Multi-layer optimizations for end-to-end data analytics.
CGO'20: PROCEEDINGS OF THE18TH ACM/IEEE INTERNATIONAL SYMPOSIUM ON CODE GENERATION AND OPTIMIZATION(2020)
摘要
We consider the problem of training machine learning models over multi-relational data. The mainstream approach is to first construct the training dataset using a feature extraction query over input database and then use a statistical software package of choice to train the model. In this paper we introduce Iterative Functional Aggregate Queries (IFAQ), a framework that realizes an alternative approach. IFAQ treats the feature extraction query and the learning task as one program given in the IFAQ's domain-specific language, which captures a subset of Python commonly used in Jupyter notebooks for rapid prototyping of machine learning applications. The program is subject to several layers of IFAQ optimizations, such as algebraic transformations, loop transformations, schema specialization, data layout optimizations, and finally compilation into efficient low-level C++ code specialized for the given workload and data.
We show that a Scala implementation of IFAQ can outperform mlpack, Scikit, and TensorFlow by several orders of magnitude for linear regression and regression tree models over several relational datasets.
更多查看译文
关键词
In-Database Machine Learning, Multi-Query Optimization, Query Compilation
AI 理解论文
溯源树
样例
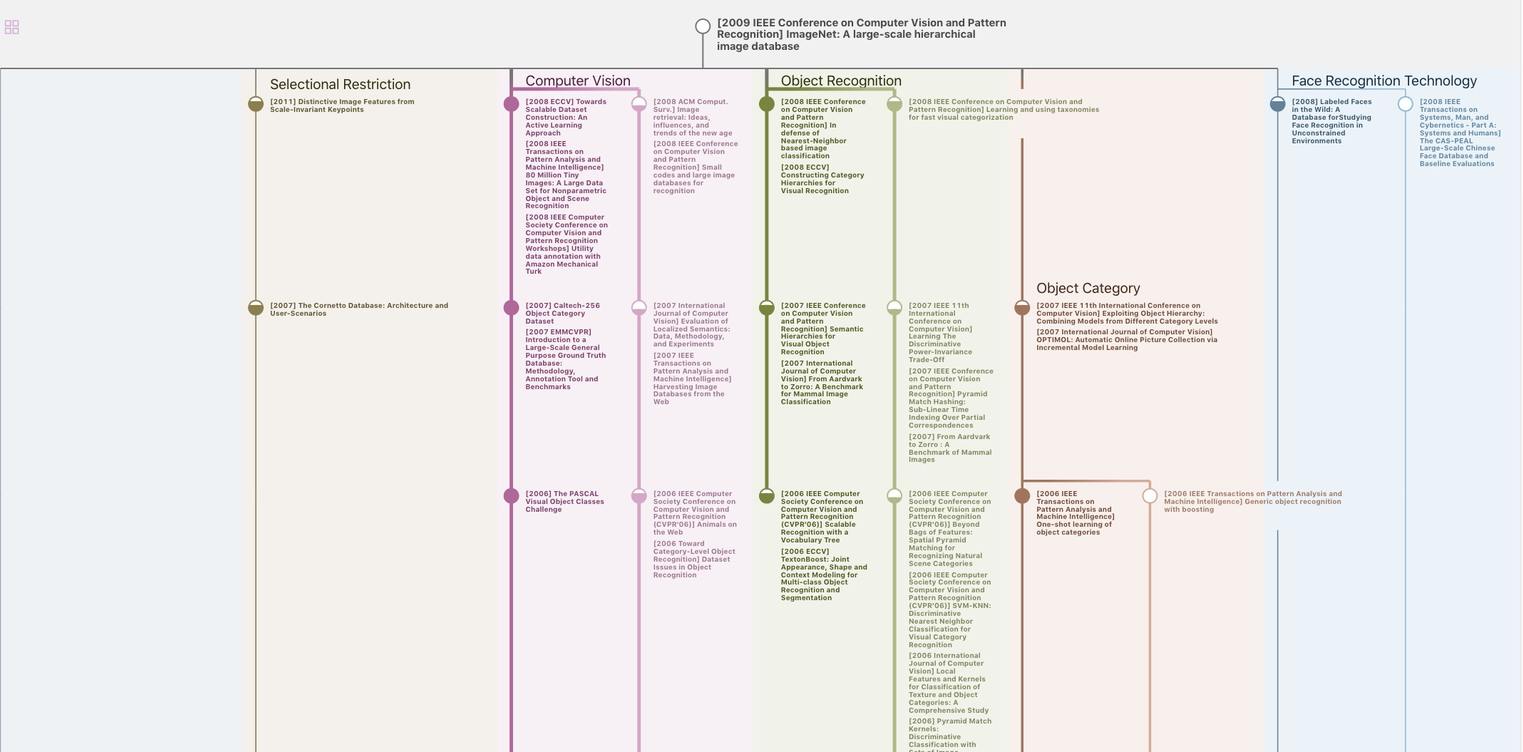
生成溯源树,研究论文发展脉络
Chat Paper
正在生成论文摘要