DeepAuto: A Hierarchical Deep Learning Framework for Real-Time Prediction in Cellular Networks
arxiv(2019)
摘要
Accurate real-time forecasting of key performance indicators (KPIs) is an essential requirement for various LTE/5G radio access network (RAN) automation. However, an accurate prediction can be very challenging in large-scale cellular environments due to complex spatio-temporal dynamics, network configuration changes and unavailability of real-time network data. In this work, we introduce a reusable analytics framework that enables real-time KPI prediction using a hierarchical deep learning architecture. Our prediction approach, namely DeepAuto, stacks multiple long short-term memory (LSTM) networks horizontally to capture instantaneous, periodic and seasonal patterns in KPI time-series. It further merge with feed-forward networks to learn the impact of network configurations and other external factors. We validate the approach by predicting two important KPIs, including cell load and radio channel quality, using large-scale real network streaming measurement data from the operator. For cell load prediction, DeepAuto model showed up to 15% improvement in Root Mean Square Error (RMSE) compared to naive method of using recent measurements for short-term horizon and up to 32% improvement for longer-term prediction.
更多查看译文
关键词
cellular networks,hierarchical deepauto learning framework,deepauto learning,prediction,real-time
AI 理解论文
溯源树
样例
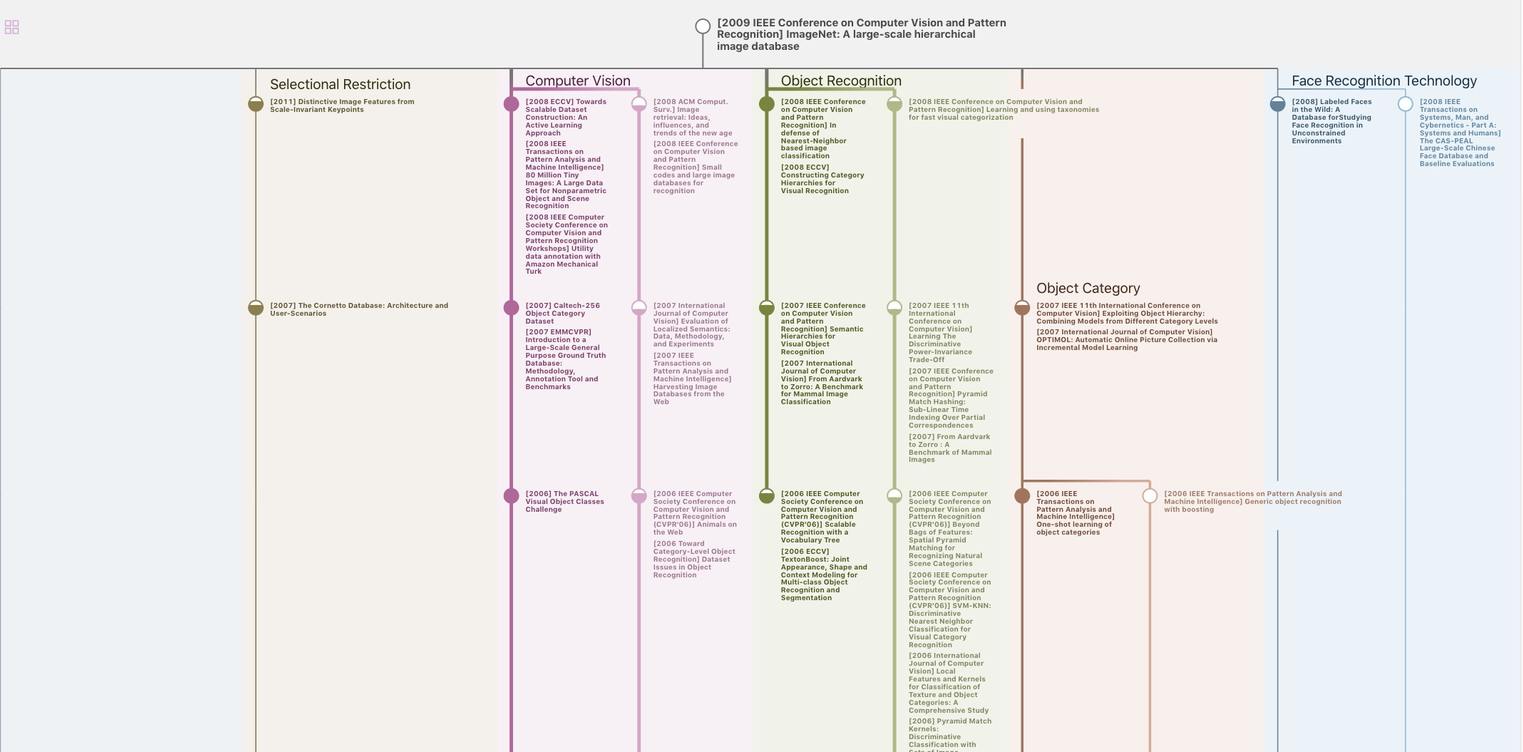
生成溯源树,研究论文发展脉络
Chat Paper
正在生成论文摘要