Parallel Cross-Validation: A Scalable Fitting Method For Gaussian Process Models
COMPUTATIONAL STATISTICS & DATA ANALYSIS(2021)
摘要
Gaussian process (GP) models are widely used to analyze spatially referenced data and to predict values at locations without observations. They are based on a statistical framework, which enables uncertainty quantification of the model structure and predictions. Both the evaluation of the likelihood and the prediction involve solving linear systems. Hence, the computational costs are large and limit the amount of data that can be handled. While there are many approximation strategies that lower the computational cost of GP models, they often provide sub-optimal support for the parallel computing capabilities of (high-performance) computing environments. To bridge this gap a parallelizable parameter estimation and prediction method is presented. The key idea is to divide the spatial domain into overlapping subsets and to use cross-validation (CV) to estimate the covariance parameters in parallel. Although simulations show that CV is less effective for parameter estimation than the maximum likelihood method, it is amenable to parallel computing and enables the handling of large datasets. Exploiting the screen effect for spatial prediction helps to arrive at a spatial analysis that is close to a global computation despite performing parallel computations on local regions. Simulation studies assess the accuracy of the parameter estimates and predictions. The implementation shows good weak and strong parallel scaling properties. For illustration, an exponential covariance model is fitted to a scientifically relevant canopy height dataset with 5 million observations. Using 512 processor cores in parallel brings the evaluation time of one covariance parameter configuration to 1.5 minutes. (C) 2020 Elsevier B.V. All rights reserved.
更多查看译文
关键词
Cross-validation, Gaussian random fields, High-performance computing, Kriging, Spatial statistics
AI 理解论文
溯源树
样例
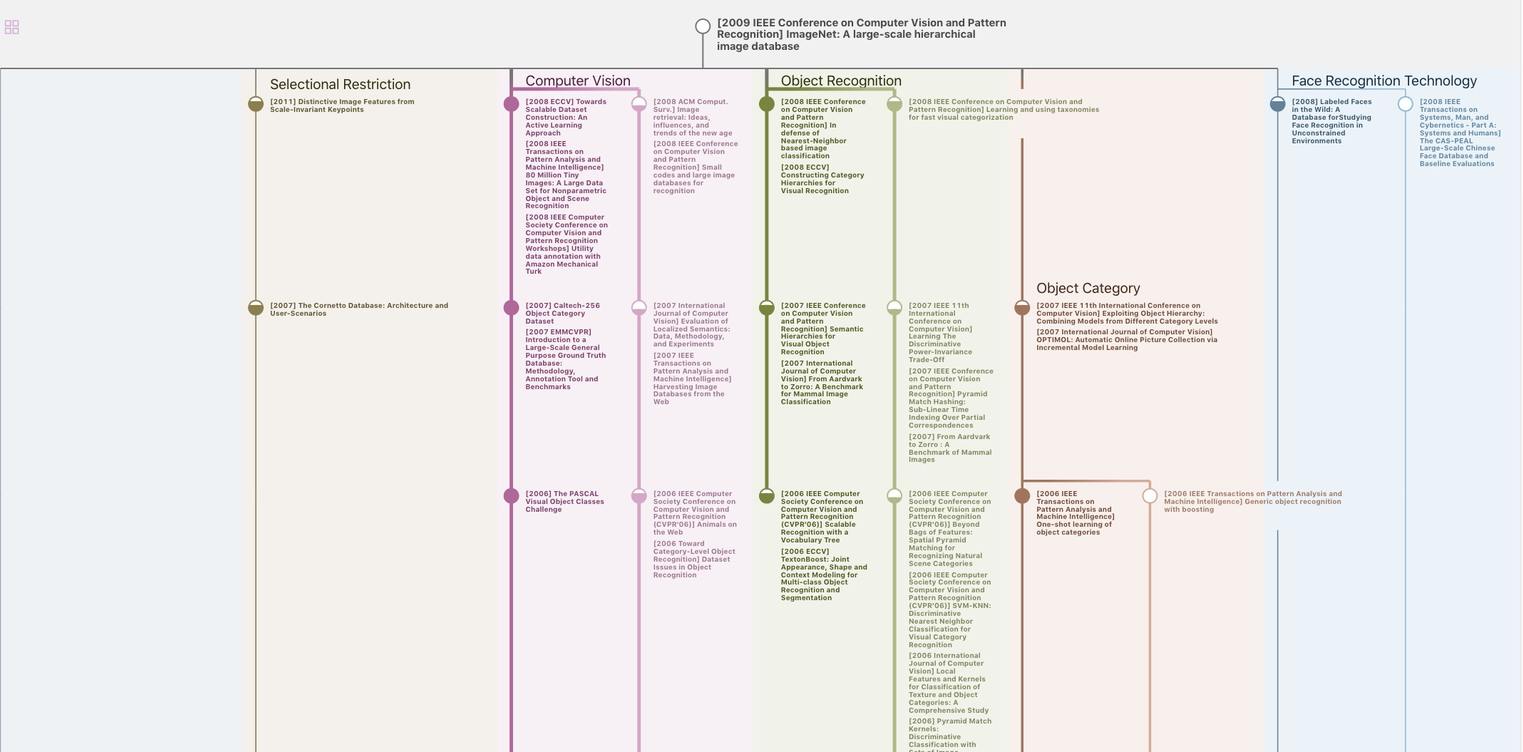
生成溯源树,研究论文发展脉络
Chat Paper
正在生成论文摘要