Robust elastic net estimators for variable selection and identification of proteomic biomarkers
ANNALS OF APPLIED STATISTICS(2019)
摘要
In large-scale quantitative proteomic studies, scientists measure the abundance of thousands of proteins from the human proteome in search of novel biomarkers for a given disease. Penalized regression estimators can be used to identify potential biomarkers among a large set of molecular features measured. Yet, the performance and statistical properties of these estimators depend on the loss and penalty functions used to define them. Motivated by a real plasma proteomic biomarkers study, we propose a new class of penalized robust estimators based on the elastic net penalty, which can be tuned to keep groups of correlated variables together in the selected model and maintain robustness against possible outliers. We also propose an efficient algorithm to compute our robust penalized estimators and derive a data-driven method to select the penalty term. Our robust penalized estimators have very good robustness properties and are also consistent under certain regularity conditions. Numerical results show that our robust estimators compare favorably to other robust penalized estimators. Using our proposed methodology for the analysis of the proteomics data, we identify new potentially relevant biomarkers of cardiac allograft vasculopathy that are not found with nonrobust alternatives. The selected model is validated in a new set of 52 test samples and achieves an area under the receiver operating characteristic (AUC) of 0.85.
更多查看译文
关键词
Robust estimation,regularized estimation,penalized estimation,elastic net penalty,proteomics biomarkers
AI 理解论文
溯源树
样例
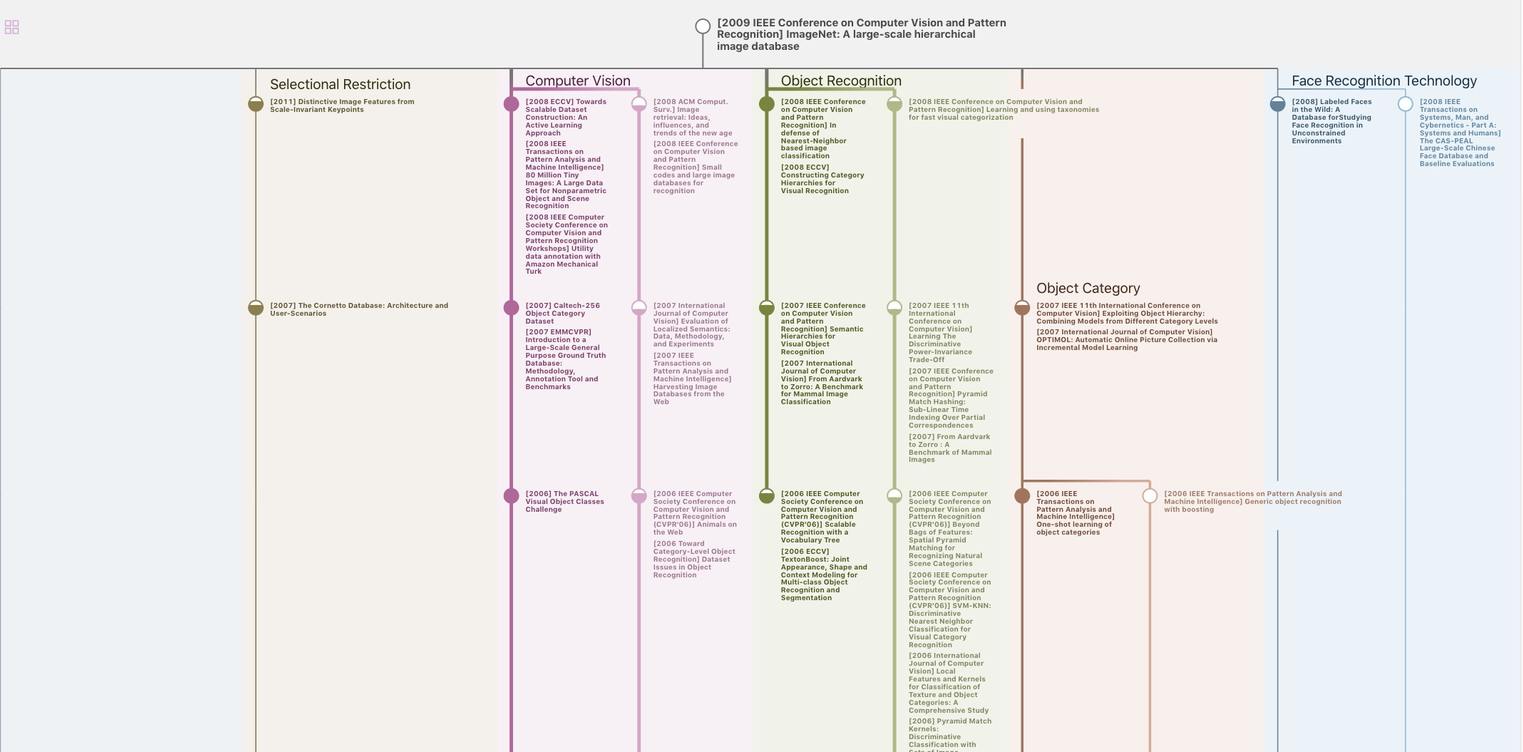
生成溯源树,研究论文发展脉络
Chat Paper
正在生成论文摘要