A content-based deep intrusion detection system
International Journal of Information Security(2021)
摘要
The growing number of Internet users and the prevalence of web applications make it necessary to deal with very complex software and applications in the network. This results in an increasing number of new vulnerabilities in the systems, and leading to an increase in cyber threats and, in particular, zero-day attacks. The cost of generating appropriate signatures for these attacks is a potential motive for using machine learning-based methodologies. Although there are many studies on using learning-based methods for attack detection, they generally use extracted features and overlook raw contents. This approach can lessen the performance of detection systems against content-based attacks like SQL injection, Cross-site Scripting (XSS), and various viruses. In this work, we propose a framework, called deep intrusion detection (DID) system, that uses the pure content of traffic flows in addition to traffic metadata in the learning and detection phases of a passive DNN IDS. To this end, we deploy and evaluate an offline IDS following the framework using LSTM as a deep learning technique. Due to the inherent nature of deep learning, it can process high-dimensional data content and, accordingly, discover the sophisticated relations between the auto extracted features of the traffic. To evaluate the proposed DID system, we use the CIC-IDS2017 and CSE-CIC-IDS2018 datasets. The evaluation metrics, such as precision and recall, reach 0.992 and 0.998 on CIC-IDS2017, and 0.933 and 0.923 on CSE-CIC-IDS2018, respectively, which show the high performance of the proposed DID method.
更多查看译文
关键词
Deep learning,Intrusion detection,Content-based attacks,Recurrent neural networks,Long short-term memory,Machine learning,Misuse,Malware detection,DoS attacks
AI 理解论文
溯源树
样例
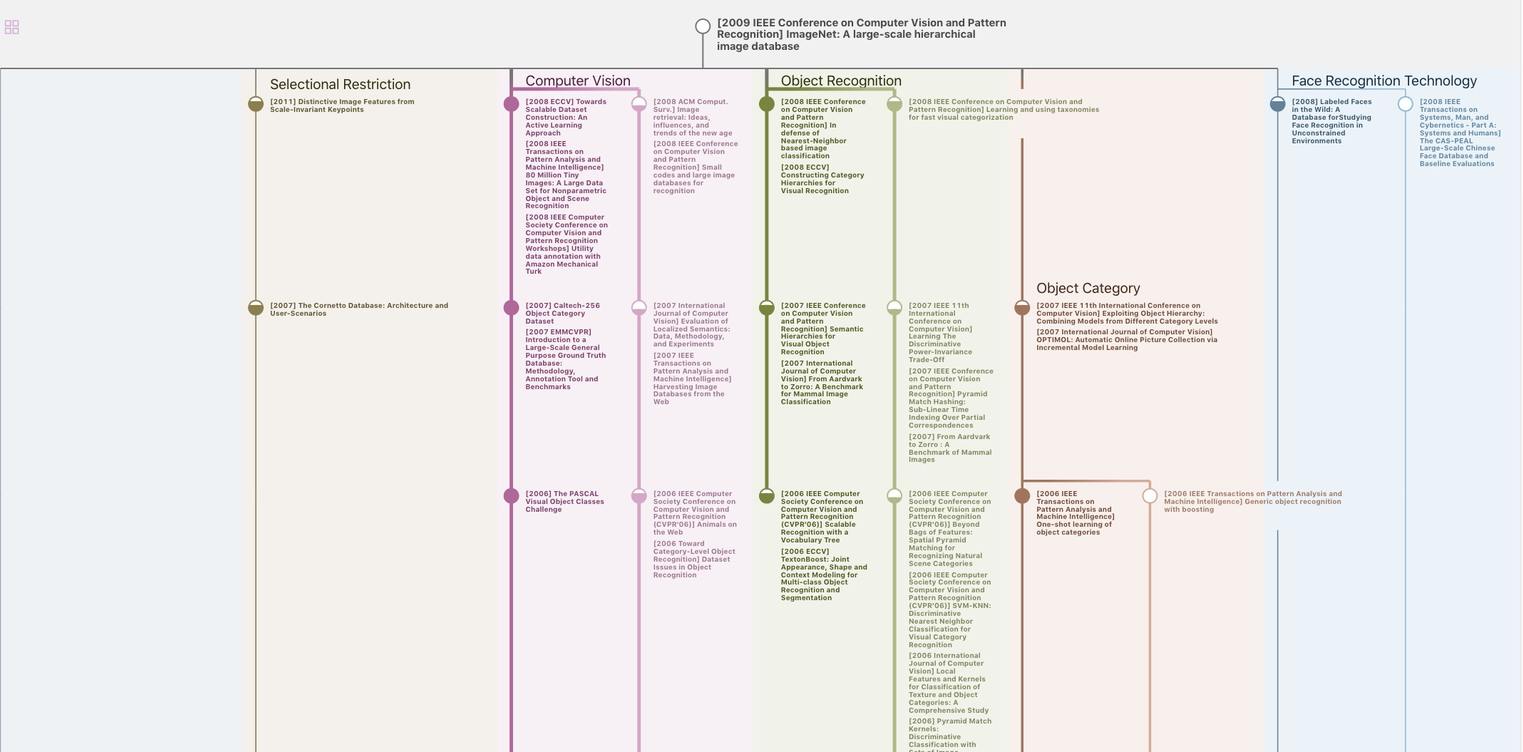
生成溯源树,研究论文发展脉络
Chat Paper
正在生成论文摘要