Adversarial Attack on Community Detection by Hiding Individuals
WWW '20: The Web Conference 2020 Taipei Taiwan April, 2020(2020)
摘要
It has been demonstrated that adversarial graphs, i.e., graphs with imperceptible perturbations added, can cause deep graph models to fail on node/graph classification tasks. In this paper, we extend adversarial graphs to the problem of community detection which is much more difficult. We focus on black-box attack and aim to hide targeted individuals from the detection of deep graph community detection models, which has many applications in real-world scenarios, for example, protecting personal privacy in social networks and understanding camouflage patterns in transaction networks. We propose an iterative learning framework that takes turns to update two modules: one working as the constrained graph generator and the other as the surrogate community detection model. We also find that the adversarial graphs generated by our method can be transferred to other learning based community detection models.
更多查看译文
关键词
adversarial attack, community detection, graph generation
AI 理解论文
溯源树
样例
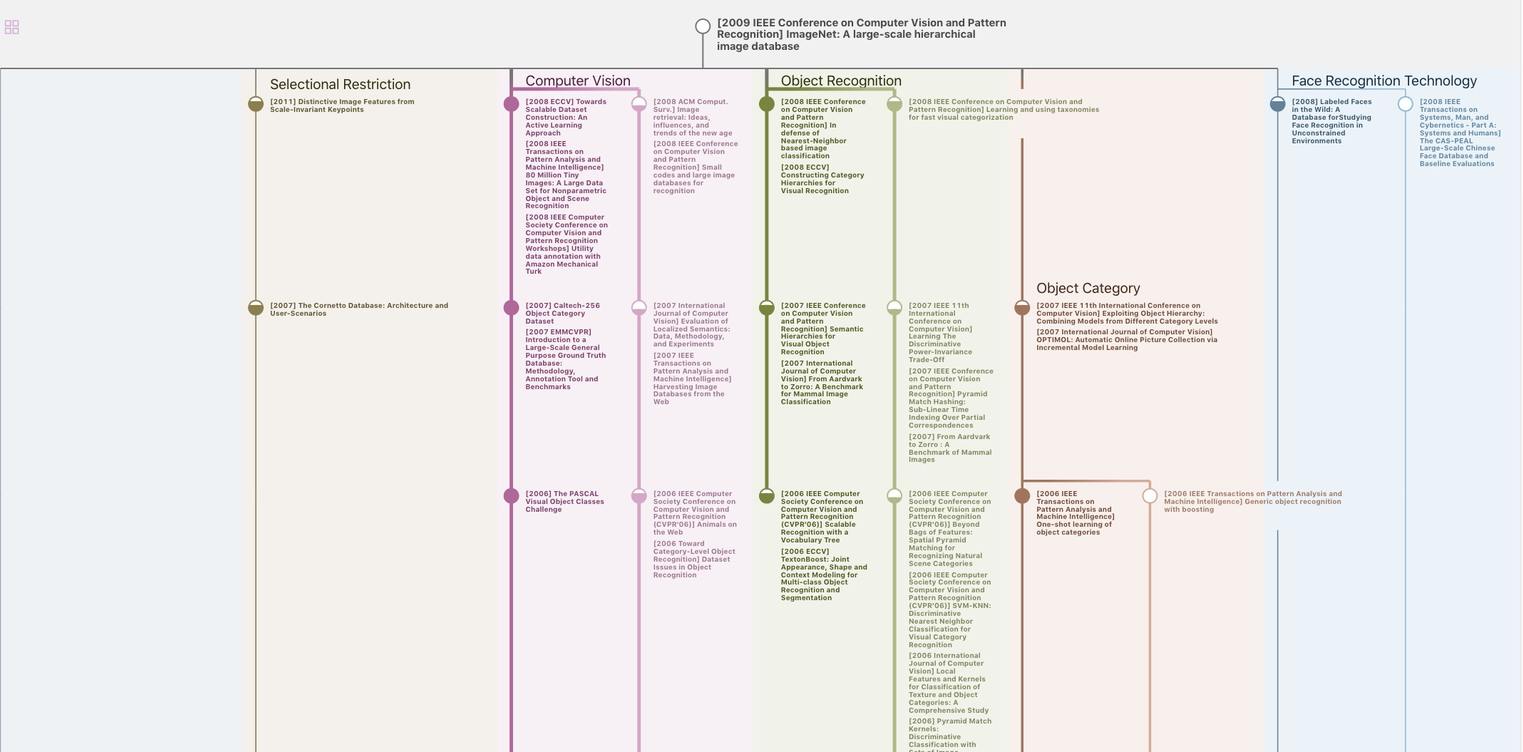
生成溯源树,研究论文发展脉络
Chat Paper
正在生成论文摘要