Few-Shot Learning With Geometric Constraints
IEEE Transactions on Neural Networks and Learning Systems(2020)
摘要
In this article, we consider the problem of few-shot learning for classification. We assume a network trained for base categories with a large number of training examples, and we aim to add novel categories to it that have only a few, e.g., one or five, training examples. This is a challenging scenario because: 1) high performance is required in both the base and novel categories; and 2) training the network for the new categories with a few training examples can contaminate the feature space trained well for the base categories. To address these challenges, we propose two geometric constraints to fine-tune the network with a few training examples. The first constraint enables features of the novel categories to cluster near the category weights, and the second maintains the weights of the novel categories far from the weights of the base categories. By applying the proposed constraints, we extract discriminative features for the novel categories while preserving the feature space learned for the base categories. Using public data sets for few-shot learning that are subsets of ImageNet, we demonstrate that the proposed method outperforms prevalent methods by a large margin.
更多查看译文
关键词
Deep learning,few-shot learning,geometric constraint,image recognition,neural network
AI 理解论文
溯源树
样例
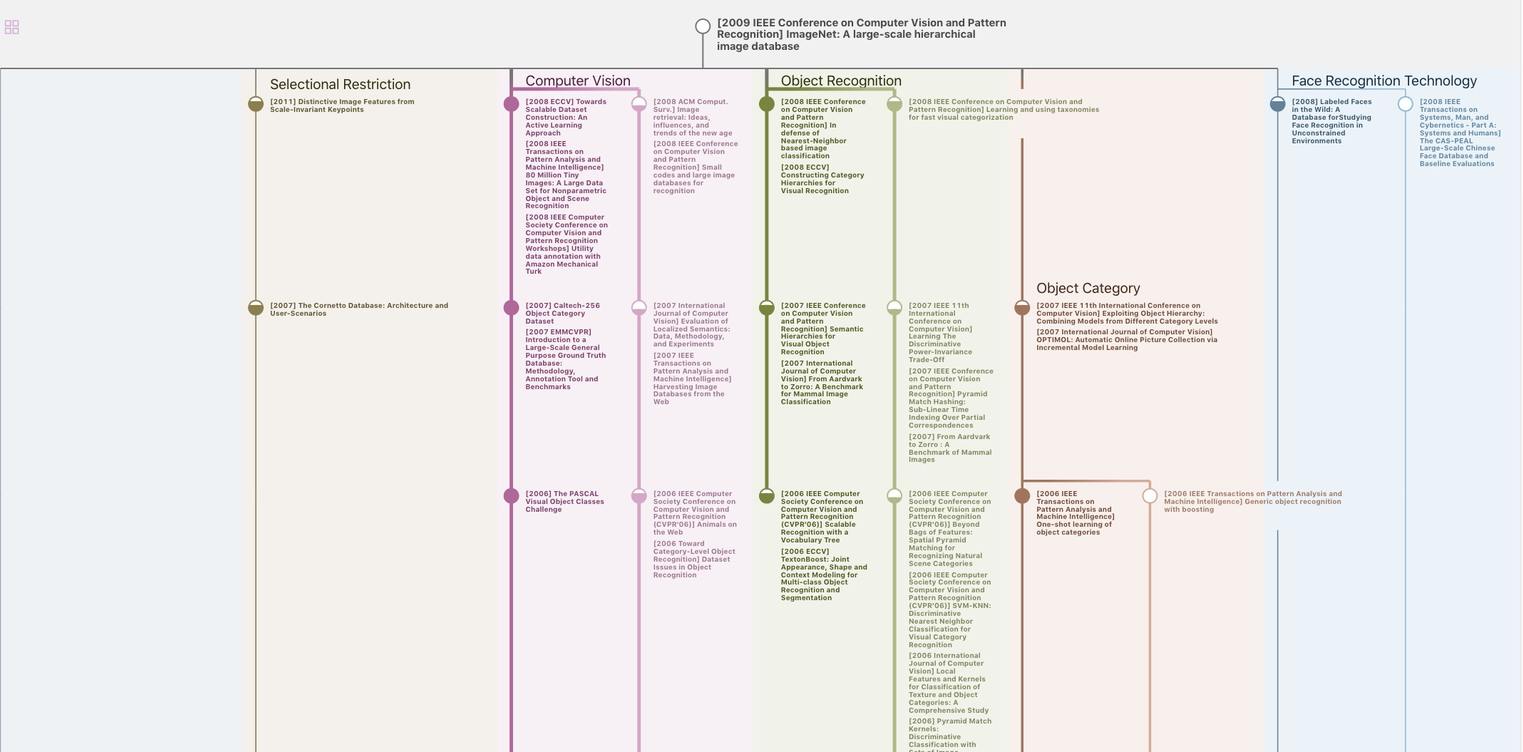
生成溯源树,研究论文发展脉络
Chat Paper
正在生成论文摘要