Unsupervised Video Action Clustering via Motion-Scene Interaction Constraint
IEEE Transactions on Circuits and Systems for Video Technology(2020)
摘要
In the past few years, scene contextual information has been increasingly used for action understanding with promising results. However, unsupervised video action clustering using context has been less explored, and existing clustering methods cannot achieve satisfactory performances. In this paper, we propose a novel unsupervised video action clustering method by using the motion-scene interaction constraint (MSIC). The proposed method takes the unique static scene and dynamic motion characteristics of video action into account, and develops a contextual interaction constraint model under a self-representation subspace clustering framework. First, the complementarity of multi-view subspace representation in each context is explored by single-view and multi-view constraints. Afterward, the context-constrained affinity matrix is calculated and the MSIC is introduced to mutually regularize the disagreement of subspace representation in scene and motion. Finally, by jointly constraining the complementarity of multi-views and the consistency of multi-contexts, an overall objective function is constructed to guarantee the video action clustering result. The experiments on four video benchmark datasets (Weizmann, KTH, UCFsports, and Olympic) demonstrate that the proposed method outperforms the state-of-the-art methods.
更多查看译文
关键词
Task analysis,Clustering methods,Dynamics,Trajectory,Manifolds,Context modeling,Linear programming
AI 理解论文
溯源树
样例
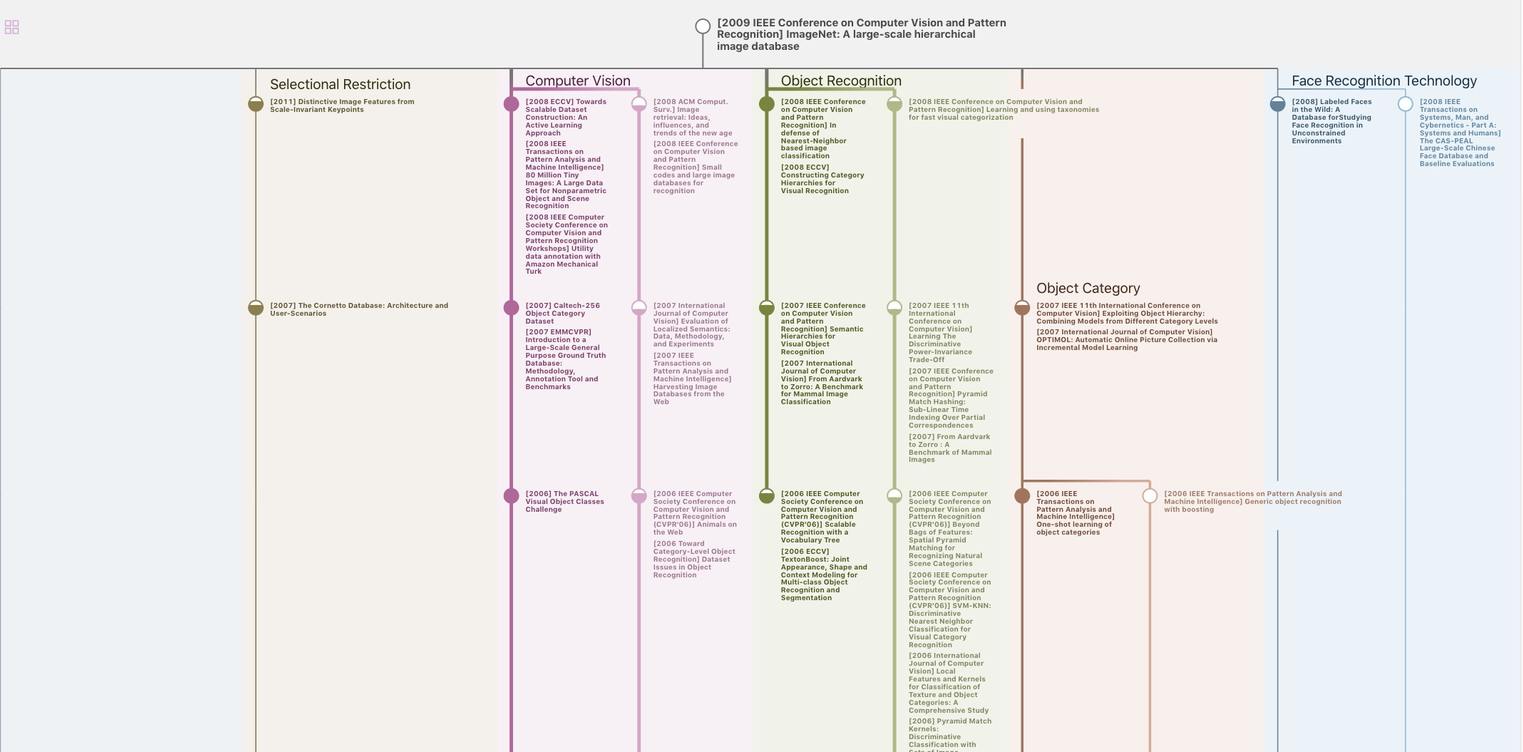
生成溯源树,研究论文发展脉络
Chat Paper
正在生成论文摘要