Robust Fake News Detection Over Time and Attack
ACM Transactions on Intelligent Systems and Technology (TIST)(2020)
摘要
In this study, we examine the impact of time on state-of-the-art news veracity classifiers. We show that, as time progresses, classification performance for both unreliable and hyper-partisan news classification slowly degrade. While this degradation does happen, it happens slower than expected, illustrating that hand-crafted, content-based features, such as style of writing, are fairly robust to changes in the news cycle. We show that this small degradation can be mitigated using online learning. Last, we examine the impact of adversarial content manipulation by malicious news producers. Specifically, we test three types of attack based on changes in the input space and data availability. We show that static models are susceptible to content manipulation attacks, but online models can recover from such attacks.
更多查看译文
关键词
Fake news,adversarial machine learning,biased news,concept drift,disinformation,fake news detection,misinformation,misleading news,robust machine learning
AI 理解论文
溯源树
样例
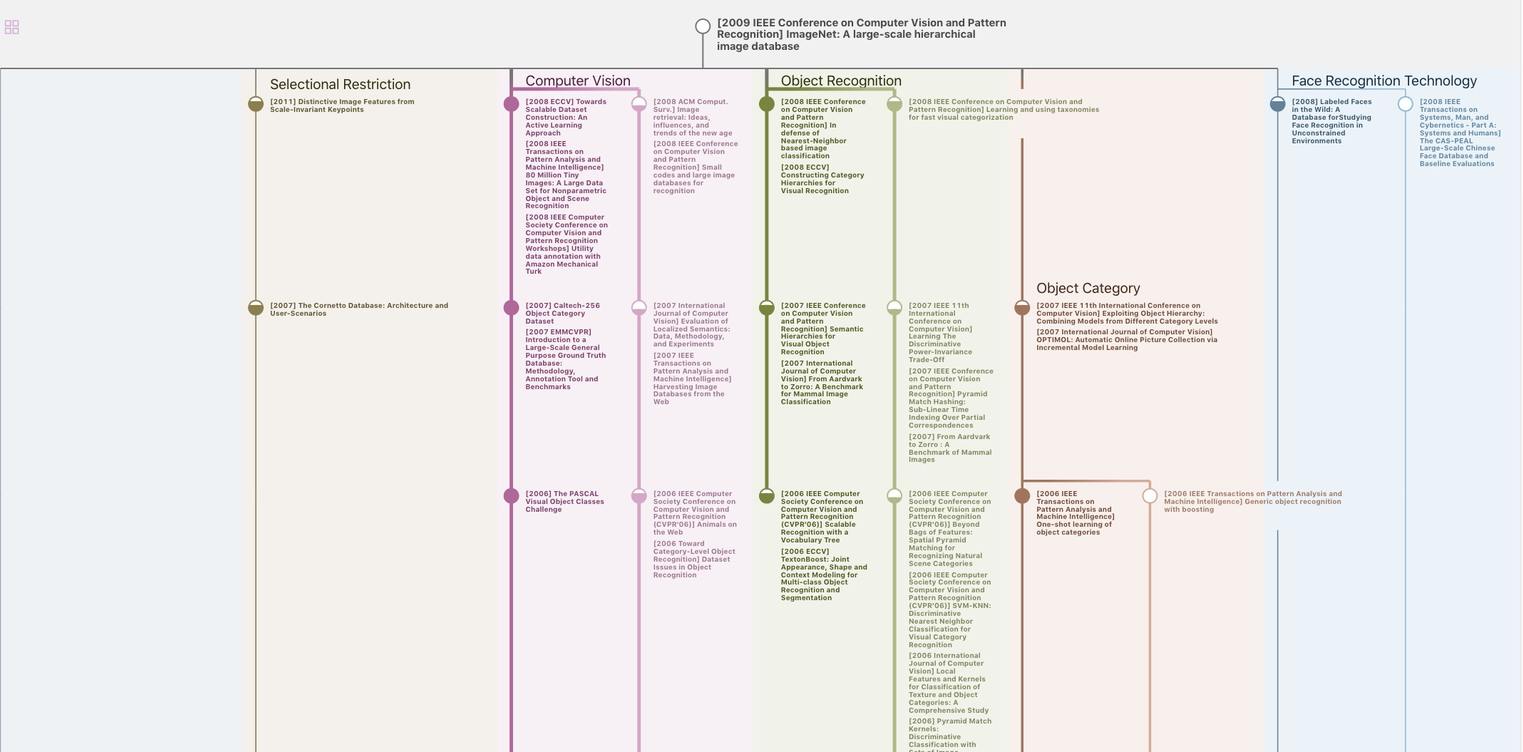
生成溯源树,研究论文发展脉络
Chat Paper
正在生成论文摘要