A Single Model CNN for Hyperspectral Image Denoising
IEEE Transactions on Geoscience and Remote Sensing(2020)
摘要
Denoising is a common preprocessing step prior to the analysis and interpretation of hyperspectral images (HSIs). However, the vast majority of methods typically adopted for HSI denoising exploit architectures originally developed for grayscale or RGB images, exhibiting limitations when processing high-dimensional HSI data cubes. In particular, traditional methods do not take into account the high spectral correlation between adjacent bands in HSIs, which leads to unsatisfactory denoising performance as the rich spectral information present in HSIs is not fully exploited. To overcome this limitation, this article considers deep learning models—such as convolutional neural networks (CNNs)—to perform spectral–spatial HSI denoising. The proposed model, called HSI single denoising CNN (HSI-SDeCNN), efficiently takes into consideration both the spatial and spectral information contained in HSIs. Experimental results on both synthetic and real data demonstrate that the proposed HSI-SDeCNN outperforms other state-of-the-art HSI denoising methods. Source code:
https://github.com/mhaut/HSI-SDeCNN
更多查看译文
关键词
Noise reduction,Data models,Hyperspectral imaging,Correlation,Task analysis,Gray-scale
AI 理解论文
溯源树
样例
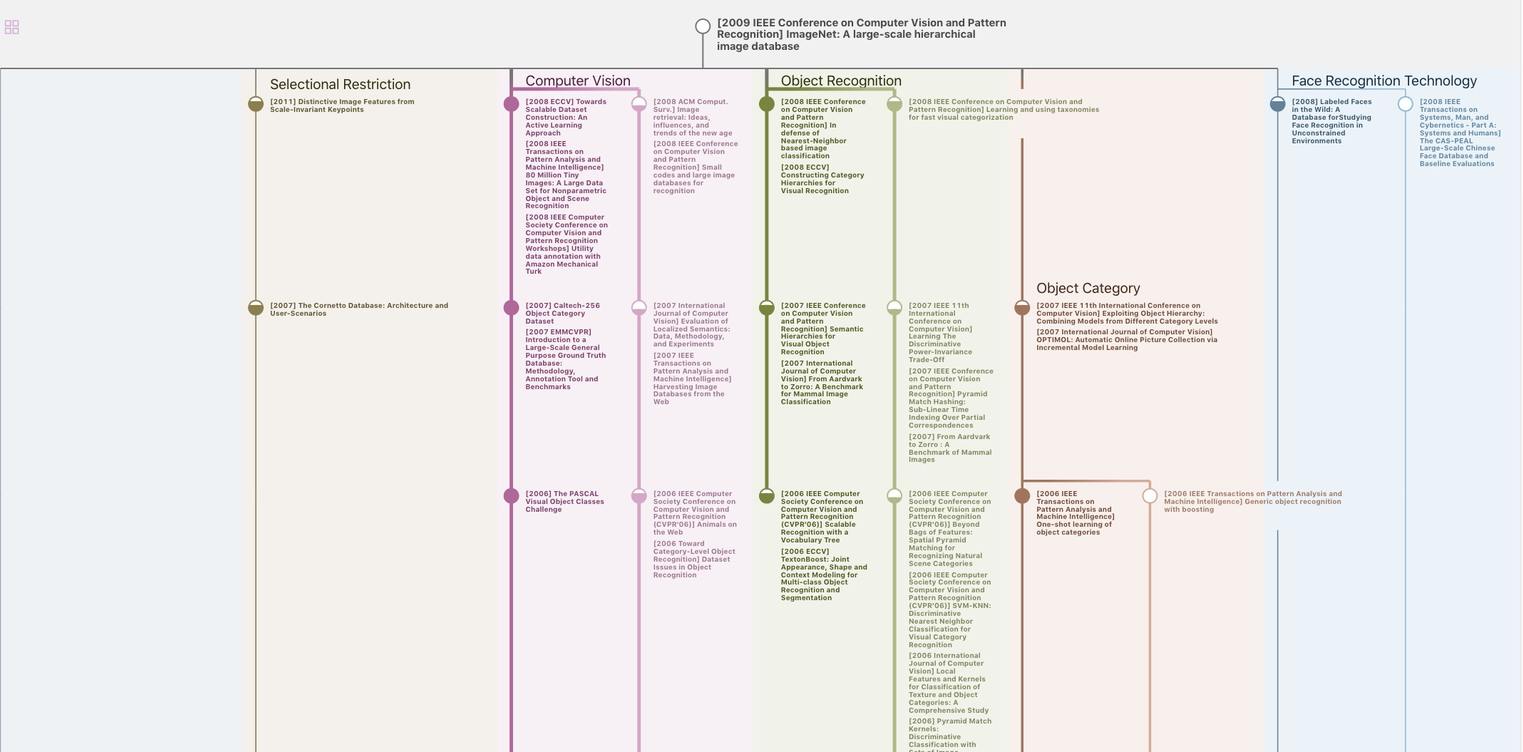
生成溯源树,研究论文发展脉络
Chat Paper
正在生成论文摘要