Attribute-Guided Feature Learning Network for Vehicle Reidentification
IEEE MultiMedia(2020)
摘要
Vehicle reidentification (reID) targets at matching same vehicle images captured from multicameras, which has become a hot topic in recent years. However, it poses the critical but challenging problem that is caused by complicated environments and diversified illuminations. Inspired by the benefit of attributes that contain detailed descriptions, this article proposes a novel attribute-guided network (AGNet), which learns global representation with the abundant attribute features in an end-to-end manner. Specially, an attribute-guided module is proposed in AGNet to generate the attribute mask (AttrMask), which inversely guides to select discriminative features for category classification. Moreover, in AGNet, an attribute-based label smoothing (ASL) loss is presented to better train the reID model, which can strengthen the distinct ability of the reID model and regularize the AGNet model according to the attributes. The experiment results validate the effectiveness of AGNet and demonstrate that AGNet achieves excellent performance on two benchmark datasets.
更多查看译文
关键词
Vehicle Re-identification,Attribute-guided Model,Attribute-based Label Smoothing Loss
AI 理解论文
溯源树
样例
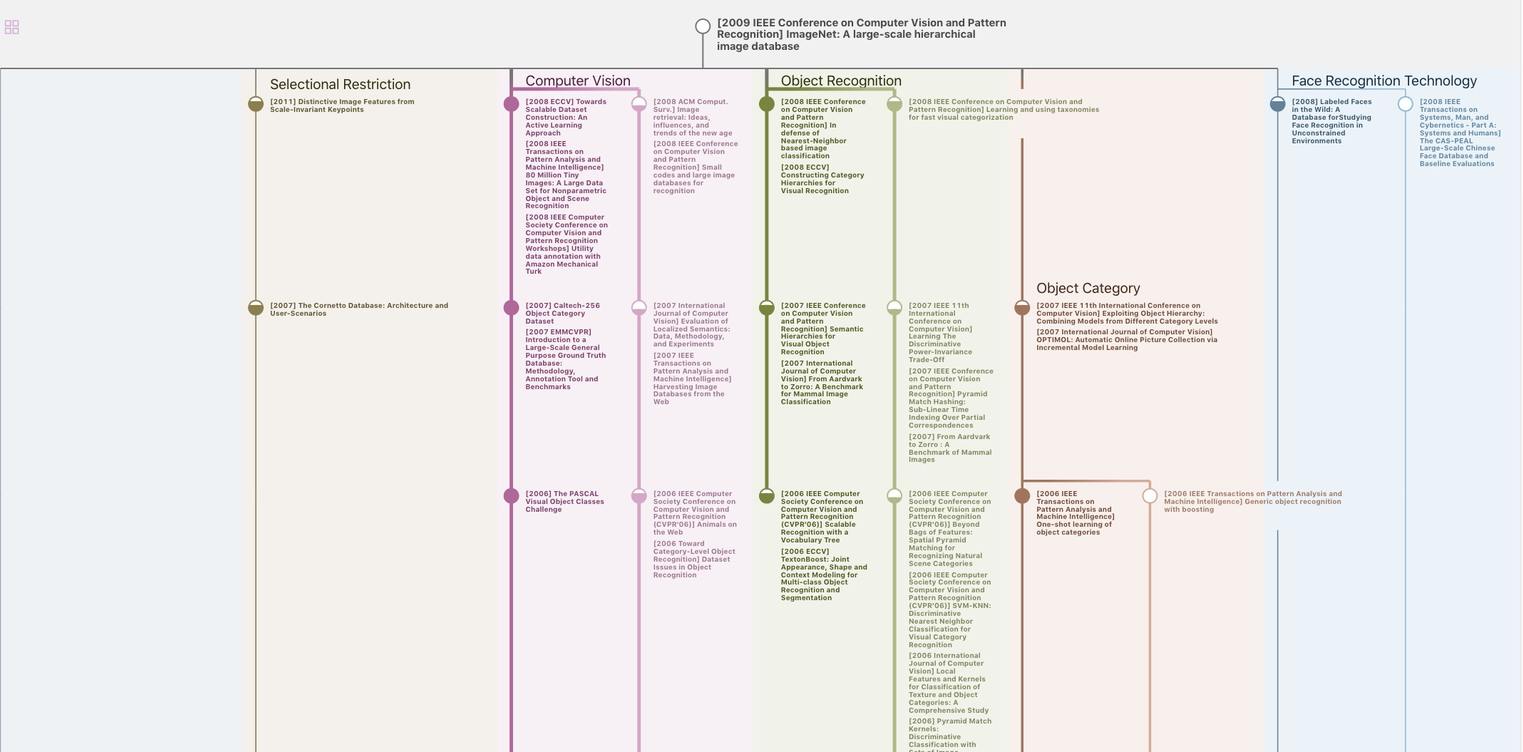
生成溯源树,研究论文发展脉络
Chat Paper
正在生成论文摘要