Analysing The Overfit Of The Auto-Sklearn Automated Machine Learning Tool
MACHINE LEARNING, OPTIMIZATION, AND DATA SCIENCE(2019)
摘要
With the ever-increasing number of pre-processing and classification algorithms, manually selecting the best algorithm and their best hyper-parameter settings (i.e. the best classification workflow) is a daunting task. Automated Machine Learning (Auto-ML) methods have been recently proposed to tackle this issue. Auto-ML tools aim to automatically choose the best classification workflow for a given dataset. In this work we analyse the predictive accuracy and overfit of the state-of-the-art auto-sklearn tool, which iteratively builds a classification ensemble optimised for the user's dataset. This work has 3 contributions. First, we measure 3 types of auto-sklearn's overfit, involving the differences of predictive accuracies measured on different data subsets: two parts of the training set (for learning and internal validation of the model) and the hold-out test set used for final evaluation. Second, we analyse the distribution of types of classification models selected by auto-sklearn across all 17 datasets. Third, we measure correlations between predictive accuracies on different data subsets and different types of overfitting. Overall, substantial degrees of overfitting were found in several datasets, and decision tree ensembles were the most frequently selected types of models.
更多查看译文
关键词
Automated Machine Learning, Overfit, Classification
AI 理解论文
溯源树
样例
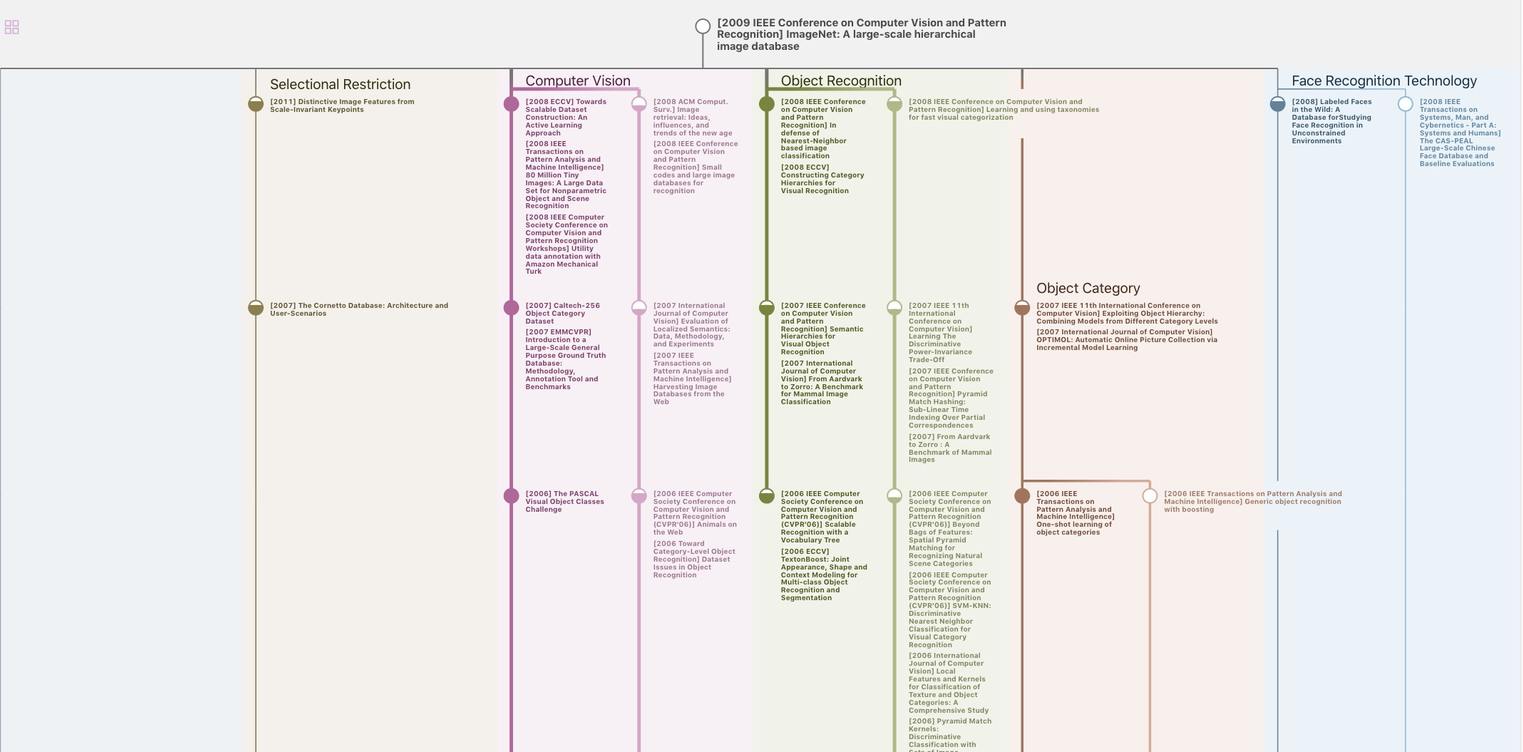
生成溯源树,研究论文发展脉络
Chat Paper
正在生成论文摘要