Common Subspace Based Low-Rank And Joint Sparse Representation For Multi-View Face Recognition
IMAGE AND GRAPHICS, ICIG 2019, PT III(2019)
摘要
Multi-view face data are very common in real-world application, since different viewpoints and various types of sensors attempt to better represent face data. However, these data have large pose variation, which dramatically degrades the performance of multi-view face recognition. To address this, we propose a common subspace based low-rank and joint sparse representation (CSLRJSR) method, which provides a framework encompassing divergence mitigation and feature fusion. In CSLRJSR method, common subspace is learnt to bridge the view, then low-rank and joint sparse representation are exploited to learn and then fuse the discriminative features. Experiments on multi-view face dataset demonstrate that CSLRJSR outperforms the state-of-the-art methods both in two-view and multi-view situations.
更多查看译文
关键词
Multi-view face recognition, Common subspace, Low-rank representation, Joint sparse
AI 理解论文
溯源树
样例
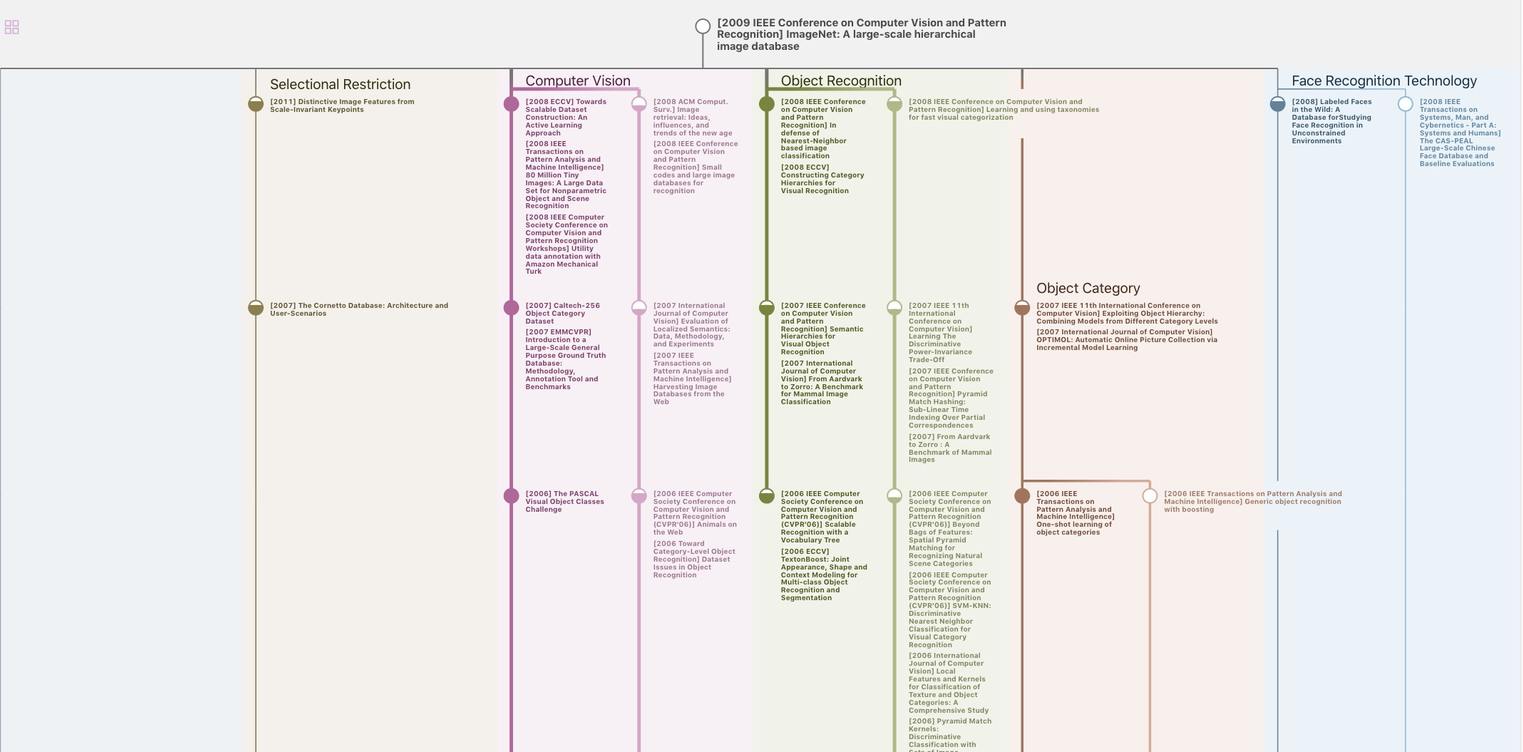
生成溯源树,研究论文发展脉络
Chat Paper
正在生成论文摘要