Flexible Multi-modal Hashing for Scalable Multimedia Retrieval
ACM Transactions on Intelligent Systems and Technology (TIST)(2020)
摘要
Multi-modal hashing methods could support efficient multimedia retrieval by combining multi-modal features for binary hash learning at the both offline training and online query stages. However, existing multi-modal methods cannot binarize the queries, when only one or part of modalities are provided. In this article, we propose a novel Flexible Multi-modal Hashing (FMH) method to address this problem. FMH learns multiple modality-specific hash codes and multi-modal collaborative hash codes simultaneously within a single model. The hash codes are flexibly generated according to the newly coming queries, which provide any one or combination of modality features. Besides, the hashing learning procedure is efficiently supervised by the pair-wise semantic matrix to enhance the discriminative capability. It could successfully avoid the challenging symmetric semantic matrix factorization and O(n2) storage cost of semantic matrix. Finally, we design a fast discrete optimization to learn hash codes directly with simple operations. Experiments validate the superiority of the proposed approach.
更多查看译文
关键词
Multi-modal hashing,efficient discrete optimization
AI 理解论文
溯源树
样例
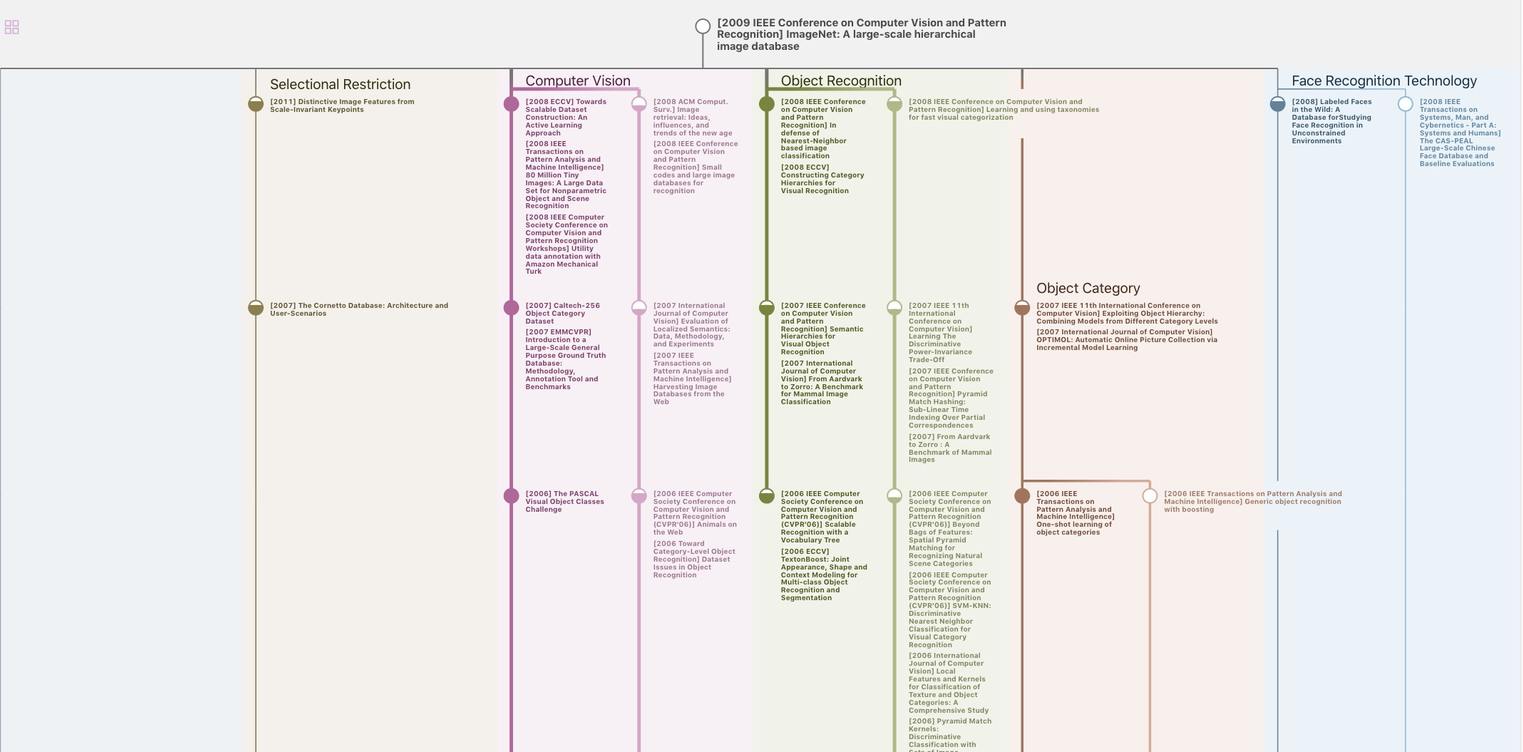
生成溯源树,研究论文发展脉络
Chat Paper
正在生成论文摘要