Polygenic risk scores outperform machine learning methods in predicting coronary artery disease status.
GENETIC EPIDEMIOLOGY(2020)
摘要
Coronary artery disease (CAD) is the leading global cause of mortality and has substantial heritability with a polygenic architecture. Recent approaches of risk prediction were based on polygenic risk scores (PRS) not taking possible nonlinear effects into account and restricted in that they focused on genetic loci associated with CAD, only. We benchmarked PRS, (penalized) logistic regression, naive Bayes (NB), random forests (RF), support vector machines (SVM), and gradient boosting (GB) on a data set of 7,736 CAD cases and 6,774 controls from Germany to identify the algorithms for most accurate classification of CAD status. The final models were tested on an independent data set from Germany (527 CAD cases and 473 controls). We found PRS to be the best algorithm, yielding an area under the receiver operating curve (AUC) of 0.92 (95% CI [0.90, 0.95], 50,633 loci) in the German test data. NB and SVM (AUC similar to 0.81) performed better than RF and GB (AUC similar to 0.75). We conclude that using PRS to predict CAD is superior to machine learning methods.
更多查看译文
关键词
classification,coronary artery disease,machine learning,polygenic risk scores,prediction
AI 理解论文
溯源树
样例
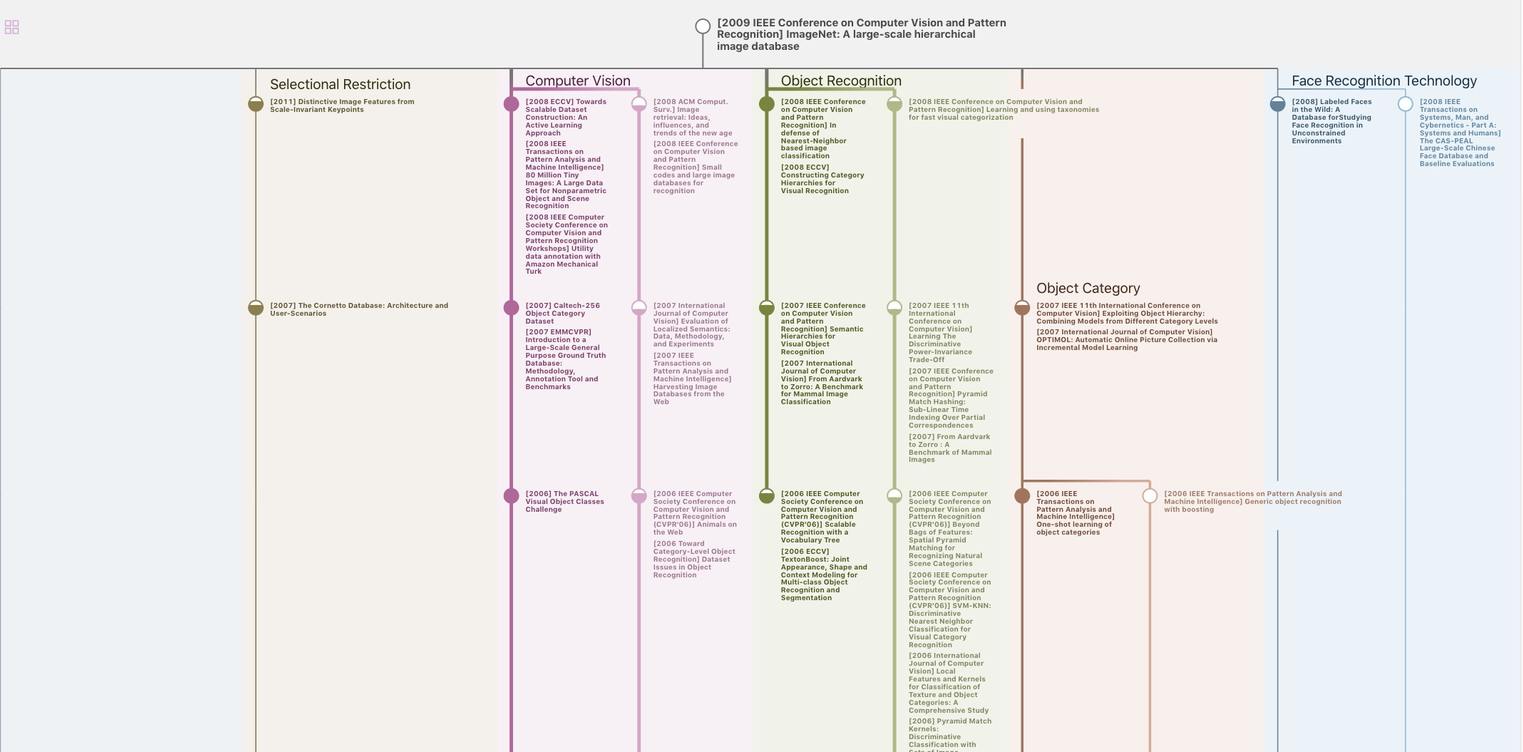
生成溯源树,研究论文发展脉络
Chat Paper
正在生成论文摘要