Unsupervised Robust Nonparametric Learning Of Hidden Community Properties
MATHEMATICAL FOUNDATIONS OF COMPUTING(2019)
摘要
We consider learning of fundamental properties of communities in large noisy networks, in the prototypical situation where the nodes or users are split into two classes according to a binary property, e.g., according to their opinions or preferences on a topic. For learning these properties, we propose a nonparametric, unsupervised, and scalable graph scan procedure that is, in addition, robust against a class of powerful adversaries. In our setup, one of the communities can fall under the influence of a knowledgeable adversarial leader, who knows the full network structure, has unlimited computational resources and can completely foresee our planned actions on the network. We prove strong consistency of our results in this setup with minimal assumptions. In particular, the learning procedure estimates the baseline activity of normal users asymptotically correctly with probability 1; the only assumption being the existence of a single implicit community of asymptotically negligible logarithmic size. We provide experiments on real and synthetic data to illustrate the performance of our method, including examples with adversaries.
更多查看译文
关键词
Nonparametric learning, unsupervised learning, hidden community, scan estimator, community properties, learning for networks, adversarial learning, non-sparse graphs, crawler, scalability
AI 理解论文
溯源树
样例
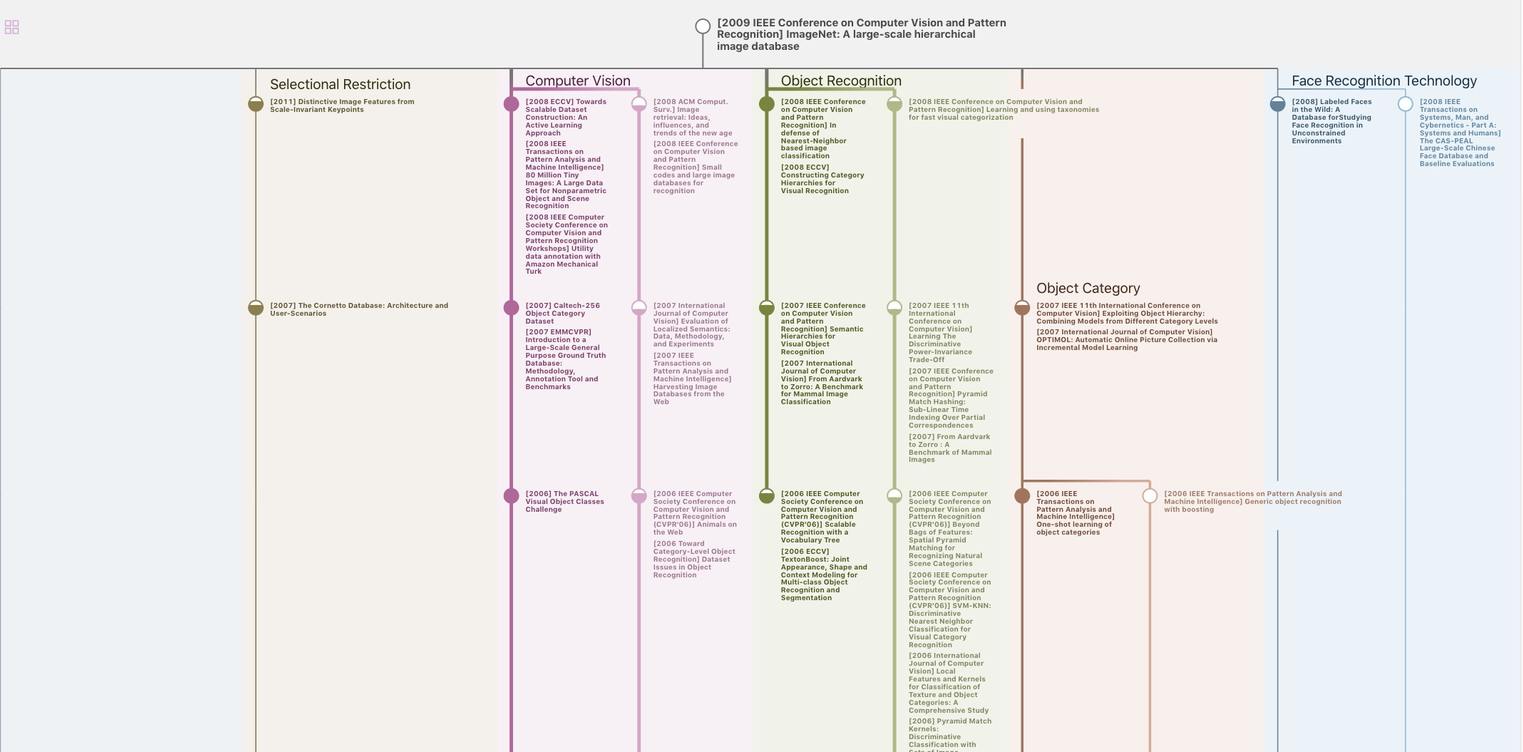
生成溯源树,研究论文发展脉络
Chat Paper
正在生成论文摘要