Exploring Model Transfer Potential For Airborne Lidar Point Cloud Classification
PATTERN RECOGNITION AND ARTIFICIAL INTELLIGENCE(2020)
摘要
The deep learning paradigm has been shown to be an effective framework in many applications, including airborne light detection and ranging point cloud classification. However, even a simple deep neural network has large quantities of parameters, and the optimal parameters generally need several hours to be learned. In this paper, we propose a framework to take full advantage of existing deep neural networks in image processing domains and to reduce the training time for classification. The framework is composed of four key steps: (1) calculate low-level features; (2) transform three-dimensional point clouds into multi-scale feature images by the proposed feature image generation strategy; (3) extract multi-scale deep features from the feature images by introducing transfer learning, i.e., a pre-trained neural network; and (4) learn higher-level features via a fully connected network and fuse higher-level features using a convolutional neural network. Our framework has been evaluated using a benchmark dataset provided by the International Society for Photogrammetry and Remote Sensing, and experimental results show that the proposed framework can reduce the time needed for obtaining an optimal classification model and effectively classify nine objects, such as buildings, the ground, and cars, with an overall accuracy of 90.1%, which is beneficial for providing reliable information for further applications.
更多查看译文
关键词
Transfer learning, Classification, Feature image, Airborne LiDAR point cloud, Multi-scale, High-level features
AI 理解论文
溯源树
样例
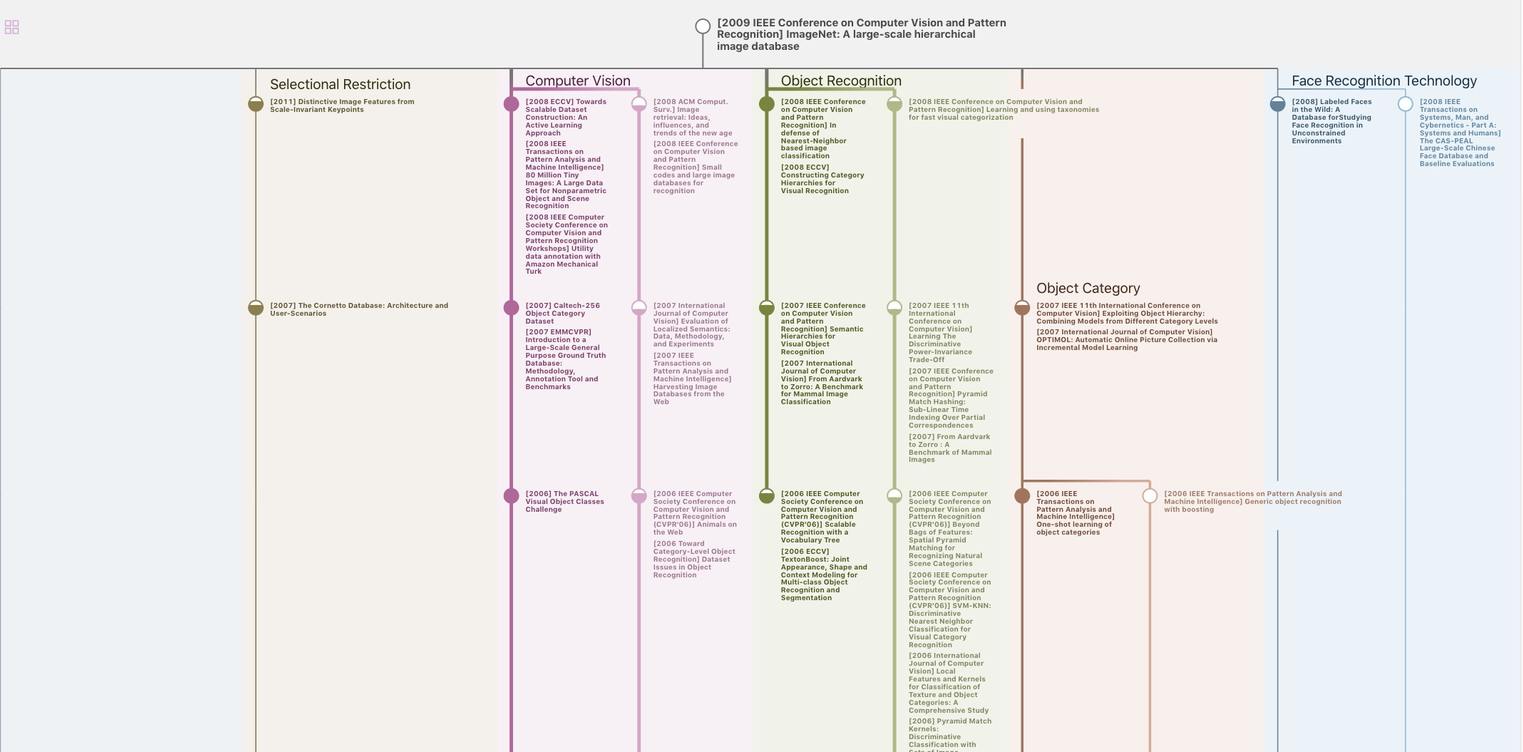
生成溯源树,研究论文发展脉络
Chat Paper
正在生成论文摘要