Application of Different Machine Learning Strategies for Current- and Vibration-based Motor Bearing Fault Detection in Induction Motors
IECON 2019 - 45th Annual Conference of the IEEE Industrial Electronics Society(2019)
摘要
In this paper, the application of different machine learning strategies for current- and vibration-based detection of bearing faults in squirrel-cage induction motors is studied. This study compares several feature extraction strategies such as a statistical and spectral analysis of vibration, a statistical analysis of the Hilbert's Transform envelope of vibration, an analysis of the currents deviation to a perfect sinusoid and a statistical and spectral analysis of the Park's Vector Modulus, with its performances being evaluated with the Support Vector Machine, Artificial Neural Network, Random Forests and Extreme Gradient Boosting algorithms. A comparison of results obtained using sampling frequencies of 0.8 kHz, 1 kHz, 2 kHz, 5 kHz and 10 kHz and analysis periods between 20 ms and 100 ms is made and promising models are achieved even with the lowest sampling frequencies.
更多查看译文
关键词
induction motor,bearing fault detection,vibration analysis,current analysis,machine learning,predictive maintenance,condition monitoring
AI 理解论文
溯源树
样例
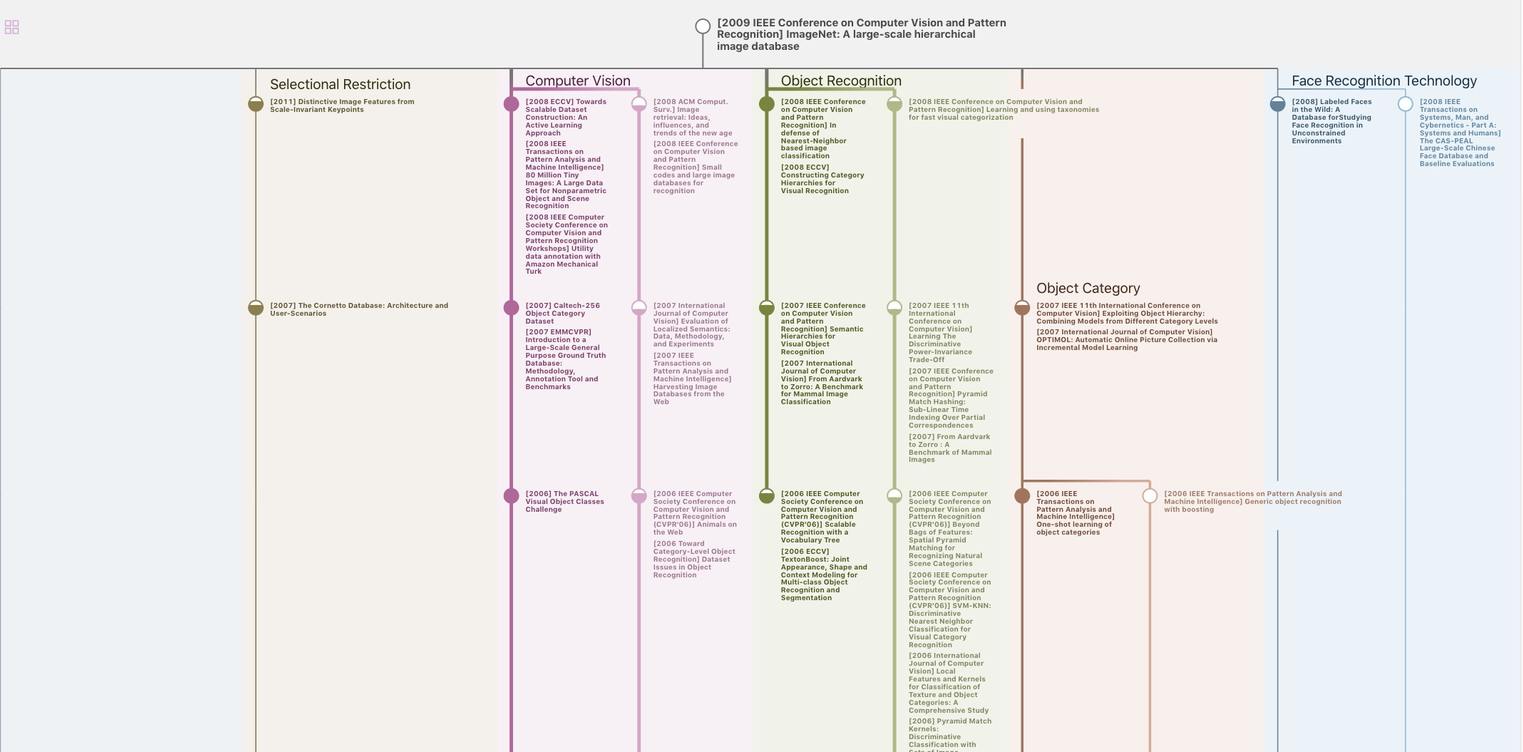
生成溯源树,研究论文发展脉络
Chat Paper
正在生成论文摘要