ProphetNet: Predicting Future N-gram for Sequence-to-Sequence Pre-training
EMNLP(2020)
摘要
In this paper, we present a new sequence-to-sequence pre-training model called ProphetNet, which introduces a novel self-supervised objective named future n-gram prediction and the proposed n-stream self-attention mechanism.Instead of the optimization of one-step ahead prediction in traditional sequence-to-sequence model, the ProphetNet is optimized by n-step ahead prediction which predicts the next n tokens simultaneously based on previous context tokens at each time step.The future n-gram prediction explicitly encourages the model to plan for the future tokens and prevent overfitting on strong local correlations. We pre-train ProphetNet using a base scale dataset (16GB) and a large scale dataset (160GB) respectively. Experimental results show ProphetNet achieves the best performance on both abstractive summarization and question generation tasks compared to the models using the same base scale pre-training dataset. For the large scale dataset pre-training, ProphetNet achieves new state-of-the-art results on Gigaword and comparable results on CNN/DailyMail using only about 1/5 pre-training epochs of the previous model.
更多查看译文
关键词
future,n-gram,sequence-to-sequence,pre-training
AI 理解论文
溯源树
样例
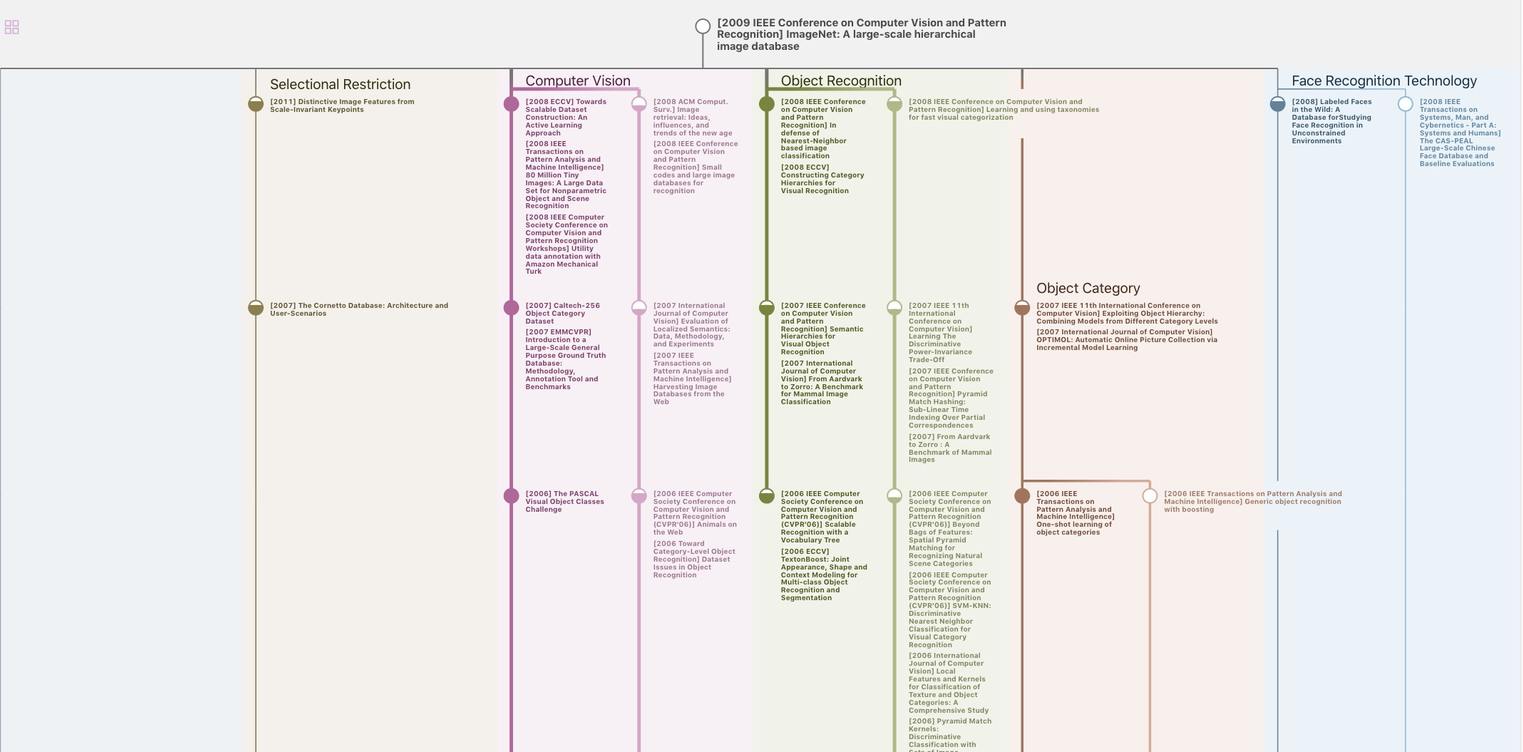
生成溯源树,研究论文发展脉络
Chat Paper
正在生成论文摘要