SME-Net: Sparse Motion Estimation for Parametric Video Prediction through Reinforcement Learning
2019 IEEE/CVF International Conference on Computer Vision (ICCV)(2019)
摘要
This paper leverages a classic prediction technique, known as parametric overlapped block motion compensation (POBMC), in a reinforcement learning framework for video prediction. Learning-based prediction methods with explicit motion models often suffer from having to estimate large numbers of motion parameters with artificial regularization. Inspired by the success of sparse motion-based prediction for video compression, we propose a parametric video prediction on a sparse motion field composed of few critical pixels and their motion vectors. The prediction is achieved by gradually refining the estimate of a future frame in iterative, discrete steps. Along the way, the identification of critical pixels and their motion estimation are addressed by two neural networks trained under a reinforcement learning setting. Our model achieves the state-of-the-art performance on CaltchPed, UCF101 and CIF datasets in one-step and multi-step prediction tests. It shows good generalization results and is able to learn well on small training data.
更多查看译文
关键词
video compression,parametric video prediction,motion vectors,sparse motion estimation,parametric overlapped block motion compensation,reinforcement learning framework,learning-based prediction methods,motion parameters,sparse motion-based prediction,neural networks
AI 理解论文
溯源树
样例
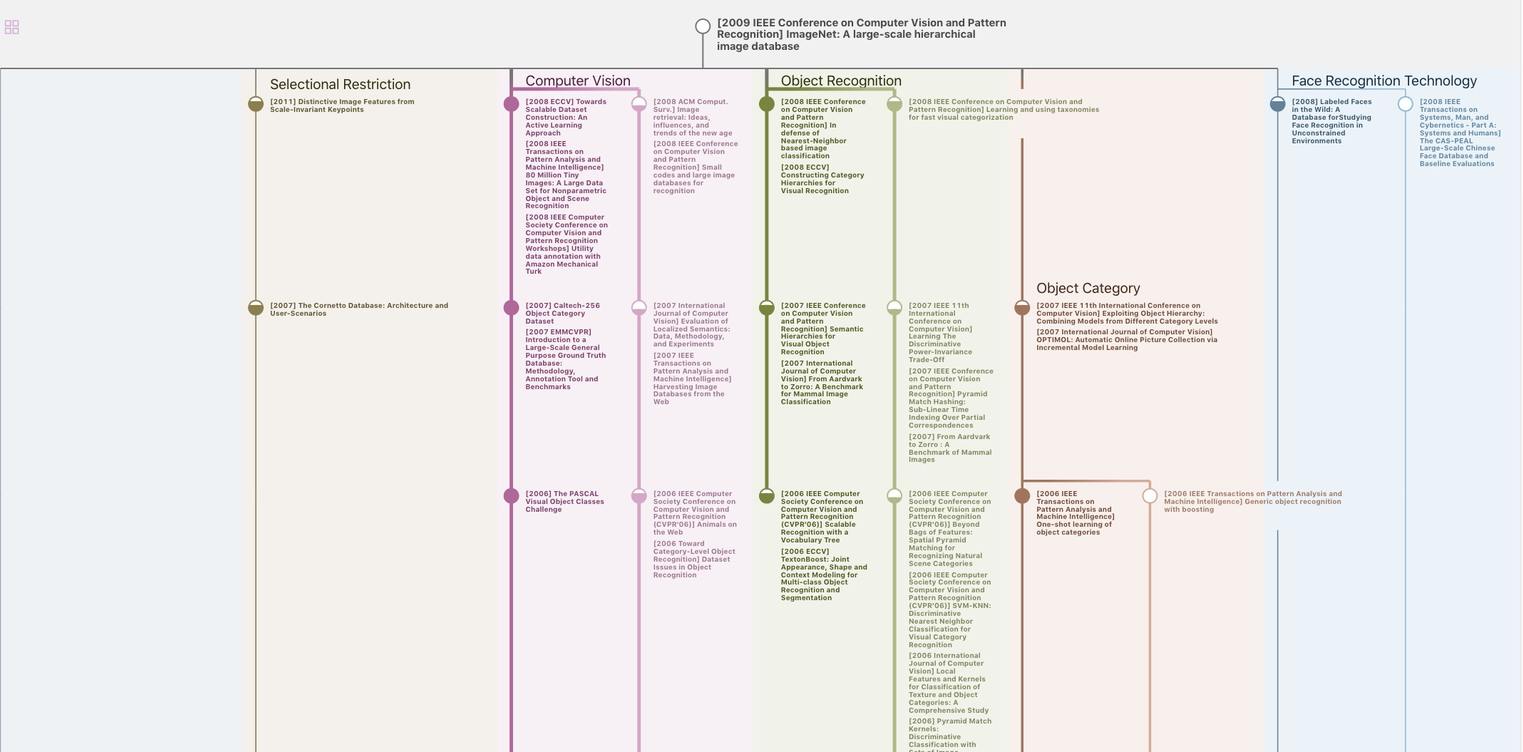
生成溯源树,研究论文发展脉络
Chat Paper
正在生成论文摘要