A Novel Framework for Anomaly Detection via Feature Selection and Dimensionality Reduction.
Frontiers in Artificial Intelligence and Applications(2019)
摘要
Anomaly detection of network traffic data is vital to the early warning of anomalous behaviors and the precaution of malicious intrusions. We consider a scenario, in which the network traffic data have large volumes and high dimensionality, and follow different patterns. To detect anomalies accurately and efficiently, a K-means and Bayesian inference based Conditional Random Fields (KBCRF) method is proposed here. Specifically, we first adopt a K-clustering method to categorize the network data into manifold classes so as to alleviate the interference from data with different patterns. Also, we propose a data reduction method via Bayesian inference to filter irrelevant data features, hence improving the performance of data training and anomaly detection. Then, we propose a Conditional Random Fields based method to determine the anomalies in the network data, upon the conditional probability of anomaly given the data distribution. For cyber security maintenance, KBCRF method provides more accurate and faster anomaly detection, compared with existing detection methods. The performance of the proposed method is evaluated by the ISCX 2012 dataset. Experimental results demonstrate the validation and efficiency of the proposed method in terms of precision, recall and F1-score.
更多查看译文
关键词
anomaly detection,machine learning,data clustering,dimensionality reduction,K-clustering,Bayesian inference,Conditional Random Fields
AI 理解论文
溯源树
样例
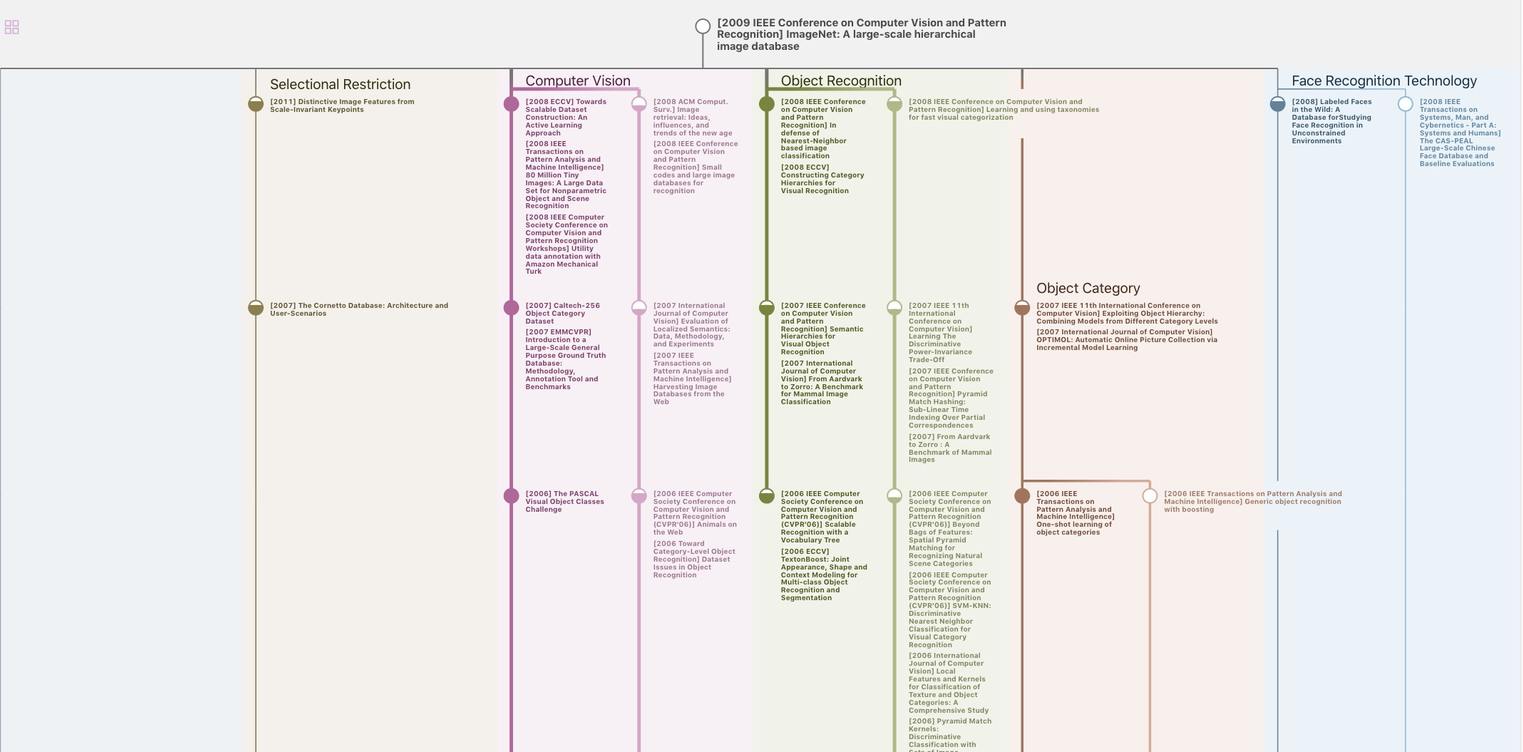
生成溯源树,研究论文发展脉络
Chat Paper
正在生成论文摘要