Network Data Flow Clustering based on Unsupervised Learning
2019 IEEE 18th International Symposium on Network Computing and Applications (NCA)(2019)
摘要
Network communication data analysis is crucial in order to provide an adequate security level in computer infrastructures. As the volume of data and the number of features rise, the difficulties associated with its study also increase. A possible approach to this problem based on Artificial Intelligence techniques is to perform clustering, creating groups of similar characteristics. Self-Organized Maps are an artificial neural networks which perform dimensionality reduction as well as clustering over numerical data. In this paper, we propose a mixed numerical-categorical version of Self-Organized Maps algorithm applied to network communication data. Moreover a study of its enhancement with the inclusion of time dependent features is performed. The technique is tested with a well known dataset for Intrusion Detection Systems and it provides a technique to create different clusters or groups of different types of traffic by means of network information.
更多查看译文
关键词
Self-Organizing Maps,IDS,Network Security,Categorical SOM,Visualization,Unsupervised Clustering
AI 理解论文
溯源树
样例
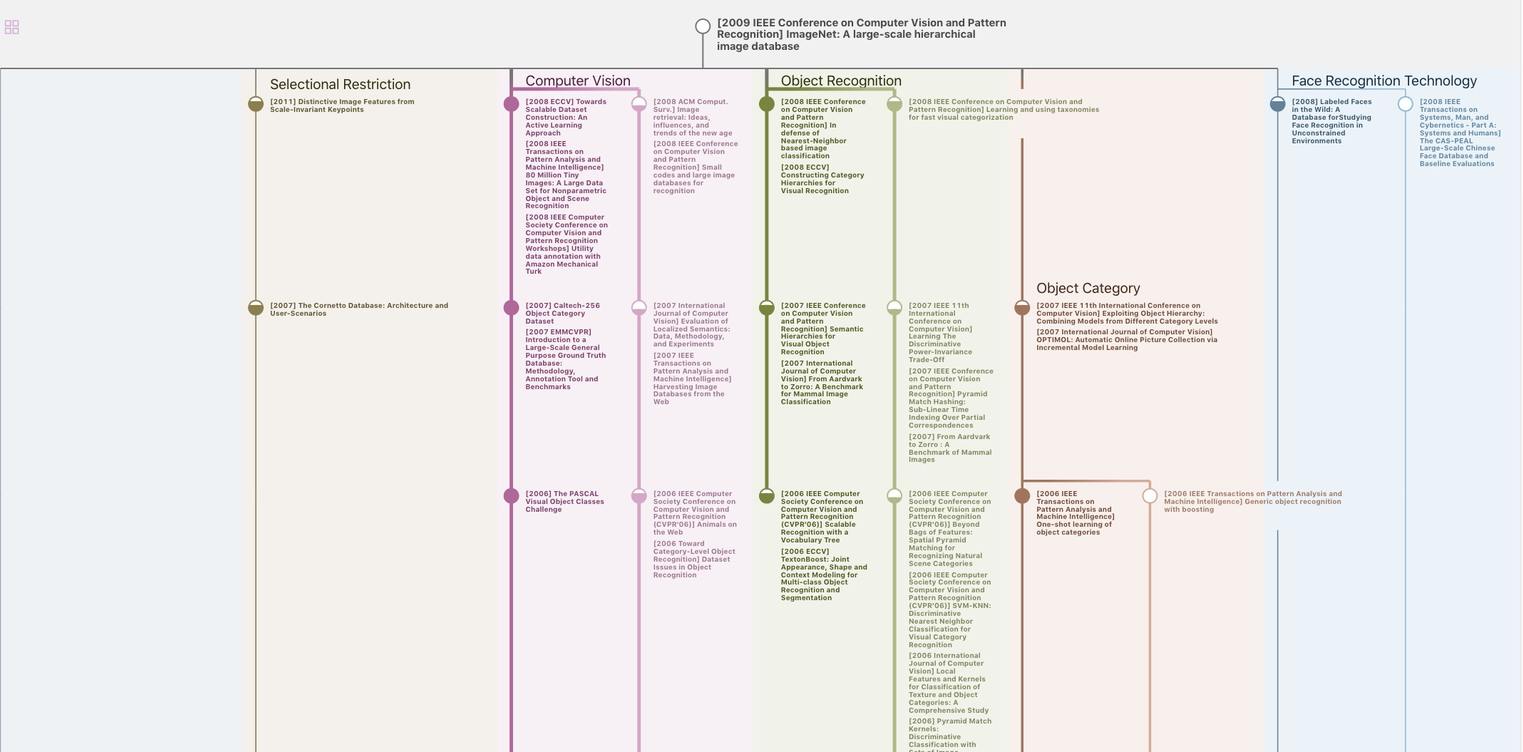
生成溯源树,研究论文发展脉络
Chat Paper
正在生成论文摘要