Performance Evaluation Of Local Surrogate Models In Bilevel Optimization
MACHINE LEARNING, OPTIMIZATION, AND DATA SCIENCE(2019)
摘要
Bilevel problems (BLPs) involve solving two nested levels of optimization, namely the upper (leader) and the lower (follower) level, usually motivated by real-world situations involving a hierarchical structure. BLPs are known to be hard and computationally expensive. When the computation of the objective functions and/or constraints require an expensive computer simulation, as in "black-box" optimization, evolutionary algorithms (EAs) are often used. As EAs may become very expensive by requiring a large number of function evaluations, surrogate models can help overcome this drawback, either by replacing expensive evaluations or allowing for increased exploration of the search space. Here we apply different types of local surrogate models at the upper level optimization, in order to enhance the overall performance of the proposed method, which is studied by means of computational experiments conducted on the well-known SMD benchmark problems.
更多查看译文
关键词
Bilevel optimization, Surrogate models, Differential evolution
AI 理解论文
溯源树
样例
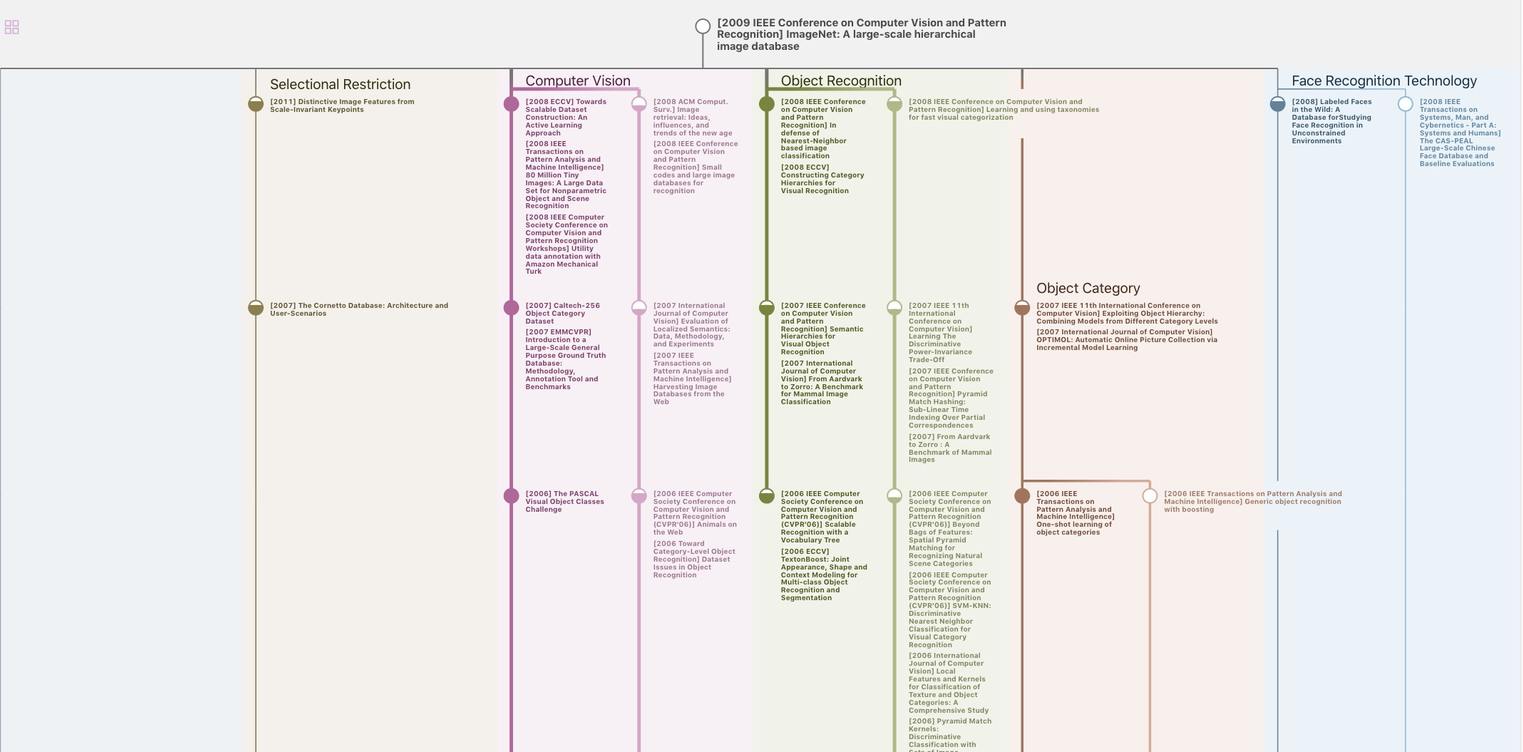
生成溯源树,研究论文发展脉络
Chat Paper
正在生成论文摘要