Reconstruction of multicontrast MR images through deep learning
MEDICAL PHYSICS(2020)
摘要
Purpose Magnetic resonance (MR) imaging with a long scan time can lead to degraded images due to patient motion, patient discomfort, and increased costs. For these reasons, the role of rapid MR imaging is important. In this study, we propose the joint reconstruction of multicontrast brain MR images from down-sampled data to accelerate the data acquisition process using a novel deep-learning network. Methods Twenty-one healthy volunteers (female/male = 7/14, age = 26 +/- 4 yr, range 22-35 yr) and 16 postoperative patients (female/male = 7/9, age = 49 +/- 9 yr, range 37-62 yr) were scanned on a 3T whole-body scanner for prospective and retrospective studies, respectively, using both T1-weighted spin-echo (SE) and T2-weighted fast spin-echo (FSE) sequences. We proposed a network which we term "X-net" to reconstruct both T1- and T2-weighted images from down-sampled images as well as a network termed "Y-net" which reconstructs T2-weighted images from highly down-sampled T2-weighted images and fully sampled T1-weighted images. Both X-net and Y-net are composed of two concatenated subnetworks. We investigate optimal sampling patterns, the optimal patch size for augmentation, and the optimal acceleration factors for network training. An additional Y-net combined with a generative adversarial network (GAN) was also implemented and tested to investigate the effects of the GAN on the Y-net performance. Single- and joint-reconstruction parallel-imaging and compressed-sensing algorithms along with a conventional U-net were also tested and compared with the proposed networks. For this comparison, the structural similarity (SSIM), normalized mean square error (NMSE), and Frechet inception distance (FID) were calculated between the outputs of the networks and fully sampled images. The statistical significance of the performance was evaluated by assessing the interclass correlation and in paired t-tests. Results The outputs from the two concatenated subnetworks were closer to the fully sampled images compared to those from one subnetwork, with this result showing statistical significance. Uniform down-sampling led to a statically significant improvement in the image quality compared to random or central down-sampling patterns. In addition, the proposed networks provided higher SSIM and NMSE values than U-net, compressed-sensing, and parallel-imaging algorithms, all at statistically significant levels. The GAN-based Y-net showed a better FID and more realistic images compared to a non-GAN-based Y-net. The performance capabilities of the networks were similar between normal subjects and patients. Conclusions The proposed X-net and Y-net effectively reconstructed full images from down-sampled images, outperforming the conventional parallel-imaging, compressed-sensing and U-net methods and providing more realistic images in combination with a GAN. The developed networks potentially enable us to accelerate multicontrast anatomical MR imaging in routine clinical studies including T1-and T2-weighted imaging.
更多查看译文
关键词
deep learning,multicontrast MRI,x-net,y-net
AI 理解论文
溯源树
样例
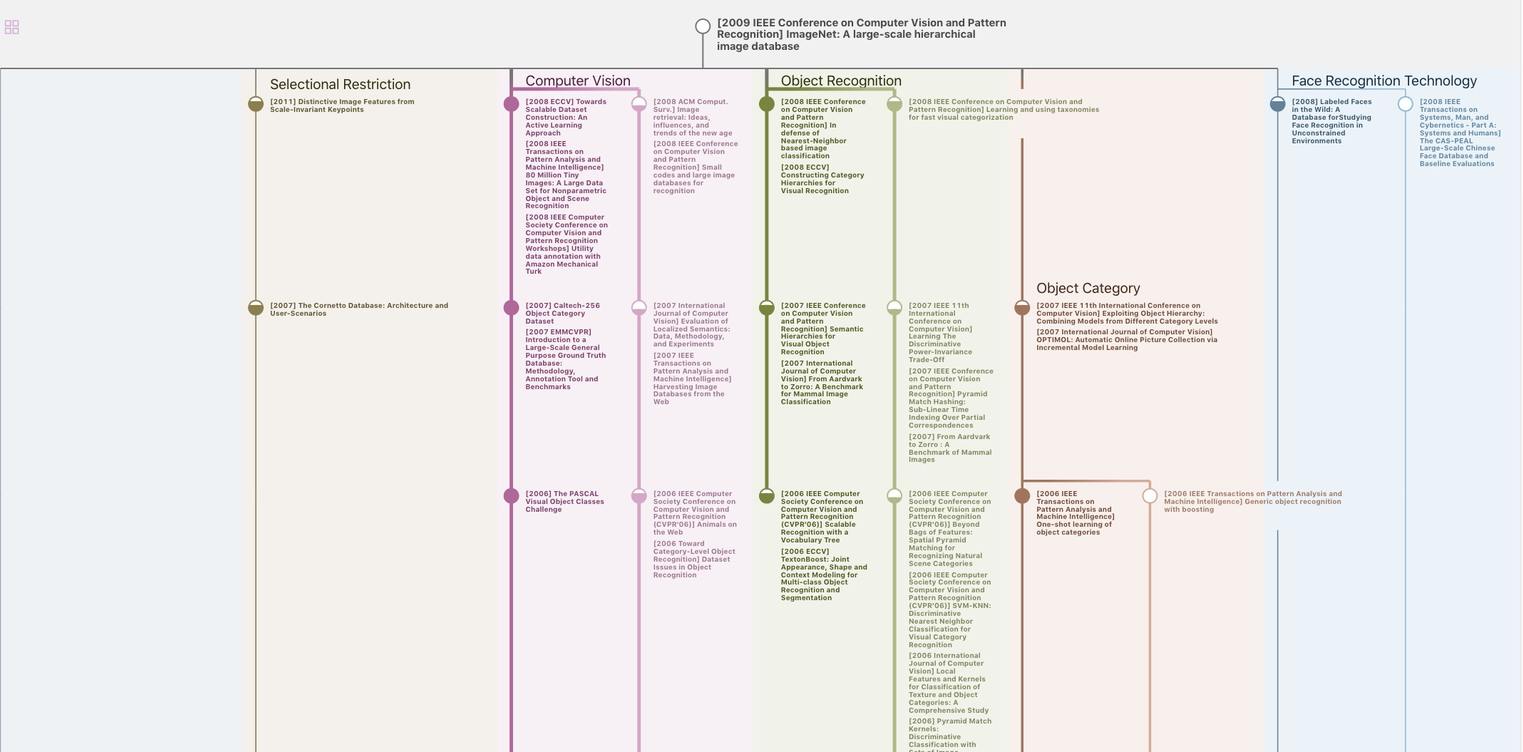
生成溯源树,研究论文发展脉络
Chat Paper
正在生成论文摘要