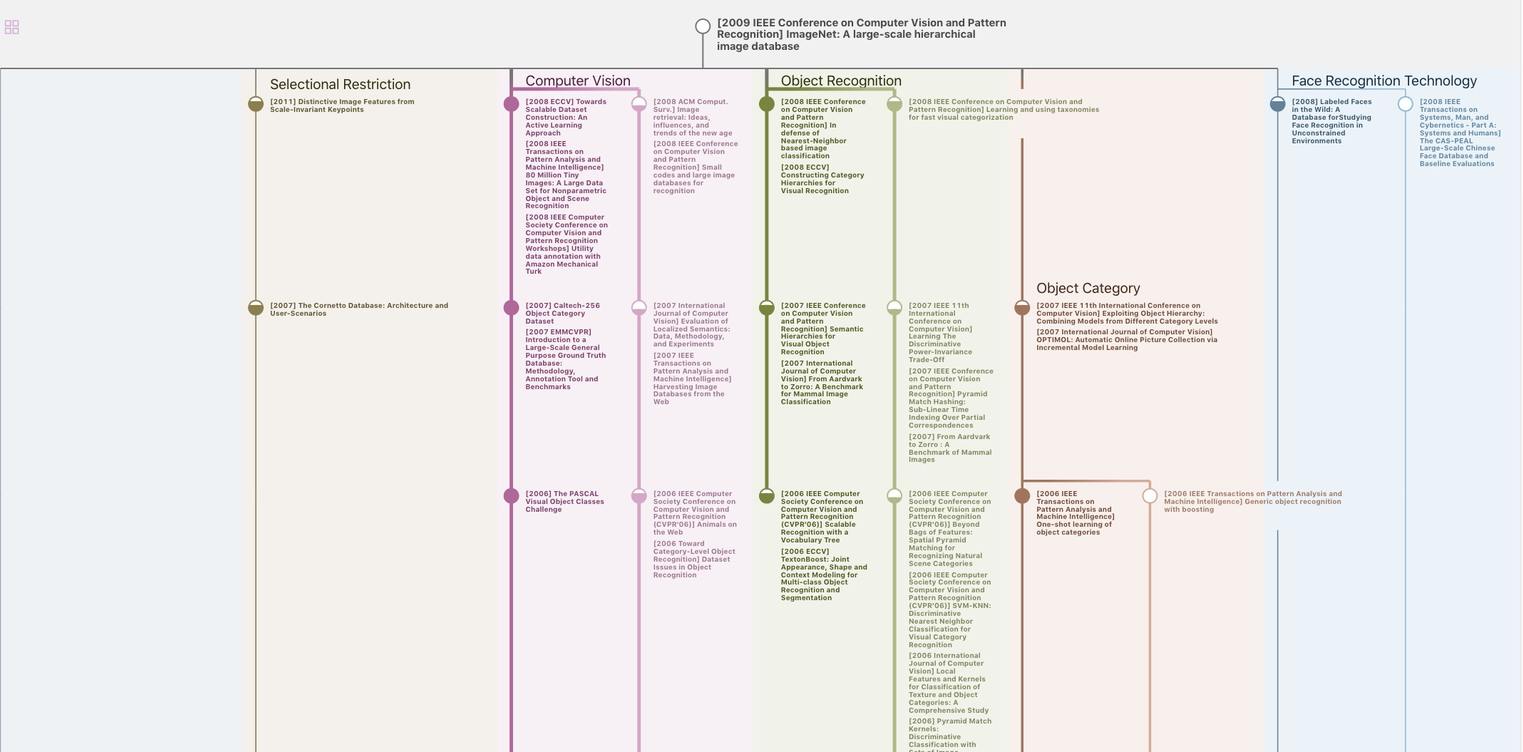
生成溯源树,研究论文发展脉络
Chat Paper
正在生成论文摘要