Static Allocation Of Parallel Tasks To Improve Schedulability In Cpu-Gpu Heterogeneous Real-Time Systems
45TH ANNUAL CONFERENCE OF THE IEEE INDUSTRIAL ELECTRONICS SOCIETY (IECON 2019)(2019)
摘要
Autonomous driving is one of the main challenges of modern cars. Computer visions and intelligent on-board decision making are crucial in autonomous driving and require heterogeneous processors with high computing capability under low power consumption constraints. The progress of parallel computing using heterogeneous processing units is further supported by software frameworks like OpenCL, OpenMP, CUDA, and C++AMP. These frameworks allow the allocation of parallel computation on different compute resources. This, however, creates a difficulty in allocating the right computation segments to the right processing units in such a way that the complete system meets all its timing requirements. In this paper, we consider pre-runtime static allocations of parallel tasks to perform their execution either sequentially on CPU or in parallel using a GPU. This allows for improving any unbalanced use of GPU accelerators in a heterogeneous environment. By performing several heuristic algorithms, we show that the overuse of accelerators results in a bottle-neck of the entire system execution. The experimental results show that our allocation schemes that target a balanced use of GPU improves the system schedulability up to 90%.
更多查看译文
关键词
static allocation,CPU-GPU heterogeneous real-time systems,autonomous driving,computer visions,low power consumption constraints,parallel computing,heterogeneous processing units,software frameworks,compute resources,pre-runtime static allocations,system schedulability,intelligent onboard decision making,heuristic algorithms
AI 理解论文
溯源树
样例
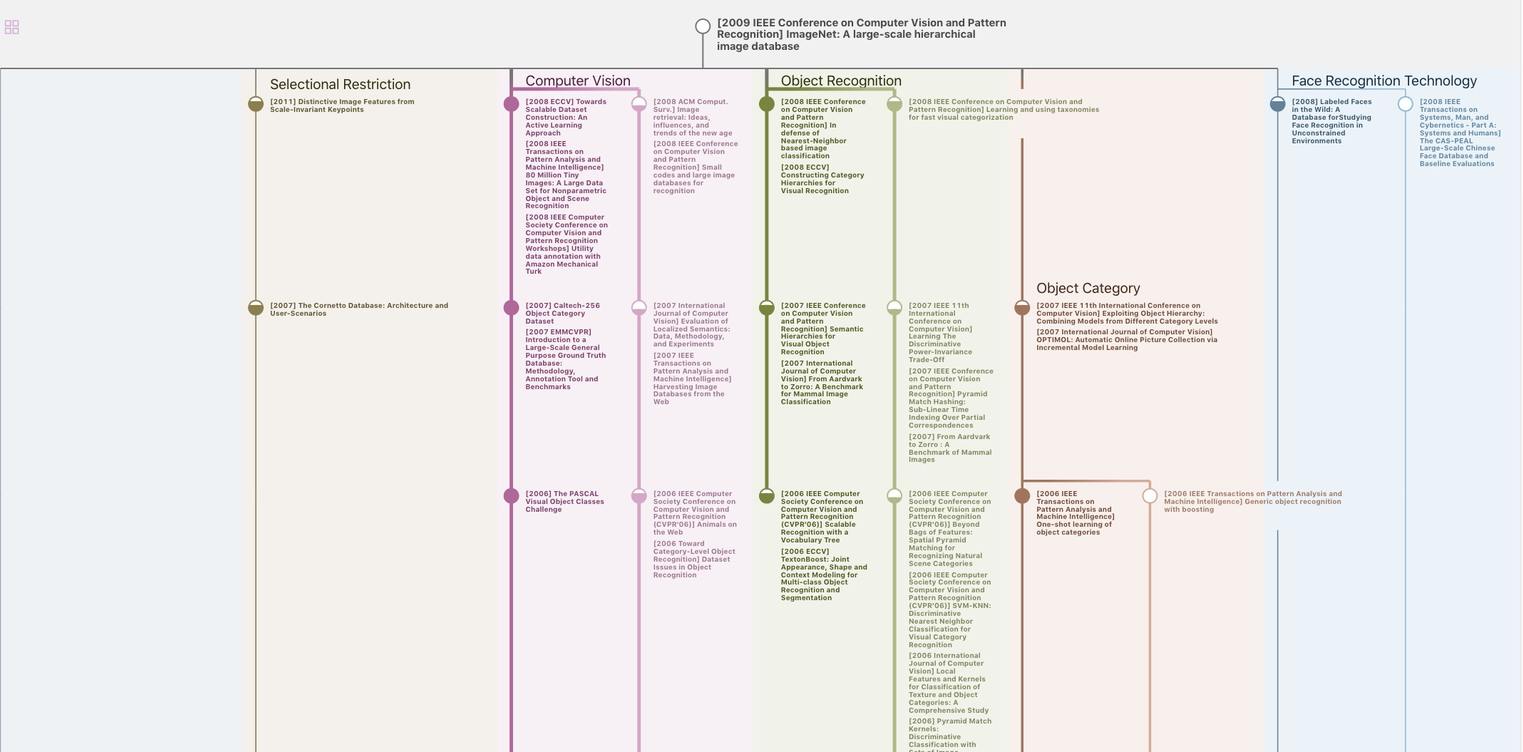
生成溯源树,研究论文发展脉络
Chat Paper
正在生成论文摘要