Restaurant Health Inspections And Crime Statistics Predict The Real Estate Market In New York City
MACHINE LEARNING, OPTIMIZATION, AND DATA SCIENCE(2019)
摘要
Predictions of apartments prices in New York City (NYC) have always been of interest to new homeowners, investors, Wall Street funds managers, and inhabitants of the city. In recent years, average prices have risen to the highest ever recorded rebounding after the 2008 economic recession. Although prices are trending up, not all apartments are. Different regions of the city have appreciated differently over time; knowing where to buy or sell is essential for all stakeholders. In this project, we propose a predictive analytics framework that analyzes new alternative data sources to extract predictive features of the NYC real estate market. Our experiments indicated that restaurant health inspection data and crime statistics can help predict apartments prices in NYC. The framework we introduce in this work uses an artificial recurrent neural network with Long Short-Term Memory (LSTM) units and incorporates the two latter predictive features to predict future prices of apartments. Empirical results show that feeding predictive features from (1) restaurant inspections data and (2) crime statistics to a neural network with LSTM units results in smaller errors than the traditional Autoregressive Integrated Moving Average (ARIMA) model, which is normally used for this type of regression. Predictive analytics based on non-linear models with features from alternative data sources can capture hidden relationships that linear models are not able not discover. The framework presented in this study has the potential to serve as a supplement to the traditional forecasting tools of real estate markets.
更多查看译文
关键词
Artificial Intelligence, Predictive analytics, Supervised learning, Recurrent neural networks, Open Data, Alternative data, Wall Street, Real estate markets
AI 理解论文
溯源树
样例
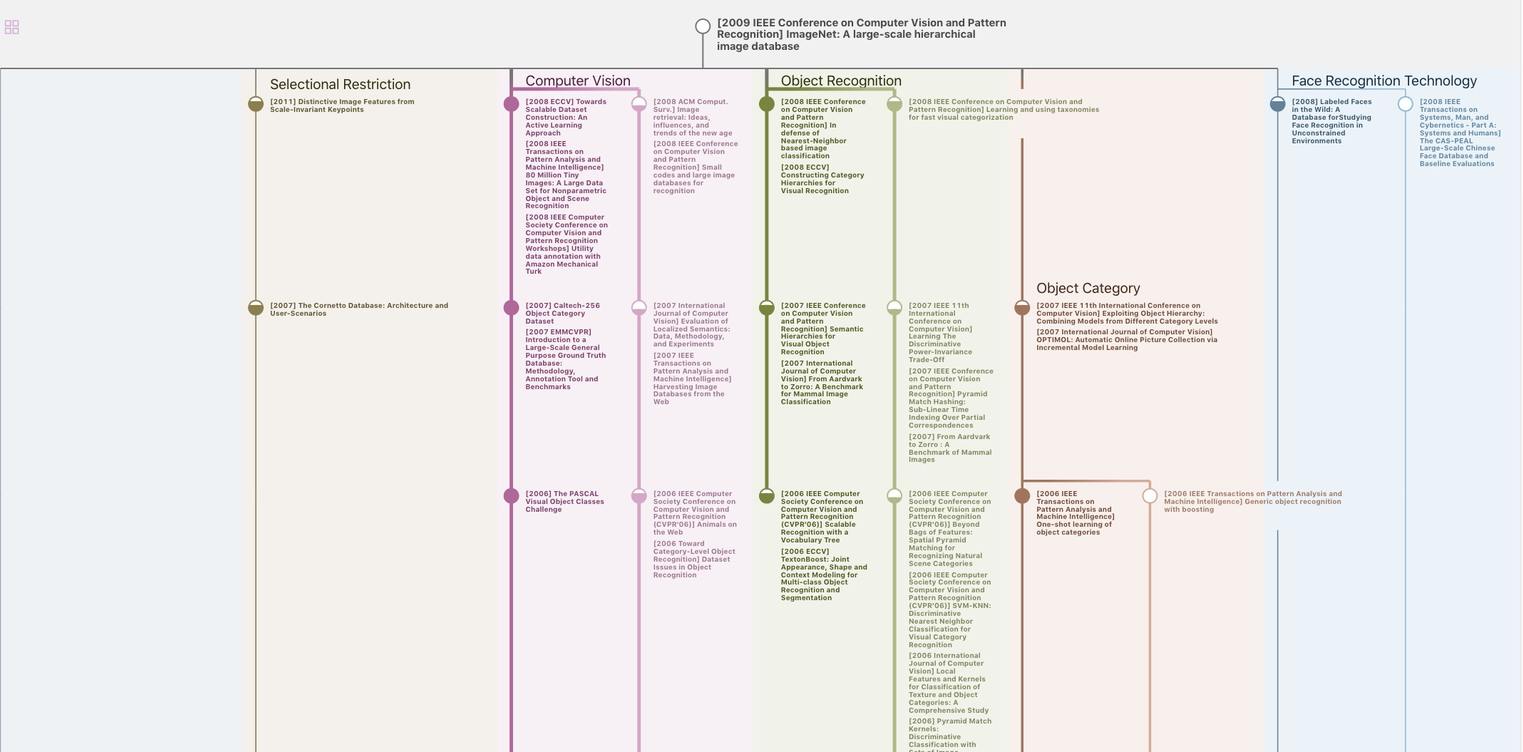
生成溯源树,研究论文发展脉络
Chat Paper
正在生成论文摘要