Adapting ClusTree for more challenging data stream environments.
JOURNAL OF INTELLIGENT & FUZZY SYSTEMS(2019)
摘要
Data stream mining seeks to extract useful information from quickly-arriving, infinitely-sized and evolving data streams. Although these challenges have been addressed throughout the literature, none of them can be considered "solved." We contribute to closing this gap for the task of data stream clustering by proposing two modifications to the well-known ClusTree data stream clustering algorithm: pruning unused branches and detecting concept drift. Our experimental results show the difficulty in tackling these aspects of data stream mining and the sensitivity of stream mining algorithms to parameter values. We conclude that further research is required to better equip stream learners for the data stream clustering task.
更多查看译文
关键词
Concept drift,data streams,ClusTree,on-line learning
AI 理解论文
溯源树
样例
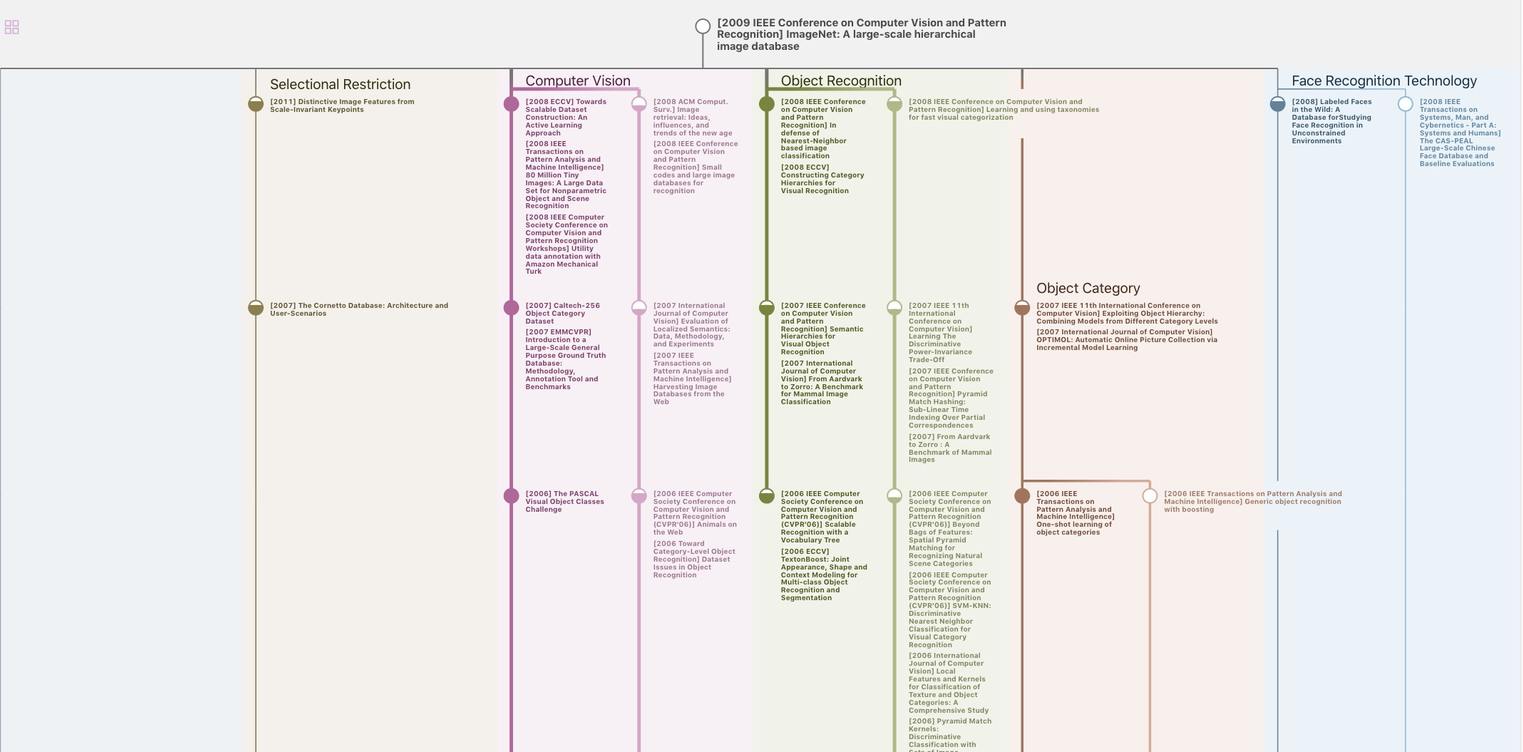
生成溯源树,研究论文发展脉络
Chat Paper
正在生成论文摘要