Learning Low-Level Behaviors And High-Level Strategies In Humanoid Soccer
FOURTH IBERIAN ROBOTICS CONFERENCE: ADVANCES IN ROBOTICS, ROBOT 2019, VOL 2(2020)
摘要
This paper investigates the learning of both low-level behaviors for humanoid robot controllers and of high-level coordination strategies for teams of robots engaged in simulated soccer. Regarding controllers, current approaches typically hand-tune behaviors or optimize them without realistic constraints, for example allowing parts of the robot to intersect with others. This level of optimization often leads to low-performance behaviors. Regarding strategies, most are hand-tuned with arbitrary parameters (like agents moving to pre-defined positions on the field such that eventually they can score a goal) and the thorough analysis of learned strategies is often disregarded. This paper demonstrates how it is possible to use a distributed framework to learn both low-level behaviors, like sprinting and getting up, and high-level strategies, like a kick-off scenario, outperforming previous approaches in the FCPortugal3D Simulated Soccer team.
更多查看译文
关键词
Multi-agent systems, Machine learning, Evolution strategies
AI 理解论文
溯源树
样例
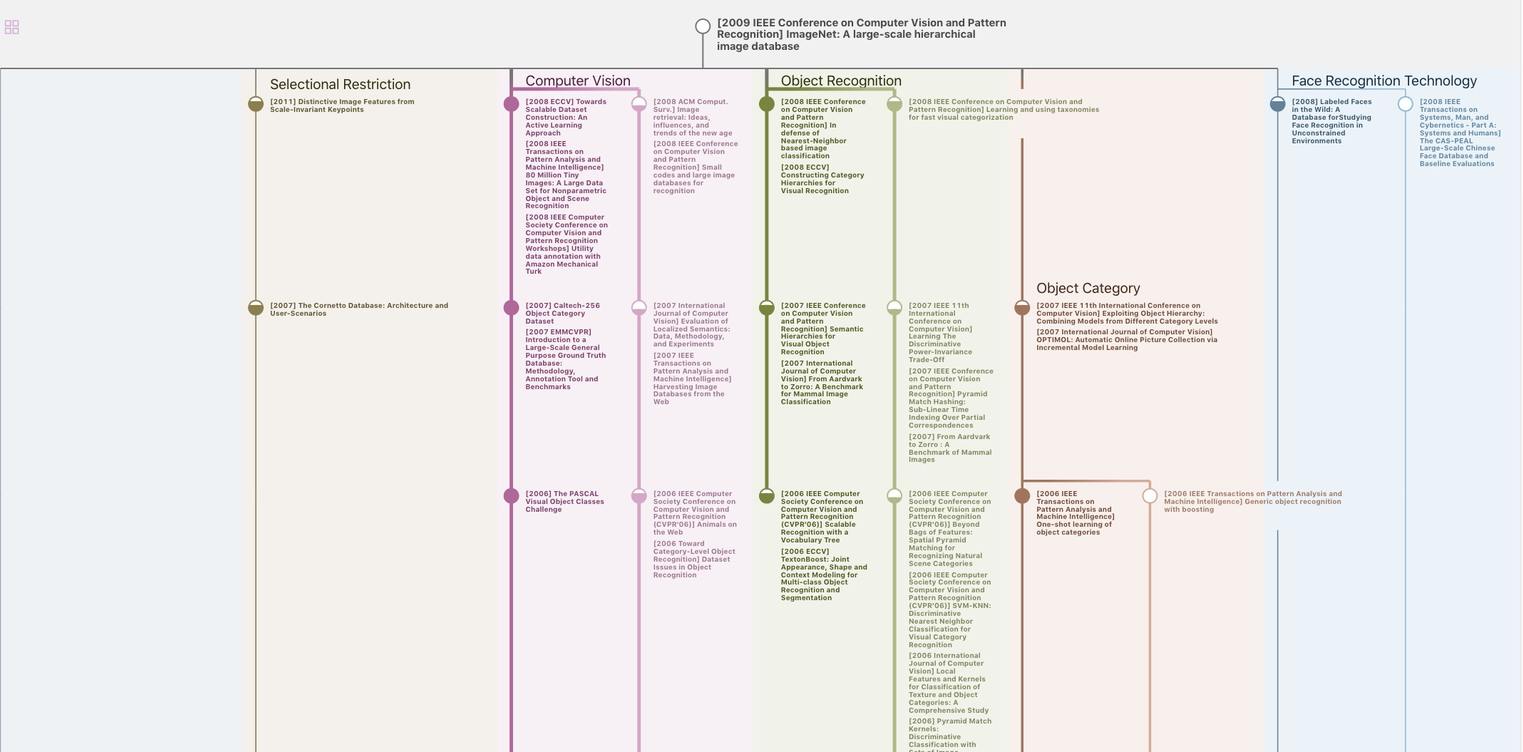
生成溯源树,研究论文发展脉络
Chat Paper
正在生成论文摘要