Data Normalization in Structural Health Monitoring by Means of Nonlinear Filtering
2019 8th Brazilian Conference on Intelligent Systems (BRACIS)(2019)
摘要
Structural Health Monitoring (SHM) is a skillful strategy for preserving different kinds of structures in short-and long-term. This work describes the effects of environmental variability and operational conditions (e.g., temperature, relative humidity, wind speed and operational load) on real structures, as well as measures efficiency in data normalization by applying state-of-the-art algorithms that carry out nonlinear principal component analysis (PCA), which are Autoassociative Neural Network (AANN), Kernel PCA (KPCA) and Greedy KPCA (GKPCA), in contrast to the respective linear version, the PCA algorithm. The results allow us to conclude that KPCA and GKPCA are the most efficient algorithms in the tasks of learning structure normal conditions and classification against the other techniques in terms of false positive and negative indications of damage, suggesting applicability in real monitoring scenarios.
更多查看译文
关键词
Data Normalization, Damage Detection, Nonlinear Principal Component Analysis, Structural Health Monitoring, Machine Learning
AI 理解论文
溯源树
样例
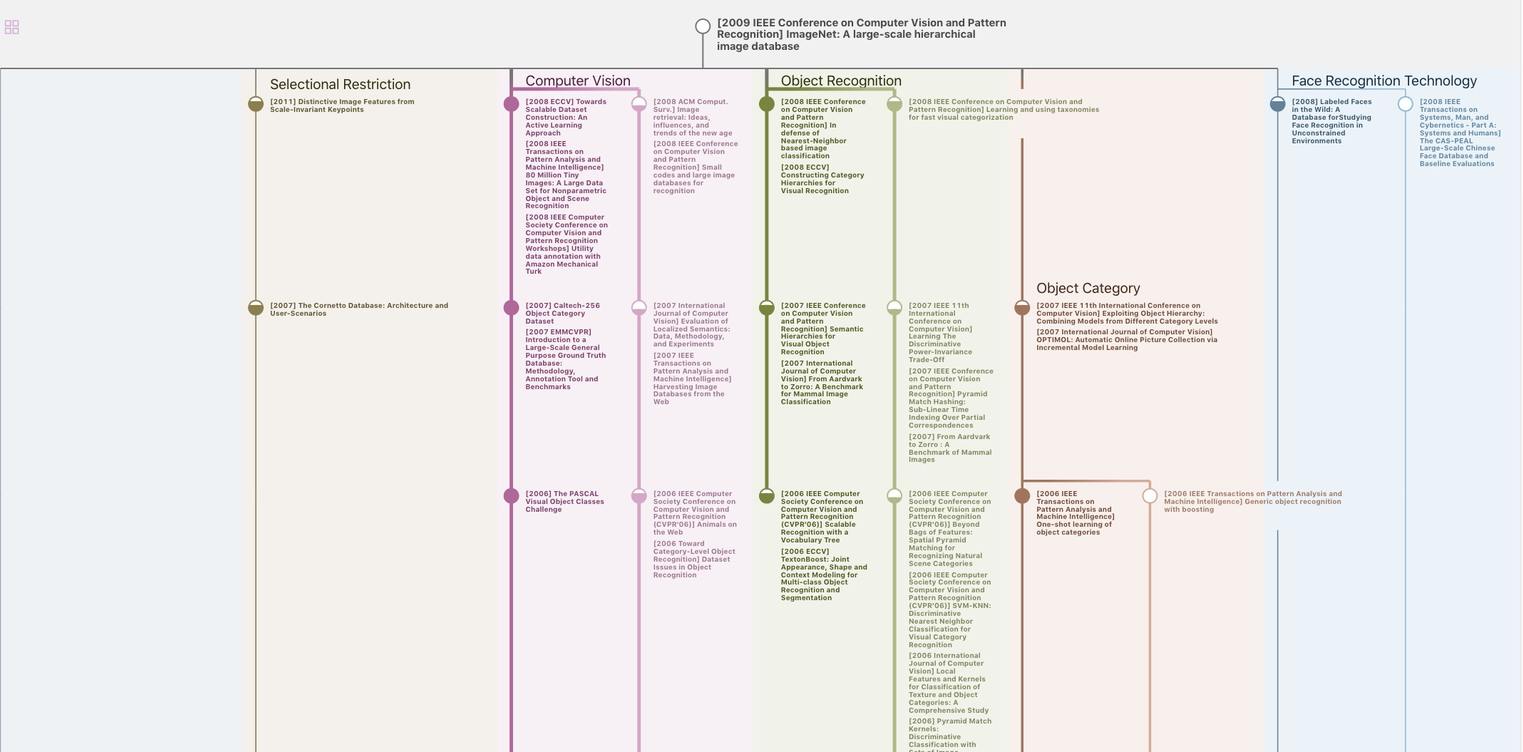
生成溯源树,研究论文发展脉络
Chat Paper
正在生成论文摘要