Assessment of variation in 30-day mortality following cancer surgeries among older adults across US hospitals.
CANCER MEDICINE(2020)
摘要
Background While public reporting of surgical outcomes for noncancer conditions is common, cancer surgeries have generally been excluded. This is true despite numerous studies showing outcomes to differ between hospitals based on their characteristics. Our objective was to assess whether three prerequisites for quality assessment and reporting are present for 30-day mortality after cancer surgery: low burden for timely reporting, hospital variation, and potential for public health gains. Study Design We used Fee-for-Service (FFS) Medicare claims to examine the extent of variation in 30-day cancer surgical mortality between 3860 US hospitals. We included 340 489 surgeries for 12 cancer types for FFS Medicare beneficiaries aged >= 66 years, 2011-2013. Hierarchical mixed-effects logistic regression models adjusted for patient and hospital characteristics and with a random hospital effect were fit to obtain hospital-specific risk-standardized mortality rates (RSMRs) and 99% confidence intervals (CI). We calculated a hospital odds ratio to describe the difference in mortality risk for a hospital above vs below average quality and estimated the potential mortality reduction. Results The median number of cancer surgeries per hospital was 34. The median RSMR overall was 2.41% (99% CI 2.28%, 2.66%). In aggregate and for most cancers, variation between hospitals exceeded that due to differences in patient and hospital characteristics. For individual cancers, relative differences exceeded 20% in mortality risk between patients undergoing surgery at a hospital below vs above average quality, with the potential for an estimated 500 deaths prevented annually given hypothetical improvements. Conclusion Quality measurement and reporting of 30-day mortality for cancer surgery is worthy of consideration.
更多查看译文
关键词
complications,health care,outcomes assessment (health care),quality indicators,quality of health care,surgery
AI 理解论文
溯源树
样例
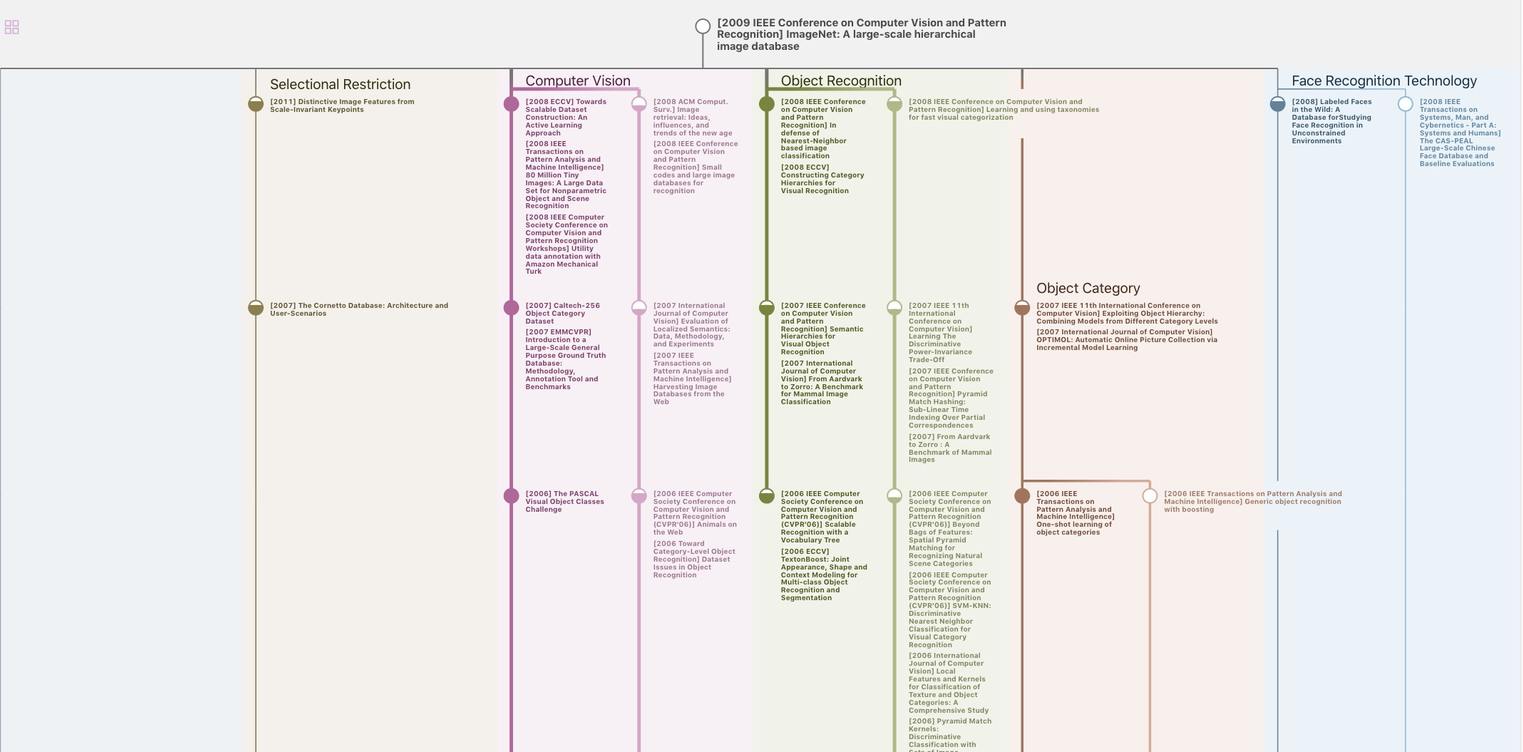
生成溯源树,研究论文发展脉络
Chat Paper
正在生成论文摘要