Challenges in Task Incremental Learning for Assistive Robotics.
IEEE ACCESS(2020)
摘要
Recent breakthroughs in computer vision areas, ranging from detection, segmentation, to classification, rely on the availability of large-scale representative training datasets. Yet, robotic vision poses new challenges towards applying visual algorithms developed from these datasets because the latter implicitly assume a fixed set of categories and time-invariant distribution of tasks. In practice, assistive robots should be able to operate in dynamic environments with everyday changes. The variations of four commonly observed factors, including illumination, occlusion, camera-object distance/angles and clutter, could make lifelong/continual learning in computer vision more challenging. Large-scale datasets previously made publicly available were relatively simple, and rarely include such real-world challenges in data collection. Benefited from the recent released OpenLORIS-Object dataset, which explicitly includes these real-world challenges in the lifelong object recognition task, we evaluate three most adopted regularization methods in lifelong/continual learning (Learning without Forgetting, Elastic Weights Consolidation, and Synaptic Intelligence). Their performances were compared with the naive and cumulative training modes as the lower bound and upper bound of performances, respectively. The experiments conducted on the dataset focused on task incremental learning, i.e., incremental difficulty based on the four environment of factors. However, all the three most reported lifelong/continual learning algorithms have failed with the increase in encountered batches across various metrics with indistinguishable performance comparing to the naive training mode. Our results highlight the current challenges in lifelong object recognition for assistive robots to operate in real-world dynamic scene.
更多查看译文
关键词
Task analysis,Rehabilitation robotics,Lighting,Clutter,Computer vision,Training,Machine intelligence,robotic vision systems
AI 理解论文
溯源树
样例
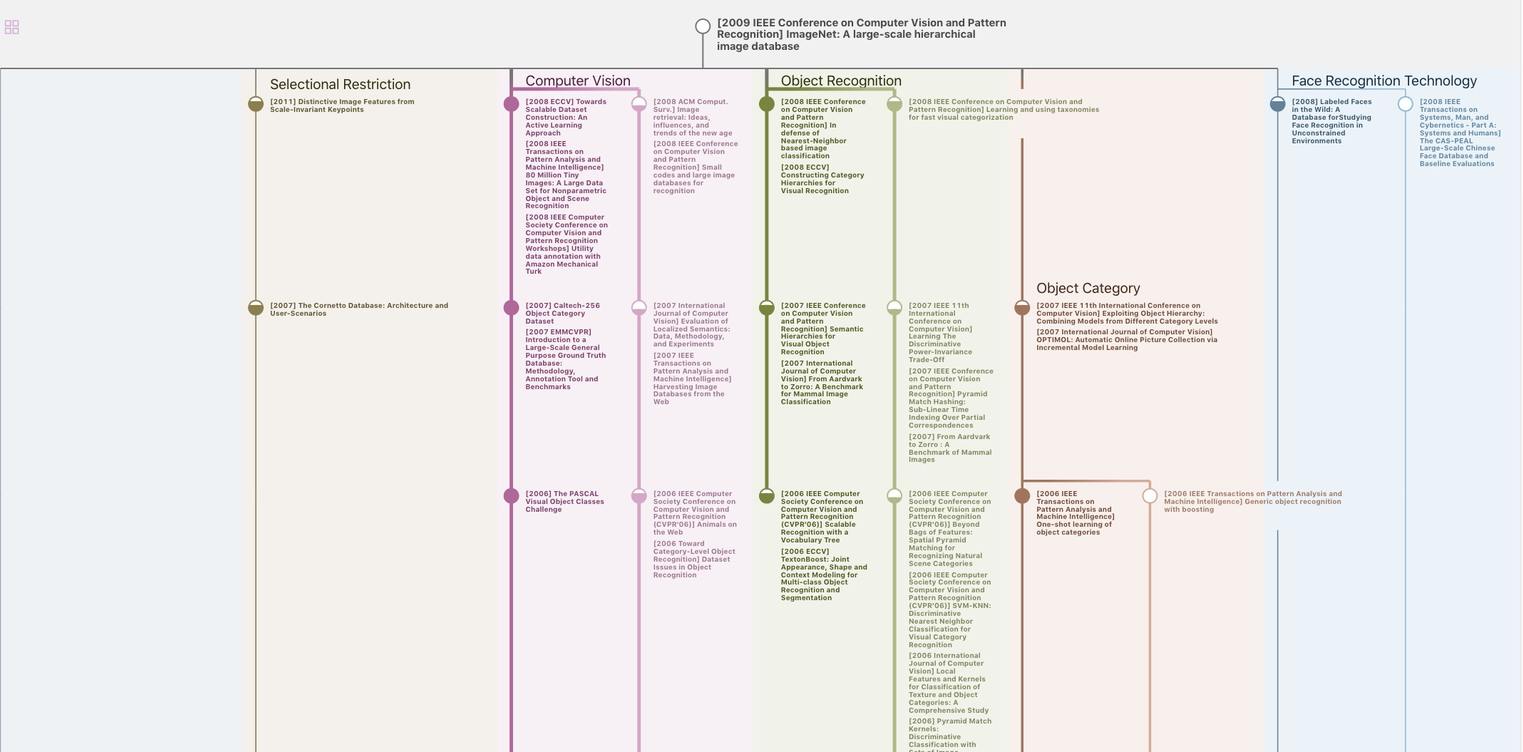
生成溯源树,研究论文发展脉络
Chat Paper
正在生成论文摘要