A Fast Privacy-Preserving Multi-Layer Perceptron Using Ring-LWE-Based Homomorphic Encryption
2019 International Conference on Data Mining Workshops (ICDMW)(2019)
摘要
Concerns about leaking privacy from data have been preventing from making good use of so-called big data, while privacy-preserving data analysis would still be a promising research direction. In this paper, we propose Privacy-Preserving Multi-Layer Perceptron (PP-MLP) that can compute the prediction real-time using Ring-LWE-based homomorphic encryption. We implement the proposed PP-MLP in the form of a two-party model consisting of client and server. The former encrypts input data and receives a classification result from a server, and the latter performs prediction over encrypted data. This scheme enables a client to acquire prediction without revealing actual data contents against a server. The proposed PP-MLP can make a fast prediction that requires up to 80 msec per input without a significant drop in classification accuracy compared to the convention multi-layer perceptron for plaintexts.
更多查看译文
关键词
privacy-preserving data mining,neural network,homomorphic encryption
AI 理解论文
溯源树
样例
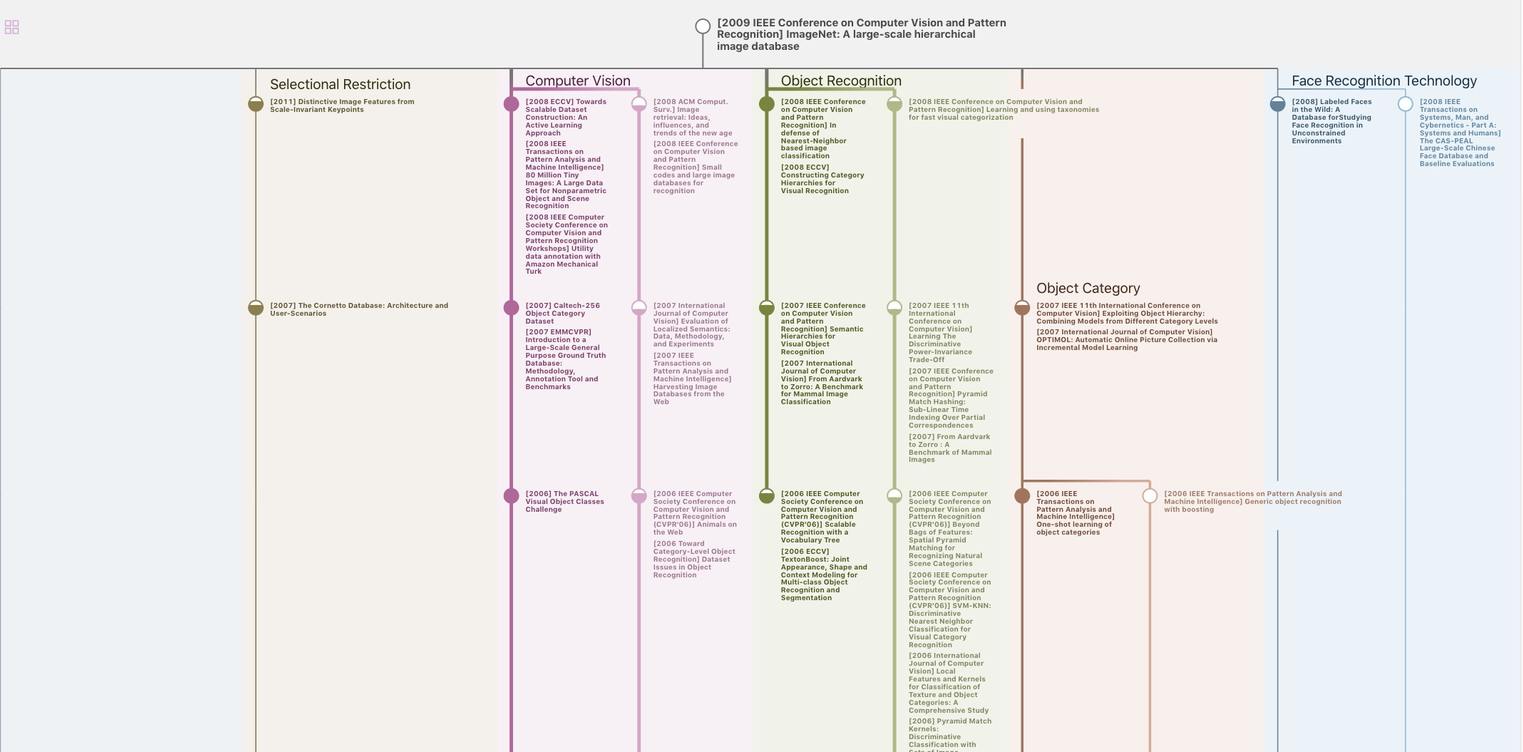
生成溯源树,研究论文发展脉络
Chat Paper
正在生成论文摘要