A Deep Model for Lung Cancer Type Identification by Densely Connected Convolutional Networks and Adaptive Boosting.
IEEE ACCESS(2020)
摘要
Timely diagnosis and determination to the type of lung cancer has important clinical significance. Generally, it requires multiple imaging methods to complement each other to obtain a comprehensive diagnosis. In this work, we propose a deep learning model to identify lung cancer type from CT images for patients in Shandong Provincial Hospital. It has a two-fold challenge: artificial intelligent models trained by public datasets cannot meet such practical requires, and the amount of collected patients' data is quite few. To solve the two-fold problem, we use image rotation, translation and transformation methods to expand and balance our training data, and then densely connected convolutional networks (DenseNet) is used to classify malignant tumor from images collected from, and finally adaptive boosting (adaboost) algorithm is used to aggregate multiple classification results to improve classification performance. Experimental results show that our method can achieve identifying accuracy 89:85%, which performs better than DenseNet without adaboost, ResNet, VGG16 and AlexNet. This provides an efficient, non-invasive detection tool for pathological diagnosis to lung cancer type.
更多查看译文
关键词
Adaboost algorithm,data enhancement,densely connected convolutional networks,lung cancer
AI 理解论文
溯源树
样例
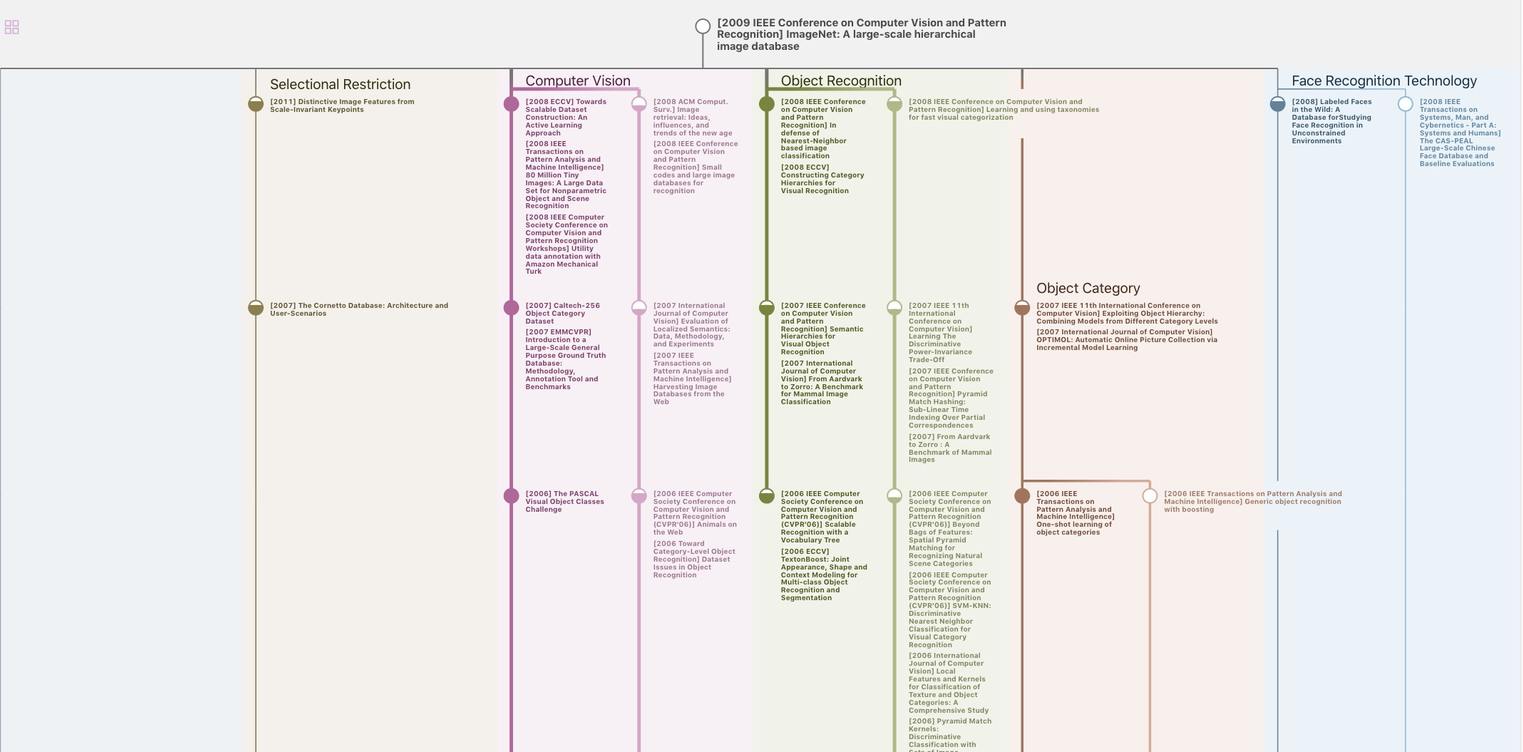
生成溯源树,研究论文发展脉络
Chat Paper
正在生成论文摘要