HIT4Mal: Hybrid image transformation for malware classification
Periodicals(2020)
摘要
AbstractAbstractModern malware evolves various detection avoidance techniques to bypass the state‐of‐the‐art detection methods. An emerging trend to deal with this issue is the combination of image transformation and machine learning models to classify and detect malware. However, existing works in this field only perform simple image transformation methods. These simple transformations have not considered color encoding and pixel rendering techniques on the performance of machine learning classifiers. In this article, we propose a novel approach to encoding and arranging bytes from binary files into images. These developed images contain statistical (eg, entropy) and syntactic artifacts (eg, strings), and their pixels are filled up using space‐filling curves. Thanks to these features, our encoding method surpasses existing methods demonstrated by extensive experiments. In particular, our proposed method achieved 93.01% accuracy using the combination of the entropy encoding and character class scheme on the Hilbert curve. View Figure In this article, we propose a novel approach to encoding and arranging bytes from binary files into images. These developed images contain statistical (eg, entropy) and syntactic artifacts (eg, strings), and their pixels are filled up using space‐filling curves. Thanks to these features, our encoding method surpasses existing methods demonstrated by extensive experiments. In particular, our proposed method achieved 93.01% accuracy using the combination of the entropy encoding and character class scheme on the Hilbert curve.
更多查看译文
AI 理解论文
溯源树
样例
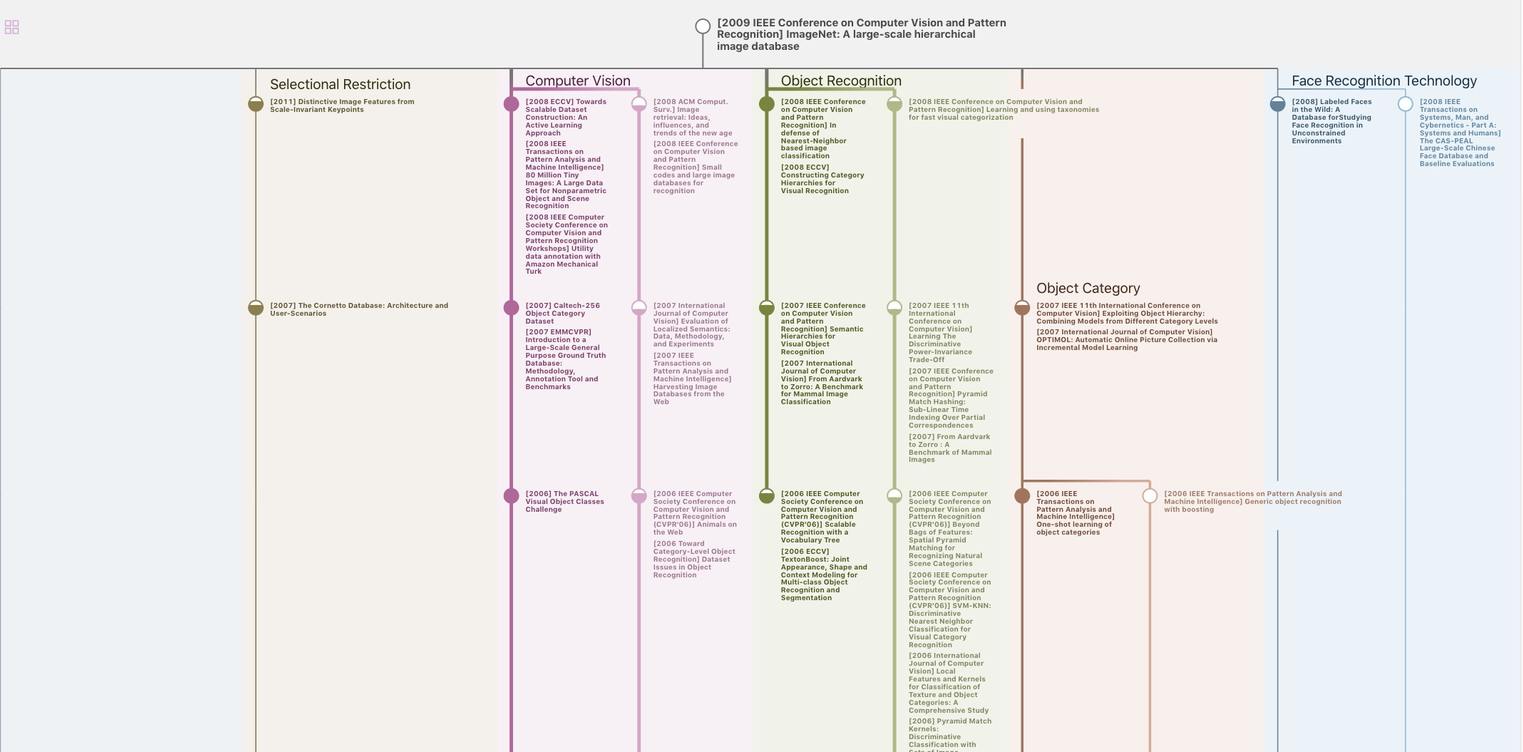
生成溯源树,研究论文发展脉络
Chat Paper
正在生成论文摘要