Data-Driven Ensembles for Deep and Hard-Decision Hybrid Decoding
2020 IEEE International Symposium on Information Theory (ISIT)(2020)
摘要
Ensemble models are widely used to solve complex tasks by their decomposition into multiple simpler tasks, each one solved locally by a single member of the ensemble. Decoding of error-correction codes is a hard problem due to the curse of dimensionality, leading one to consider ensembles-of-decoders as a possible solution. Nonetheless, one must take complexity into account, especially in decoding. We suggest a low-complexity scheme where a single member participates in the decoding of each word. First, the distribution of feasible words is partitioned into non-overlapping regions. Thereafter, specialized experts are formed by independently training each member on a single region. A classical hard-decision decoder (HDD) is employed to map every word to a single expert in an injective manner. FER gains of up to 0.4dB at the waterfall region, and of 1.25dB at the error floor region are achieved for two BCH(63,36) and (63,45) codes with cycle-reduced parity-check matrices, compared to the previous best result of [1].
更多查看译文
关键词
Deep Learning,Error Correcting Codes,Machine-Learning,Ensembles,Belief Propagation
AI 理解论文
溯源树
样例
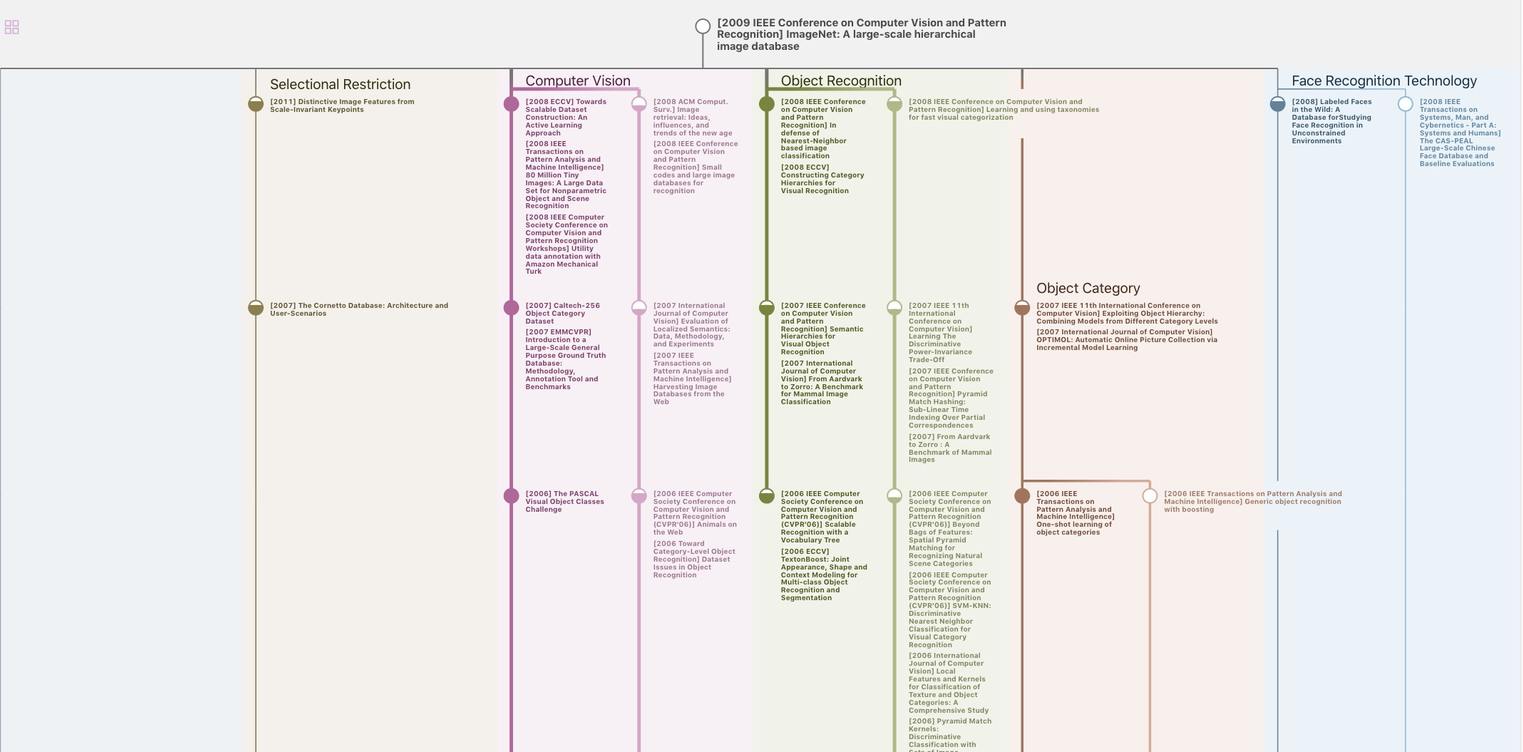
生成溯源树,研究论文发展脉络
Chat Paper
正在生成论文摘要