Distributed Possibilistic Learning in Multi-Agent Systems
arxiv(2020)
摘要
Possibility theory is proposed as an uncertainty representation framework for distributed learning in multi-agent systems and robot swarms. In particular, we investigate its application to the best-of-n problem where the aim is for a population of agents to identify the highest quality out of n options through local interactions between individuals and limited direct feedback from the environment. In this context we claim that possibility theory provides efficient mechanisms by which an agent can learn about the state of the world, and which can allow them to handle inconsistencies between what they and others believe by varying the level of imprecision of their own beliefs. We introduce a discrete time model of a population of agents applying possibility theory to the best-of-n problem. Simulation experiments are then used to investigate the accuracy of possibility theory in this context as well as its robustness to noise under varying amounts of direct evidence. Finally, we compare possibility theory in this context with a similar probabilistic approach.
更多查看译文
关键词
possibilistic learning,systems,multi-agent
AI 理解论文
溯源树
样例
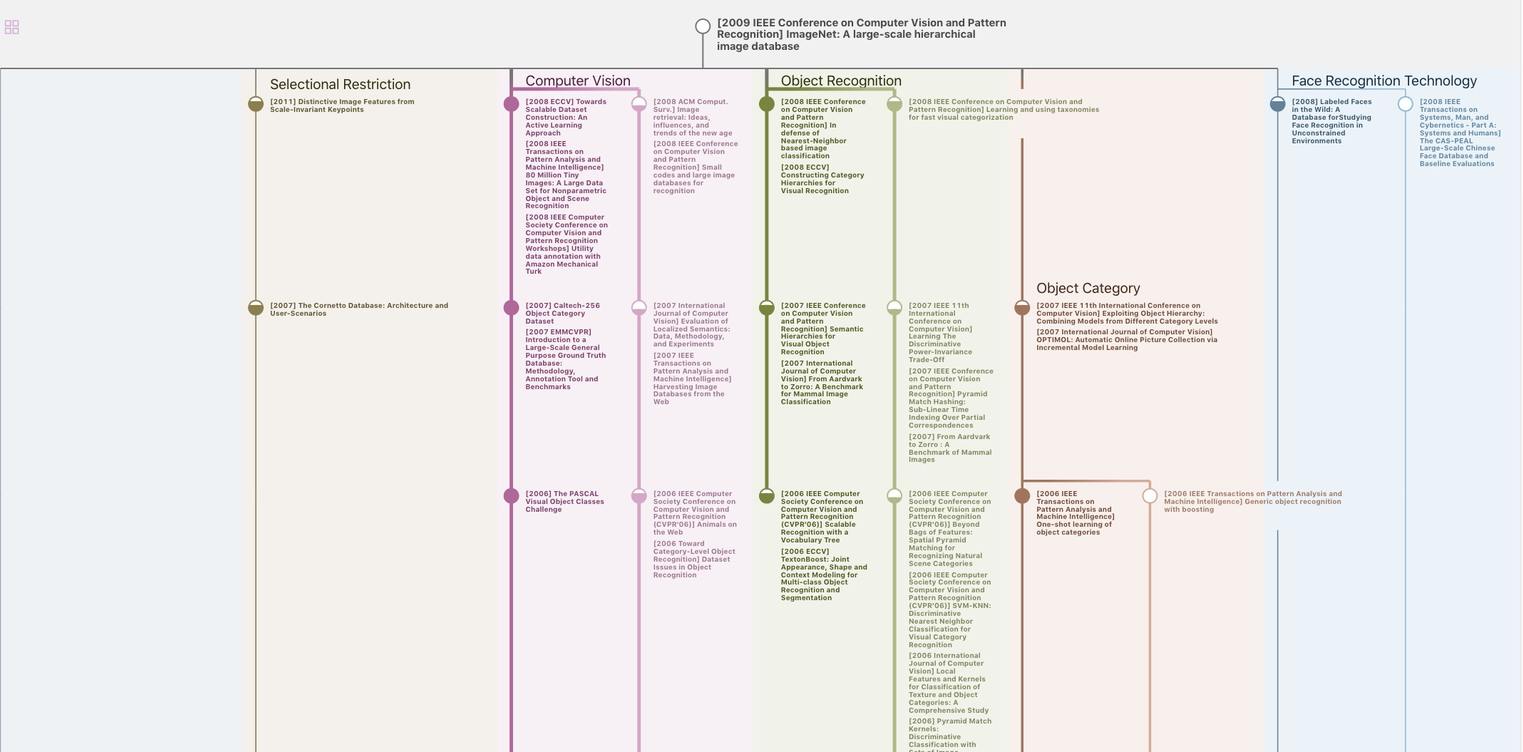
生成溯源树,研究论文发展脉络
Chat Paper
正在生成论文摘要