An Efficient Quality Enhancement Solution For Stereo Images
IMAGE AND GRAPHICS, ICIG 2019, PT III(2019)
摘要
Recently, with additional information in the disparity variant, quality enhancement for stereo images has become an active research field. Current methods generally adopt cost volumes for stereo matching methods to learn correspondence between stereo image pairs. However, with the large disparity in the different viewpoints of stereo images, how to learn the accurate corresponding information remains a challenge. In addition, as the network deepens, traditional convolutional neural networks (CNNs) adopt cascading methods, which results in the high computational cost and memory consumption. In this paper, we propose an end-to-end effective CNN model. Channel-wise attention-based information distillation and long short-term memory (LSTM) are the basic components, which contribute to reconstruct high quality image (DCL network). Within a stereo image pair, we use high quality (HQ) image to guide the image reconstruction of low quality (LQ). To incorporate the stereo correspondence, information fusion-based LSTM module can be used to learn the disparity variant in stereo images. Specially, in order to distill and enhance effective features map, we introduce channel-wise attention-based a long distillation information module with the consideration of interdependencies among feature channels. Experimental results demonstrate that the proposed network achieves the best performance with comparatively less parameters.
更多查看译文
关键词
Quality enhancement, Stereo image, Information distillation, Channel attention, LSTM
AI 理解论文
溯源树
样例
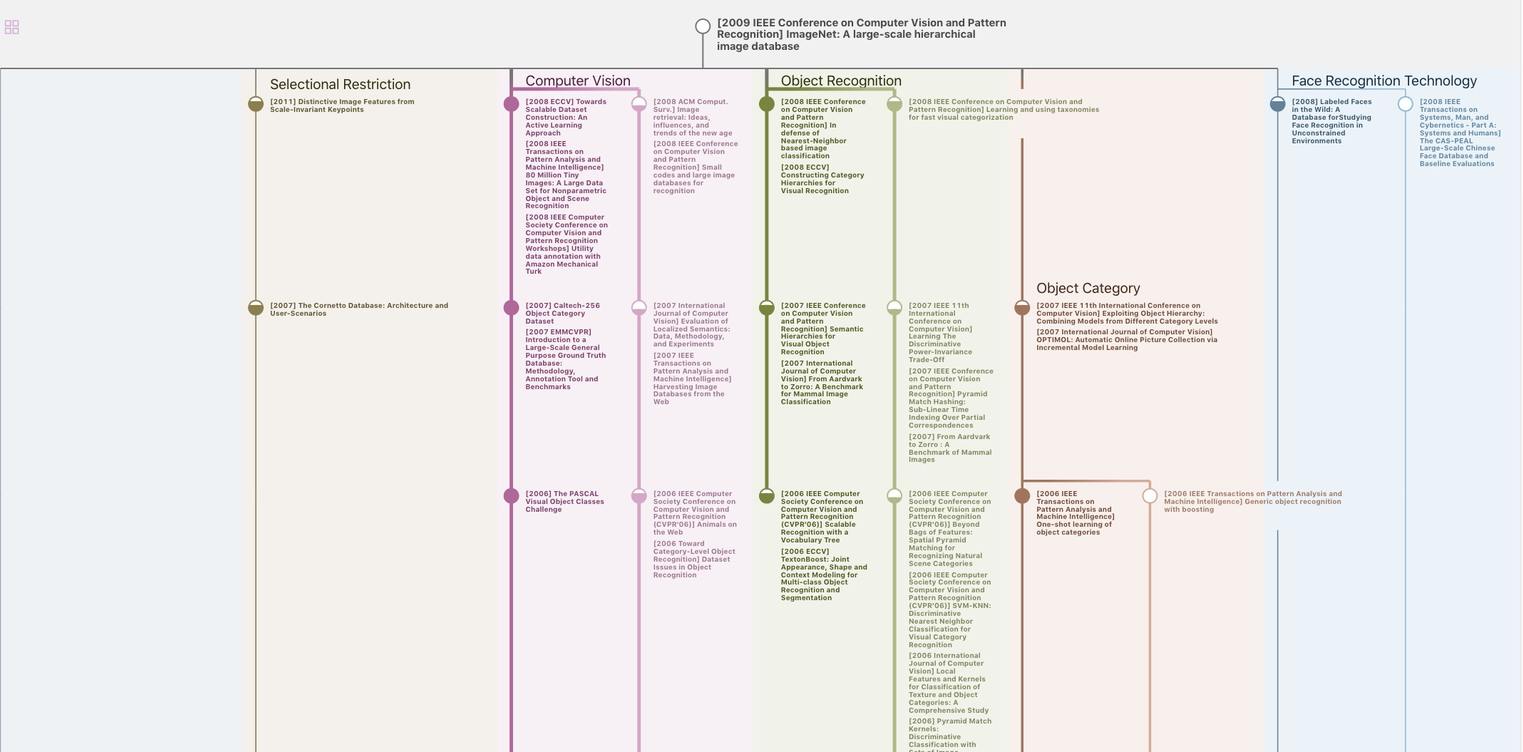
生成溯源树,研究论文发展脉络
Chat Paper
正在生成论文摘要